Location
DATAWorks will be held at IDA's Potomac Yard Location.

IDA (Potomac Yard) is located at 730 East Glebe Road, Alexandria, VA, 22305.
- Potomac Yard Metro Station is now open and within a 5 min walking distance from the IDA conference center. Public transportation is strongly encouraged.
- Paid street and garage parking are available on Dogue Street at the American Physical Therapy Association (see map). IDA does not validate external parking.
- Do not park in the Potomac Yard Center (Target) lot or empty pavement directly across from the IDA building on Seaton Ave; towing is enforced.
- Please note, there will be no IDA shuttle provided this year.
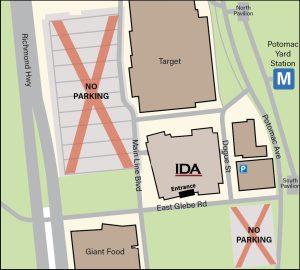
Lodging
*Please note, there will be no IDA shuttle provided this year.
Hyatt Centric Old Town Alexandria
1625 King Street
Alexandria, VA 22314
Telephone: (703) 548-1050
Group Name: DATAWorks
Dates: April 15-19, 2024
Rate: $224
Book your group rate for DATAWorks Room Block
Last Day to Book: Monday, March 25, 2024
Residence Inn Alexandria Old Town/Duke Street
1456 Duke Street
Alexandria, VA 22314
Telephone: (703) 548-5474
Group Name: DATAWorks Room Block
Dates: April 15-19, 2024
Rate: Prevailing Government Per Diem Rate
Book your group rate for DATAWorks Room Block
Last Day to Book: Monday, March 18, 2024
Renaissance Arlington Capital View Hotel
2800 South Potomac Ave
Arlington, VA 22202
Telephone: (703) 413-1300
Group Name: DATAWorks Room Block
Dates: April 15-19, 2024
Rate: Prevailing Government Per Diem Rate
Book your group rate for DATAWorks Room Block
Last Day to Book: Monday, March 18, 2024
The Westin Crystal City
1800 Richmond Hwy
Arlington, VA 22202
Telephone: (703) 486-1111
Group Name: DATAWorks Room Block
Dates: April 15-19, 2024
Rate: Prevailing Government Per Diem Rate
Book your group rate for DATAWorks Room Block
Last Day to Book: Tuesday, March 5, 2024