DATAWorks Speakers and Abstracts

Aayushi Verma
Data Science Fellow II, IDA
From Text to Metadata: Automated Product Tagging with Python and NLP
Day 2, Room: B 3:30 PM-5:00 PM
Aayushi Verma is a Data Science Fellow at the Institute for Defense Analyses (IDA), where she collaborates with the Chief Data Officer to drive IDA's Data Strategy. She has developed numerous data pipelines and visualization dashboards to bring data-driven insights to staff. Her data science interests include machine learning/deep learning, image processing, and extracting stories from data. Aayushi holds an M.S. in Data Science from Pace University, and a B.Sc. (Hons.) in Astrophysics from the University of Canterbury.
Abstract: From Text to Metadata: Automated Product Tagging with Python and NLP
As a research organization, the Institute for Defense Analyses (IDA) produces a variety of deliverables like reports, memoranda, slides, and other formats for our sponsors. Due to their length and volume, summarizing these products quickly for efficient retrieval of information on specific research topics poses a challenge. IDA has led numerous initiatives for historical tagging of documents, but this is a manual and time-consuming process, and must be led periodically to tag newer products. To address this challenge, we have developed a Python-based automated product tagging pipeline using natural language processing (NLP) techniques.
This pipeline utilizes NLP keyword extraction techniques to identify descriptive keywords within the content. Filtering these keywords with IDA's research taxonomy terms produces a set of product tags, serving as metadata. This process also enables standardized tagging of products, compared to the manual tagging process, which introduces variability in tagging quality across project leaders, authors, and divisions. Instead, the tags produced through this pipeline are consistent and descriptive of the contents. This product-tagging pipeline facilitates an automated and standardized process for streamlined topic summarization of IDA's research products, and has many applications for quantifying and analyzing IDA's research in terms of these product tags.
Session Materials: https://dataworks.testscience.org/wp-content/uploads/formidable/23/Wed_SS_Verma.pdf
Adam Miller
Research Staff Member, IDA
Operational T&E of AI-supported Data Integration, Fusion, and Analysis Systems
Day 2, Room: A 10:30 AM-12:30 PM
Adam Miller has been a Research Staff Member at IDA since 2022. He is a member of the IDA Test Science team supporting HSI evaluations of Land and Expeditionary Warfare systems. Previously, Adam worked as a behavioral neuroscientist at The Hospital for Sick Children in Toronto, ON, where he studied how memories are encoded in the brain. He has a PhD in Psychology from Cornell University, and a B.A. in Psychology from Providence College.
Abstract: Operational T&E of AI-supported Data Integration, Fusion, and Analysis Systems
Advancing Test & Evaluation of Emerging and Prevalent Technologies
AI will play an important role in future military systems. However, large questions remain about how to test AI systems, especially in operational settings. Here, we discuss an approach for the operational test and evaluation (OT&E) of AI-supported data integration, fusion, and analysis systems. We highlight new challenges posed by AI-supported systems and we discuss new and existing OT&E methods for overcoming them. We demonstrate how to apply these OT&E methods via a notional test concept that focuses on evaluating an AI-supported data integration system in terms of its technical performance (how accurate is the AI output?) and human systems interaction (how does the AI affect users?).
Session Materials: https://dataworks.testscience.org/wp-content/uploads/formidable/23/Wed_1A_Miller.pptx
Adam Miller
Research Staff Member, IDA
Statistical Advantages of Validated Surveys over Custom Surveys
Day 2, Room: Cafe 5:00 PM-7:00 PM
Adam Miller has been a Research Staff Member at IDA since 2022. He is a member of the IDA Test Science team supporting HSI evaluations of Land and Expeditionary Warfare systems. Previously, Adam worked as a behavioral neuroscientist at The Hospital for Sick Children in Toronto, ON, where he studied how the brain stores memories. He has a PhD in Psychology from Cornell University, and a B.A. in Psychology from Providence College.
Abstract: Statistical Advantages of Validated Surveys over Custom Surveys
Improving the Quality of Test & Evaluation
Surveys play an important role in quantifying user opinion during test and evaluation (T&E). Current best practice is to use surveys that have been tested, or “validated,” to ensure that they produce reliable and accurate results. However, unvalidated (“custom”) surveys are still widely used in T&E, raising questions about how to determine sample sizes for—and interpret data from— T&E events that rely on custom surveys. In this presentation, I characterize the statistical properties of validated and custom survey responses using data from recent T&E events, and then I demonstrate how these properties affect test design, analysis, and interpretation. I show that validated surveys reduce the number of subjects required to estimate statistical parameters or to detect a mean difference between two populations. Additionally, I simulate the survey process to demonstrate how poorly designed custom surveys introduce unintended changes to the data, increasing the risk of drawing false conclusions.

Alex Margolis
Subject Matter Expert, Edaptive Computing, Inc
Bringing No-Code Machine Learning to the average user
Day 3, Room: A 9:50 AM-11:50 AM
Alex Margolis is a Subject Matter Expert at Edaptive Computing, Inc. in Dayton, OH. He has 11 years experience in software development with a focus on Machine Learning and AI. He lead development of ECI AWESOME, a tool designed to bring machine learning into the hands of non-analysts, and ECI SEAMLESS, a tool designed to scan ML/AI algorithms for potential vulnerabilities and limitations.
Abstract: Bringing No-Code Machine Learning to the average user
Sharing Analysis Tools, Methods, and Collaboration Strategies
In the rapidly evolving landscape of technology, Artificial Intelligence (AI) and Machine Learning (ML) have emerged as powerful tools with transformative potential. However, the adoption of these advanced technologies has often been limited to individuals with coding expertise, leaving a significant portion of the population, particularly those without programming skills, on the sidelines. This shift towards user-friendly AI/ML interfaces not only enhances inclusivity but also opens new avenues for innovation. A broader spectrum of individuals can combine the benefits of these cutting-edge technologies with their own domain knowledge to solve complex problems rapidly and effectively. Bringing no-code AI/ML to subject matter experts is necessary to ensure that the massive amount of data being produced by the DoD is properly analyzed and valuable insights are captured. This presentation delves into the importance of making AI and ML accessible to individuals with no coding experience. By doing so, it opens a world of possibilities for diverse participants to engage with and reap the benefits of the AI revolution.
While the prospect of making AI and ML accessible to individuals without coding experience is promising, it comes with its own set of challenges, particularly in addressing the barriers for individuals lacking a background in data analysis. One significant hurdle lies in the complexity of AI and ML algorithms, which often require a nuanced understanding of statistical concepts, data preprocessing, and model evaluation. Individuals without a foundation in analysis may find it challenging to interpret results accurately, hindering their ability to derive meaningful insights from AI-driven applications.
Another challenge is the availability of data, especially in the defense domain. Many models require large amounts of data to be effective. Ensuring the quality and consistency of the chosen dataset is a challenge, as individuals may encounter missing values, outliers, or inaccuracies that can adversely impact the performance of their ML models. Data preprocessing steps such as categorical variable encoding, interpolation, and normalization can be performed automatically, but it is important to understand when to use these techniques and why. Applying transformations such as logarithmic or polynomial transformations can enhance model performance. However, individuals with limited experience may struggle to determine when and how to apply these techniques effectively.
The lack of familiarity with key concepts such as feature engineering, model selection, and hyperparameter tuning can impede users from effectively utilizing AI tools. The black-box nature of some advanced models further complicates matters, as users may struggle to comprehend the inner workings of these algorithms, raising concerns about transparency and trust in AI-generated outcomes. Ethical considerations and biases inherent in AI models also pose substantial challenges. Users without an analysis background may inadvertently perpetuate biases or misinterpret results, underscoring the need for education and awareness to navigate these ethical complexities.
In this talk, we delve into the multifaceted challenges of bringing AI and ML to individuals without a background in analysis, emphasizing the importance of developing solutions that empower individuals to harness the potential of these technologies while mitigating potential pitfalls.

Allison Holston
Mathematical Statistician, Army Evaluation Center
Improving Data Visualizations Through R Shiny
Day 2, Room: Cafe 5:00 PM-7:00 PM
Allison Holston is the Lead Statistician for the Army Evaluation Center in Aberdeen Proving Ground, Maryland. She has an M.S. in Statistics from the University of Georgia and a B.S. in Mathematics & Statistics from Virginia Tech.
Abstract: Improving Data Visualizations Through R Shiny
Sharing Analysis Tools, Methods, and Collaboration Strategies
Poster Abstract
Shiny is an R package and framework used to build applications in a web browser without needing any programming experience. This capability allows the power and functionality of R to be accessible to audiences that would typically not utilize R because of the roadblocks with learning a programming language.
Army Evaluation Center (AEC) has rapidly increased their utilization of R shiny to develop apps for use in Test and Evaluation. These apps have allowed evaluators to upload data, perform calculations, and then create data visualizations which can be customized for their reporting needs. The apps have streamlined and standardized the analysis process.
The poster outlines the before and after of three data visualizations utilizing the shiny apps. The first displays survey data using the likert package. The second graphs a cyberattack cycle timeline. And the third displays individual qualification course results and calculates qualification scores. The apps are hosted in a cloud environment and their usage is tracked with an additional shiny app.

Andrei Gribok, Mike DiNicola
Regularization Approach to Learning Bioburden Density for Planetary Protection
Day 2, Room: C 3:30 PM-5:00 PM
Andrei Gribok is a Distinguished Research Scientist in Instrumentation, Controls, and Data Science Department. He received his Ph.D. in Mathematical Physics from Moscow Institute of Biological Physics in 1996 and his B.S. and M.S. degrees in systems science/nuclear engineering from Moscow Institute of Physics and Engineering in 1987. Dr. Gribok worked as an instrumentation and control researcher at the Institute of Physics and Power Engineering, Russia where he conducted research on advanced data driven algorithms for fault detection and prognostics for fast breeder reactors. He also worked as an invited research scientist at Cadarache Nuclear Research Center, France, where his research focus was on ultrasonic visualization systems for liquid metal reactors. Dr. Gribok holds position of Research Associate Professor with Department of Nuclear Engineering, University of Tennessee, Knoxville. From 2005 until 2015 Dr. Gribok was employed as a Research Scientist with Telemedicine and Advanced Technology Research Center of the U.S. Army Medical Research and Materiel Command, USDA, and USARIEM. His research interests included military operational medicine and telemedicine for combat casualty care missions. Dr. Gribok was a member of a number of international programs including IAEA coordinated research program on acoustical signal processing for the detection of sodium boiling or sodium-water reaction in LMFRs and large-scale experiments on acoustical water-in-sodium leak detection in LMFBR. Dr. Gribok is an author and co-author of three book chapters, over 40 journal peer-reviewed papers and numerous peer-reviewed conference papers. He is also a co-author of the book "Optimization Techniques in Computer Vision: Ill-Posed Problems and Regularization " Springer, 2016
Michael DiNicola is a senior systems engineer in the Systems Modeling, Analysis & Architectures Group at the Jet Propulsion Laboratory (JPL). At JPL, Michael has worked on several mission concept developments and flight projects, including Europa Clipper, Europa Lander and Mars Sample Return, developing probabilistic models to evaluate key mission requirements, including those related to planetary protection. He works closely with microbiologists in the Planetary Protection group to model assay and sterilization methods, and applies mathematical and statistical methods to improve Planetary Protection engineering practices at JPL and across NASA. At the same time, he also works with planetary scientists to characterize the plumes of Enceladus in support of future mission concepts. Michael earned his B.S. in Mathematics from the University of California, Los Angeles and M.A. in Mathematics from the University of California, San Diego.
Abstract: Regularization Approach to Learning Bioburden Density for Planetary Protection
Over the last 2 years, the scientific community and the general public both saw a surge of practical application of artificial intelligence (AI) and machine learning (ML) to numerous technological and everyday problems. The emergence of AI/ML data-driven tools was enabled by decades of research in statistics, neurobiology, optimization, neural networks, statistical learning theory, and other fields—research that synergized into an overarching discipline of learning from data.
Learning from data is one of the most fundamental problems facing empirical science. In the most general setting, it may be formulated as finding the true data-generating function or dependency given a set of noisy empirical observations. In statistics, the most prominent example is estimation of the cumulative distribution function or probability density function from a limited number of observations. The principal difficulty in learning functional dependencies from a limited set of noisy data is the ill-posed nature of this problem. Here, “ill-posed” is used in the sense suggested by Hadamard—namely, that the problem’s solution lacks existence, uniqueness, or stability with respect to minor variations in the data. In other words, ill-posed problems are underdetermined as the data do not contain all the information necessary to arrive at a unique, stable solution.
Finding functional dependencies from noisy data may in fact be hindered by all three conditions of ill-posedness: the data may not contain information about the solution, numerous solutions can be found to fit the data, and the solution may be unstable with respect to minor variations in the data. To deal with ill-posed problems, a regularization method was proposed for augmenting the information contained in the data with some additional information about the solution (e.g., its smoothness). In this presentation, we demonstrate how the regularization techniques, as applied to learning function dependencies with neural networks, can be successfully applied to the planetary protection problem of estimating microbial bioburden density (i.e., spores per square meter) on spacecraft.
We shall demonstrate that the problem of bioburden density estimation can be formulated as a solution to a least squares problem, and that this problem is indeed ill-posed. This presentation will elucidate the relationship between maximum likelihood estimates and the least squares solution by demonstrating their mathematical equivalence. It will be shown that the maximum likelihood estimation is identical to the differentiation of the cumulative count of colony forming units which can be represented as a least squares problem. Since the problem of differentiation of noisy data is ill-posed the method of regularization will be applied to obtain a stable solution.
It will demonstrate that the problem of bioburden density estimation can be cast as a problem of regularized differentiation of the cumulative count of colony-forming units found on the spacecraft. The regularized differentiation will be shown to be a shrinkage estimator and its performance compared with other shrinkage estimators commonly used in statistics for simultaneously estimating parameters of a set of independent Poisson distributions. The strengths and weaknesses of the regularized differentiation will then be highlighted in comparison to the other shrinkage estimators.
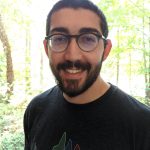
Andrew Cooper
Graduate Student, Virginia Tech Department of Statistics
Data-Driven Robust Design of an Aeroelastic Wing
Day 2, Room: B 3:30 PM-5:00 PM
Andrew Cooper is a 4th-year PhD candidate in Virginia Tech's Department of Statistics. He received his bachelors and masters degrees in Statistical Science from Duke University. His research areas include computer experiments and surrogate modeling, as well as Bayesian methodology.
Abstract: Data-Driven Robust Design of an Aeroelastic Wing
Improving the Quality of Test & Evaluation
This paper applies a Bayesian Optimization approach to the design of a wing subject to stress and aeroelastic constraints. The parameters of these constraints, which correspond to various flight conditions and uncertain parameters, are prescribed by a finite number of scenarios. Chance-constrained optimization is used to seek a wing design that is robust to the parameter variation prescribed by such scenarios. This framework enables computing designs with varying degrees of robustness. For instance, we can deliberately eliminate a given number of scenarios in order to obtain a lighter wing that is more likely to violate a requirement, or might seek a conservative wing design that satisfies the constraints for as many scenarios as possible.
Session Materials: https://dataworks.testscience.org/wp-content/uploads/formidable/23/Wed_SS_Cooper.pdf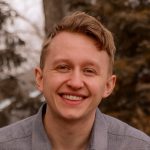
Andrew Simpson
Ph.D. Student, South Dakota State University
Clustering Singular and Non-Singular Covariance Matrices for Classification
Day 2, Room: B 3:30 PM-5:00 PM
Ph.D. Student in the Computational Science and Statistics program at South Dakota State University. Research focuses on novel methods for modeling data generated from a hierarchical sampling process where subpopulation structures exist. The main application of this research is to forensic statistics and source identification.
Abstract: Clustering Singular and Non-Singular Covariance Matrices for Classification
In classification problems when working in high dimensions with a large number of classes and few observations per class, linear discriminant analysis (LDA) requires the strong assumptions of a shared covariance matrix between all classes and quadratic discriminant analysis leads to singular or unstable covariance matrix estimates. Both of these can lead to lower than desired classification performance. We introduce a novel, model-based clustering method which can relax the shared covariance assumptions of LDA by clustering sample covariance matrices, either singular or non-singular. This will lead to covariance matrix estimates which are pooled within each cluster. We show using simulated and real data that our method for classification tends to yield better discrimination compared to other methods.
Session Materials: https://dataworks.testscience.org/wp-content/uploads/formidable/23/Wed_SS_Simpson.pdfAnna Flowers
Graduate Student, Virginia Tech
Statistical Modeling of Machine Learning Operating Envelopes
Day 2, Room: Cafe 5:00 PM-7:00 PM
Anna Flowers is a third-year Ph.D student in the statistics department at Virginia Tech, where she is a recipient of the Jean Gibbons Fellowship. She received a B.S. in Mathematical Statistics from Wake Forest University in 2021 and an M.S. in Statistics from Virginia Tech in 2023. Her research focuses on mixture modeling and large-scale Gaussian Process approximation, particularly as it applies to estimating model performance. She is co-advised by Bobby Gramacy and Chris Franck.
Abstract: Statistical Modeling of Machine Learning Operating Envelopes
Sharing Analysis Tools, Methods, and Collaboration Strategies
Characterizing a model’s operating envelope, or the range of values for which the model performs well, is often of interest to a researcher. Of particular interest is estimating the operating envelope of a model at each phase of a testing process. Bayesian methods have been developed to complete this task for relatively simple models, but at present there is no method for more complicated models, in particular Machine Learning models. Preliminary research has shown that metadata influences model performance, although this work has primarily focused on categorical metadata. We are currently conducting a more rigorous investigation of the effect of metadata on a Machine Learning model’s operating envelope using the MNIST handwritten data set.
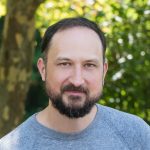
Austin Amaya and Sean Dougherty
The Role of Bayesian Multilevel Models in Performance Measurement and Prediction
Day 3, Room: C 1:00 PM-3:00 PM
Dr. Austin Amaya is the lead for Algorithm Testing and Evaluation at MORSE. He has more than 10 years' of experience developing and testing AI/ML-driven systems within the DoD.
Abstract: The Role of Bayesian Multilevel Models in Performance Measurement and Prediction
Advancing Test & Evaluation of Emerging and Prevalent Technologies
T&E relies on series of observations under varying conditions in order to assess overall performance. Traditional evaluation methods can in fact oversimplify complex structures in the data, where variance within groups of observations made under identical experimental conditions differs significantly from that between such groups, introducing biases and potentially misrepresenting true performance capabilities. To address these challenges, MORSE is implementing Bayesian multilevel models. These models adeptly capture the nuanced group-wise structure inherent in T&E data, simultaneously estimating intragroup and intergroup parameters while efficiently pooling information across different model levels. This methodology is particularly adept at regressing against experimental parameters, a feature that conventional models often overlook. A distinct advantage of employing Bayesian approaches lies in their ability to generate comprehensive uncertainty distributions for all model parameters, providing a more robust and holistic understanding how performance varies. Our application of these Bayesian multilevel models has been instrumental in generating credible intervals for performance metrics for applications with varying levels of risk tolerance. Looking forward, our focus will shift towards advancing T&E past the idea of measuring performance towards the idea of modeling performance.
Session Materials: https://dataworks.testscience.org/wp-content/uploads/formidable/23/Thrs_CS3_Amaya.pdf
Dr. Barney Ricca
University of Colorado, Colorado Springs
R for Reproducible Scientific Analysis
Day 1, Room: C 9:00 AM-4:00 PM
Dr. Bernard (Barney) Ricca is a Research Associate Professor at the Lyda Hill Institute for Human Resilience at the University of Colorado Colorado Springs, and the President of the Society for Chaos Theory, Psychology, and Life Sciences. His research focuses on the development of nonlinear dynamical systems analysis approaches and the applications of dynamical systems to the social and behavioral sciences, particularly to dynamics of trauma survivors. Recent projects include analyses of hurricane and wildfire survivors and a study of small-group dynamics in schools. He is currently the Co-Principal Investigator on an NSF-funded project to investigate the post-trauma dynamics of motor vehicle accident survivors. He received a Ph.D. in Physics from the University of Michigan.
Abstract: R for Reproducible Scientific Analysis
The Carpentries more introductory R lesson. In addition to their standard content, this workshop covers data analysis and visualization in R, focusing on working with tabular data and other core data structures, using conditionals and loops, writing custom functions, and creating publication-quality graphics. As their more introductory R offering, this workshop also introduces learners to RStudio and strategies for getting help. This workshop is appropriate for learners with no previous programming experience.
Session Materials Website: https://barneyricca.github.io/2024-04-16-ida/

Boris Chernis
Research Associate, IDA
Advancing Reproducible Research: Concepts, Compliance, and Practical Applications
Day 2, Room: D 1:40 PM-3:10 PM
I have an MS in Computer Science and have been working at the Institute for Defense Analyses since 2020. I have experience in data analytics, machine learning, cyber security, and miscellaneous software engineering.
Abstract: Advancing Reproducible Research: Concepts, Compliance, and Practical Applications
Advancing Test & Evaluation of Emerging and Prevalent Technologies
Reproducible research principles ensure that analyses can be verified and defended by meeting the criterion that conducting the same analysis on the same data should yield identical results. Not only are reproducible analyses more defensible and less susceptible to errors, but they also enable faster iteration and yield cleaner results. In this seminar, we will delve into how to conceptualize reproducible research and explore how reproducible research practices align with government policies. Additionally, we will provide hands-on examples, using Python and MS Excel, illustrating various approaches for conducting reproducible research.
Session Materials: https://dataworks.testscience.org/wp-content/uploads/formidable/23/Wed_MT2_Chernis.zip
Dr. Bram Lillard
Director, IDA
Day 3, Room: A+B 8:30 AM-8:45 AM
Abstract:

Cameron Liang
Research Staff Member, IDA
Demystifying Deep Learning - Aircraft Identification from Satellite Images
Day 3, Room: A 1:00 PM-3:00 PM
Dr. Cameron Liang is a research staff member. He received his Ph.D in Astronomy & Astrophysics in 2018 from the University of Chicago. Prior to joining IDA, he was a Postdoctoral Researcher at the University of California, Santa Barbara. Dr. Liang worked on theoretical and observational aspects of galaxy formation using magneto-hydrodynamic simulations. At IDA, he works on a variety of space-related topics, such as orbital debris and dynamic space operations.
Abstract: Demystifying Deep Learning - Aircraft Identification from Satellite Images
Sharing Analysis Tools, Methods, and Collaboration Strategies
In the field of Artificial Intelligence and Machine Learning (AI/ML), the literature can be filled with technical language and/or buzzwords, making it challenging for readers to understand the content. It will be a pedagogical talk focusing on demystifying "Artificial Intelligence" by providing a mathematical, but most importantly, an intuitive understanding of how deep learning really works. I will provide some existing tools and practical steps for how one can train their own neutral networks using an example of automatically identifying aircrafts and their attributes (e.g., civil vs. military, engine types, and size) from satellite images. Audience members with some knowledge of linear regression and coding will be armed with an increased understanding, confidence, and practical tools to develop their own AI applications.
Session Materials: https://dataworks.testscience.org/wp-content/uploads/formidable/23/Thrs_CS1_Liang.pptx
Charles Wheaton
Student, United States Military Academy
Evaluating Military Vehicle Object Detectors with Synthetic Data
Day 2, Room: Cafe 5:00 PM-7:00 PM
I am a fourth-year cadet at the United States Military Academy. After my graduation this spring, I will join the US Army Cyber branch as a capabilities developer. I am interested in data science and artificial intelligence, specifically graph neural networks.
Abstract: Evaluating Military Vehicle Object Detectors with Synthetic Data
The prevalence of unmanned aerial systems (UAS) and remote sensing technology on the modern battlefield has laid the foundation for an automated targeting system. However, no computer vision model has been trained to support such a system. Difficulties arise in creating these models due to a lack of available battlefield training data. This work aims to investigate the use of synthetic images generated in Unreal Engine as supplementary training data for a battlefield image classifier. We test state-of-the-art computer vision models to determine their performance on drone images of modern battlefields and the suitability of synthetic images as training data. Our results suggest that synthetic training images can improve the performance of state-of-the-art models in battlefield computer vision tasks.
This is an abstract for a student poster.
Chris Jenkins
R&D S&E, Cybersecurity, Sandia National Labs
Moving Target Defense for Space Systems
Day 2, Room: B 10:30 AM-12:30 PM
Chris is a Principal Cybersecurity Research & Development staff member in the Systems Security Research Department as part of Sandia National Laboratories’ Information Operations Center. Chris supports Sandia’s mission in three key areas: cyber-physical cybersecurity research, space cybersecurity, and cybersecurity expertise outside the lab. Chris regularly publishes in the open literature, is responsible for multiple technical advances, has been granted patents, and actively seeks opportunities to transition technology outside of Sandia.
Chris leads a team researching innovative ways to protect critical infrastructure and other high-consequence operational technology. His work uses a technology called moving target defense (MTD) to protect these systems from adversary attack. He has partnered with Purdue University to determine the strength of the innovative, patent pending MTD algorithm he created. His work has explored integrating MTD into real-time communication systems employed in space systems and other national security relevant communications architectures. His current research represents Sandia’s national commitment to space systems and Sandia’s strategic investment in the Science and Technology Advancing Resilience for Contested Space Mission Campaign.
Abstract: Moving Target Defense for Space Systems
Advancing Test & Evaluation of Emerging and Prevalent Technologies
Space systems provide many critical functions to the military, federal agencies, and infrastructure networks. In particular, MIL-STD-1553 serves as a common command and control network for space systems, nuclear weapons, and DoD weapon systems. Nation-state adversaries have shown the ability to disrupt critical infrastructure through cyber-attacks targeting systems of networked, embedded computers. Moving target defenses (MTDs) have been proposed as a means for defending various networks and systems against potential cyber-attacks. In addition, MTDs could be employed as an ‘operate through’ mitigation for improving cyber resilience.
We devised a MTD algorithm and tested its application to a MIL-STD-1553 network. We demonstrated and analyzed four aspects of the MTD algorithm usage: 1) characterized the performance, unpredictability, and randomness of the core algorithm, 2) demonstrated feasibility by conducting experiments on actual commercial hardware, 3) conducted an exfiltration experiment where the reduction in adversarial knowledge was 97%, and 4) employed the LSTM machine learning model to see if it could defeat the algorithm and glean information about the algorithm’s resistance to machine learning attacks. Given the above analysis, we show that the algorithm has the ability to be used in real-time bus networks as well as other (non-address) applications.
Session Materials: https://dataworks.testscience.org/wp-content/uploads/formidable/23/Wed_1B_Jenkins.pdf
Christian Smart and Murray Cantor
Best of Both Worlds: Combining Parametric Cost Risk Analysis with Earned Value Management
Day 3, Room: C 9:50 AM-11:50 AM
Dr. Christian Smart is a Senior Programmatic Risk Analyst with NASA’s Jet Propulsion Laboratory. He has experience supporting both NASA and the Department of Defense in the theory and application of risk, cost, and schedule analytics for cutting-edge programs, including nuclear propulsion and hypersonic weapon systems. For several years he served as the Cost Director for the Missile Defense Agency. An internationally recognized expert on risk analysis, he is the author of Solving for Project Risk Management: Understanding the Critical Role of Uncertainty in Project Management (McGraw-Hill, 2020).
Dr. Smart received the 2021 Frank Freiman lifetime achievement award from the International Cost Estimating and Analysis Association. In 2010, he received an Exceptional Public Service Medal from NASA for the application of risk analysis. Dr. Smart was the 2009 recipient of the Parametrician of the Year award from the International Society of Parametrics Analysts. Dr. Smart has BS degrees in Mathematics and Economics from Jacksonville State University, an MS in Mathematics from the University of Alabama in Huntsville (UAH), and a PhD in Applied Mathematics from UAH.
Murray Cantor is a retired IBM Distinguished Engineer. With his Ph.D. in mathematics from the University of California at Berkeley and extensive experience in managing complex, innovative projects, he has focused on applying predictive reasoning and causal analysis to the execution and economics of project management.
In addition to many journal articles, Murray is the author of two books: Object-Oriented Project Management with UML and Software Leadership. He is an inventor of 15 IBM patents. After retiring from IBM, he was a founder and lead scientist of Aptage, which developed and delivered tools for learning and tracking the probability of meeting project goals. Aptage was sold to Planview.
Dr. Cantor’s quarter-century career with IBM included two periods:
• An architecture and senior project manager for the Workstation Division and
• An IBM Distinguished Engineer in the Software Group and an IBM Rational CTO team member.
The second IBM stint began with IBM acquiring Rational Software, where Murray was the Lead Engineer for Rational Services. In that role, he consulted on delivering large projects at Boeing, Raytheon, Lockheed, and various intelligence agencies. He was the IBM representative to SysML partners who created the Object Management Group’s System Modeling Language standard. While at Rational, He was the lead author of the Rational Unified Process for System Engineering (RUPSE).
Before joining Rational, he was project lead at the defense and intelligence contractor TASC, delivering systems for Space Command.
Abstract: Best of Both Worlds: Combining Parametric Cost Risk Analysis with Earned Value Management
Solving Program Evaluation Challenges
Murray Cantor, Ph.D., Cantor Consulting
Christian Smart, Ph.D., Jet Propulsion Laboratory, California Institute of Technology
Cost risk analysis and earned value data are typically used separately and independently to estimate Estimates at Completion (EAC). However, there is significant value to combining the two in order to improve the accuracy of EAC forecasting. In this paper, we provide a rigorous method for doing this using Bayesian methods.
In earned value management (EVM), the Estimate at Completion (EAC) is perhaps the critical metric. It is used to forecast the effort’s total work cost as it progresses. In particular, it is used to see if the work is running over or under its planned budget, specified as the budget at completion (BAC).
Separate probability distribution functions (PDF) of the EAC at the onset of the effort and after some activities have been completed show the probability that EAC will fall within the BAC, and, conversely, the probability it won’t. At the onset of an effort, the budget is fixed, and the EAC is uncertain. As the work progresses, some of the actual costs (AC) are reported. The EAC uncertainty should then decrease and the likelihood of meeting the budget should increase. If the area under the curve to the left of the BAC decreases as the work progresses, the budget is in jeopardy, and some management action is warranted.
This paper will explain how to specify the initial PDF and learn the later PDFs from the data tracked in EVM. We describe the technique called Bayesian parameter learning (BPL). We chose this technique because it is the most robust for exploiting small sets of progress data and is most easily used by practitioners. This point will be elaborated further in the paper.

Christian Frederiksen
PhD Student, Tulane University
Bayesian Design of Experiments and Parameter Recovery
Day 2, Room: B 3:30 PM-5:00 PM
Christian is a 5th year mathematics PhD student at Tulane University expecting to graduate in 2024. His research involves a combination of Bayesian statistics, partial differential equations, and Markov Chain Monte Carlo and includes both abstract and applied components. After working at the Virginia Tech National Security Institute during the summer of 2023 where his work largely focused on Bayesian design of experiments he is interested in pursuing a career in testing and evaluation.
Abstract: Bayesian Design of Experiments and Parameter Recovery
Improving the Quality of Test & Evaluation
With recent advances in computing power, many Bayesian methods that were once impracticably expensive are becoming increasingly viable. Parameter recovery problems present an exciting opportunity to explore some of these Bayesian techniques. In this talk we briefly introduce Bayesian design of experiments and look at a simple case study comparing its performance to classical approaches. We then discuss a PDE inverse problem and present ongoing efforts to optimize parameter recovery in this more complicated setting. This is joint work with Justin Krometis, Nathan Glatt-Holtz, Victoria Sieck, and Laura Freeman.
Session Materials: https://dataworks.testscience.org/wp-content/uploads/formidable/23/Wed_SS_Frederiksen.pdfChristian Smart
Senior Programmatic Risk Analyst, JPL
Beyond the Matrix: The Quantitative Cost and Schedule Risk Management Imperative
Day 3, Room: C 9:50 AM-11:50 AM
Dr. Christian Smart is a Senior Programmatic Risk Analyst with NASA’s Jet Propulsion Laboratory. He has experience supporting both NASA and the Department of Defense in the theory and application of risk, cost, and schedule analytics for cutting-edge programs, including nuclear propulsion and hypersonic weapon systems. For several years he served as the Cost Director for the Missile Defense Agency. An internationally recognized expert on risk analysis, he is the author of Solving for Project Risk Management: Understanding the Critical Role of Uncertainty in Project Management (McGraw-Hill, 2020).
Dr. Smart received the 2021 Frank Freiman lifetime achievement award from the International Cost Estimating and Analysis Association. In 2010, he received an Exceptional Public Service Medal from NASA for the application of risk analysis. Dr. Smart was the 2009 recipient of the Parametrician of the Year award from the International Society of Parametrics Analysts. Dr. Smart has BS degrees in Mathematics and Economics from Jacksonville State University, an MS in Mathematics from the University of Alabama in Huntsville (UAH), and a PhD in Applied Mathematics from UAH.
Abstract: Beyond the Matrix: The Quantitative Cost and Schedule Risk Management Imperative
Solving Program Evaluation Challenges
In the modern world, we underappreciate the role of uncertainty and tend to be blind to risk. As the Nobel Prize–winning economist Kenneth Arrow once wrote, “Most individuals underestimate the uncertainty of the world . . . our knowledge of the way things work, in society or in nature, comes trailing clouds of vagueness.” The resistance to recognition of risk and uncertainty includes project management. As a colleague of mine aptly put it, “Project management types, especially, have a tendency to treat plans as reality.” However, projects of all types – weapon systems, robotic and human space efforts, dams, tunnels, bridges, the Olympics, etc. – experience regular and often extreme cost growth and schedule delays. Cost overruns occur in 80% or more of project development efforts and schedule delays happen in 70% or more. For many types of projects, average cost growth is 50% or more and these costs double in more than one in every six. These widespread and enduring increases reflect the high degree of risk inherent in projects. If there were no recurring history of cost and schedule growth, there would be no need for resource risk analysis and management. The planning of such projects would be as easy as planning a trip to a local dry cleaner. Instead, the tremendous risk inherent in such projects necessitates the consideration of risk and uncertainty throughout a program’s life cycle.
In practice the underestimation of project risk manifests itself in one of four ways. First, projects often completely ignore variation and rely exclusively on point estimates. As we will demonstrate, overlooking risk in the planning stages guarantees cost growth and schedule delays. Second, even when variation is considered, there is often an exclusive reliance on averages. We will show that there is much more to risk than simple averages. Third, even when the potential consequences are considered, risk matrices are often used in place of a rigorous quantitative analysis overreliance on risk matrices. We will provide proof that qualitative risk matrices underestimate the true degree of uncertainty. Fourth, an often-overlooked weakness in quantitative applications is the human element. There is an innate bias early in a project’s lifecycle to perceive less risk than is present which leads to a significant underestimation in uncertainty until late in a project’s development, at which point many of the risks a project faces have either been avoided, mitigated, or confronted. Even the application of best practices in risk analysis often lead to uncertainty ranges that are significantly tighter than indicated by history. Reasons for this phenomenon are discussed and the calibration of risk analysis outputs to historical cost growth and schedule delays is presented as a remedy.
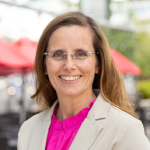
Christina Houfek and Maegan Nix
Lead PjM, VT-ARC
The Joint Test Concept: Reimagining T&E for the Modern Joint Environment
Day 2, Room: A 10:30 AM-12:30 PM
Christina Houfek joined Virginia Tech’s Applied Research Corporation in 2022 following her time as an Irregular Warfare Analyst at the Naval Special Warfare Command and as Senior Professional Staff at the Johns Hopkins University Applied Physics Laboratory. She holds an M.A. in Leadership in Education from Notre Dame of Maryland University and a Graduate Certificate in Terrorism Analysis awarded by the University of Maryland.
Dr. Maegen Nix, Ph.D. is a veteran and a former intelligence officer with 25 years of experience in the national security community and academia and currently serves as the Director of the Decision Science Division at Virginia Tech’s Applied Research Corporation. Her civilian career has focused on the development of portfolios related to irregular warfare and insurgencies, cybersecurity, critical infrastructure security, national communications, autonomous systems, and intelligence. Dr. Nix earned a Ph.D. in government and politics from the University of Maryland, an M.A. in political science from Virginia Tech, and a B.S. in political science from the U.S. Naval Academy.
Abstract: The Joint Test Concept: Reimagining T&E for the Modern Joint Environment
Improving the Quality of Test & Evaluation
The Joint force will likely be contested in all domains during the execution of distributed and potentially non-contiguous, combat operations. This challenge inspires the question, “How do we effectively reimagine efficient T&E within the context of expected contributions to complex Joint kill/effects webs?” The DOT&E sponsored Joint Test Concept applies an end-to-end capability lifecycle campaign of learning approach, anchored in mission engineering, and supported by a distributed live, virtual, constructive environment to assess material and non-material solutions’ performance, interoperability, and impact to service and Joint mission execution. Relying on input from the expanding JTC community of interest and human centered design facilitation, the final concept is intended to ensure data quality, accessibility, utility, and analytic value across existing and emergent Joint mission (kill/effects) webs for all systems under test throughout the entire capability lifecycle. Using modeling and simulation principles, the JTC team is developing an evaluation model to assess the impact of the JTC within the current T&E construct to identify the value proposition across a diverse stakeholder population.
Session Materials: https://dataworks.testscience.org/wp-content/uploads/formidable/23/Wed_1A_Houfek_Nix-1.zip
Christina Houfek and Kelli Esser
Leading Change: Applying Human Centered Design Facilitation Techniques
Day 2, Room: D 3:30 PM-5:00 PM
Christina Houfek joined Virginia Tech’s Applied Research Corporation in 2022 following her time as an Irregular Warfare Analyst at the Naval Special Warfare Command and as Senior Professional Staff at the Johns Hopkins University Applied Physics Laboratory. She holds a B.S. in behavioral sciences, an M.A. in Leadership in Education from Notre Dame of Maryland University and a Graduate Certificate in Terrorism Analysis awarded by the University of Maryland.
Abstract: Leading Change: Applying Human Centered Design Facilitation Techniques
Improving the Quality of Test & Evaluation
First introduced in 1987, modern design thinking was popularized by the Stanford Design School and the global design and innovation company, IDEO. Design thinking is now recognized as a “way of thinking which leads to transformation, evolution and innovation” and has been so widely accepted across industry and within the DoD, that universities offer graduate degrees in the discipline. Relying on the design thinking foundation, the Decision Science Division (DSD) of Virginia Tech Applied Research Corporation (VT-ARC) human centered design facilitation technique integrates related methodologies including liberating structures and open thinking. Liberating structures, are “simple and concrete tools that can enhance group performance in diverse organizational settings.” Open thinking, popularized by Dan Pontefract, provides a comprehensive approach to decision-making that incorporates critical and creative thinking techniques. The combination of these methodologies enables tailored problem framing, innovative solution discovery, and creative adaptability to harness collaborative analytic potential, overcome the limitations of cognitive biases, and lead change. DSD VT-ARC applies this approach to complex and wicked challenges to deliver solutions that address implementation challenges and diverse stakeholder requirements. Operating under the guiding principle that collaboration is key to success, DSD regularly partners with other research organizations, such as Virginia Tech National Security Institute (VT NSI), in human centered design activities to help further the understanding, use, and benefits of the approach. This experiential session will provide attendees with some basic human centered design facilitation tools and an understanding of how these techniques might be applied across a multitude of technical and non-technical projects.
Session Materials: https://dataworks.testscience.org/wp-content/uploads/formidable/23/Wed_MT3_Houfek_for_website.pdf
Cooper Klein
Cadet, United States Military Academy, West Point
Enhancing Battlefield Intelligence with ADS-B Change Detection
Day 2, Room: Cafe 5:00 PM-7:00 PM
Cooper Klein is an applied statistics and data science major from Seattle, Washington. A senior at West Point, he is researching fusion of air traffic control data to inform battlefield intelligence. Cooper will commission as a military intelligence officer in the United States Army this May. He represents West Point on the Triathlon Team where he pursues his passion of endurance sports.
Abstract: Enhancing Battlefield Intelligence with ADS-B Change Detection
Advancing Test & Evaluation of Emerging and Prevalent Technologies
The ability to detect change in flight patterns using air traffic control (ATC) communication can better inform battlefield intelligence. ADS-B (Automatic Dependent Surveillance Broadcast) technology has this capability to capture movement of both military and civilian aircraft over conflict zones. Leveraging the inclusivity of ADS-B in flight tracking and its widespread global availability, we focus on its application in understanding changes leading up to conflicts, with a specific case study on Ukraine.
In this presentation we analyze days leading up to Russia’s February 24 invasion to understand how ADS-B technology can indicate change in Russo-Ukrainian military movements. The proposed detection algorithm encourages the use of ADS-B technology in future intelligence efforts. The potential for fusion with GICB (Ground-initiated Comm-B) ATC communication and other modes of data is also explored.
This is a submission for the Student Poster Competition
Curtis Miller
Research Staff Member, IDA
A preview of functional data analysis for modeling and simulation validation
Day 2, Room: A 1:40 PM-3:10 PM
Dr. Curtis Miller is a research staff member of the Operational Evaluation Division at the Institute for Defense Analyses. In that role, he advises analysts on effective use of statistical techniques, especially pertaining to modeling and simulation activities and U.S. Navy operational test and evaluation efforts, for the division's primary sponsor, the Director of Operational Test and Evaluation. He obtained a PhD in mathematics from the University of Utah and has several publications on statistical methods and computational data analysis, including an R package. In the past, he has done research on topics in economics including estimating difference in pay between male and female workers in the state of Utah on behalf of Voices for Utah Children, an advocacy group.
Abstract: A preview of functional data analysis for modeling and simulation validation
Modeling and simulation (M&S) validation for operational testing often involves comparing live data with simulation outputs. Statistical methods known as functional data analysis (FDA) provides techniques for analyzing large data sets ("large" meaning that a single trial has a lot of information associated with it), such as radar tracks. We preview how FDA methods could assist M&S validation by providing statistical tools handling these large data sets. This may facilitate analyses that make use of more of the data available and thus allows for better detection of differences between M&S predictions and live test results. We demonstrate some fundamental FDA approaches with a notional example of live and simulated radar tracks of a bomber’s flight.
David Niblick
AI Evaluator, Army Test and Evaluation Command
Development, Test, and Evaluation of Small-Scale Artificial Intelligence Models
Day 3, Room: A 9:50 AM-11:50 AM
MAJ David Niblick graduated from the United States Military Academy at West Point in 2010 with a BS in Electrical Engineering. He served in the Engineer Branch as a lieutenant and captain at Ft. Campbell, KY with the 101st Airborne Division (Air Assault) and at Schofield Barracks, HI with the 130th Engineer Brigade. He deployed twice to Afghanistan ('11-'12 and '13-'14) and to the Republic of Korea ('15-'16). After company command, he attended Purdue University and received an MS in Electrical and Computer Engineering with a thesis in computer vision and deep learning. He instructed in the Department of Electrical Engineering and Computer Science at USMA, after which he transferred from the Engineer Branch to Functional Area 49 (Operations Research and Systems Analysis). He currently serves as an Artificial Intelligence Evaluator with Army Test and Evaluation Command at Aberdeen Proving Ground, MD.
Abstract: Development, Test, and Evaluation of Small-Scale Artificial Intelligence Models
As data becomes more commoditized across all echelons of the DoD, developing Artificial Intelligence (AI ) solutions, even at small scales, offer incredible opportunity for advanced data analysis and processing. However, these solutions require intimate knowledge of the data in question, as well as robust Test and Evaluation (T&E) procedures to ensure performance and trustworthiness. This paper presents a case study and recommendations for developing and evaluating small-scale AI solutions. The model automates an acoustic trilateration system. First, the system accurately identifies the precise times of acoustic events across a variable number of sensors using a neural network. It then corresponds the events across the sensors through a heuristic matching process. Finally, using the correspondences and difference of times, the system triangulates a physical location. We find that even a relatively simple dataset requires extensive understanding at all phases of the process. Techniques like data augmentation and data synthesis, which must capture the unique attributes of the real data, were necessary both for improved performance, as well as robust T&E. The T&E metrics and pipeline required unique approaches to account for the AI solution, which lacked traceability and explainability. As leaders leverage the growing availability of AI tools to solve problems within their organizations, strong data analysis skills must remain at the core of process.
Session Materials: https://dataworks.testscience.org/wp-content/uploads/formidable/23/Thrs_4A_Niblick.pptx
Dr. David "Fuzzy" Wells Other
Principal Cyber Simulationist, The MITRE Corporation
Recommendations for Cyber Test & Evaluation of Space Systems
Day 2, Room: B 10:30 AM-12:30 PM
Dr. David “Fuzzy” Wells is Principal Cyber Simulationist for The MITRE Corporation supporting the National Cyber Range Complex. Dr. Wells is the former Director of U.S. Indo-Pacific Command’s Cyber War Innovation Center where he built the first combatant command venue for cyber testing, training, and experimentation; managed the Command's joint cyber innovation & experimentation portfolio; and executed cyber range testing and training events for service, joint, and coalition partners. Dr. Wells was the first Air Force officer to obtain a Ph.D. in Modeling, Virtual Environments, and Simulation from the Naval Postgraduate School and M.S. in Modeling & Simulation from the Air Force Institute of Technology. He is a Certified Modeling & Simulation Professional Charter Member.
Abstract: Recommendations for Cyber Test & Evaluation of Space Systems
Advancing Test & Evaluation of Emerging and Prevalent Technologies
This presentation marks the conclusion of a study aimed to understand the current state of cyber test and evaluation (T&E) activities that occur within the space domain. This includes topics such as cyber T&E challenges unique to the space domain (e.g., culture and motivations, space system architectures and threats, cyber T&E resources), cyber T&E policy and guidance, and results from a space cyber T&E survey and set of interviews. Recommendations include establishing a cyber T&E helpdesk and rapid response team, establishing contracting templates, incentivizing space cyber T&E innovation, growing and maturing the space cyber T&E workforce, and learning from cyber ranges.
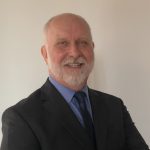
Dean Thomas
Researcher, George Mason University
What drove the Carrington event? An analysis of currents and geospace regions.
Day 2, Room: Cafe 5:00 PM-7:00 PM
Dr. Dean Thomas works with the George Mason University Space Weather Lab, supporting a collaborative effort led by NASA Goddard. His research focuses on space weather phenomena related to solar storms. During these storms, the sun can eject billions of tons of plasma into space over just a few hours. Most of these storms miss the Earth, but they can create large geomagnetically-induced currents (GIC), cause electrical blackouts, force airliners to change course, and damage satellites. Dr. Thomas’ research examines some of the largest storms observed, and the major factors that drive effects observed on the earth’s surface. Earlier in his career, he was Deputy Director for the Operational Evaluation Division at the Institute for Defense Analyses, helping to manage a team of 150 researchers. The division supports the Director, Operational Test and Evaluation (DOT&E) within the Pentagon. DOT&E is responsible for operational testing of new military systems including aircraft, ships, ground vehicles, sensors, weapons, and information technology systems. Dean Thomas received his PhD in Physics from Stony Brook University in 1987, and in 1982, his Bachelor of Science in Engineering Physics from the Colorado School of Mines.
Abstract: What drove the Carrington event? An analysis of currents and geospace regions.
Sharing Analysis Tools, Methods, and Collaboration Strategies
The 1859 Carrington Event is the most intense geomagnetic storm in recorded history. This storm produced large changes to the geomagnetic field observed on the Earth’s surface, damaged telegraph systems, and created aurora visible over large portions of the earth. The literature provides numerous explanations for which phenomena drove the observed effects. Previous analyses typically relied upon on the historic magnetic field data from the event, newspaper reports, and empirical models. These analyses generally focus on whether one current system (e.g., magnetospheric currents) is more important than another (e.g., ionospheric currents). We expand the analysis by using results from the Space Weather Modeling Framework (SWMF), a complex magnetohydrodynamics code, to compute the contributions that various currents and geospace regions make to the northward magnetic field on the Earth’s surface. The analysis considers contributions from magnetospheric currents, ionospheric currents, and gap region field-aligned currents (FACs). In addition, we evaluate contributions from specific regions: the magnetosheath (between the earth and the sun), near Earth (within 6.6 earth radii), and the neutral sheet (behind the earth). Our analysis indicates that magnetic field changes observed during the Carrington Event involved a combination of current systems and regions rather than being driven by one specific current or region.

Dhruv Patel
Research Staff Member, IDA
A practitioner's framework for federated model V&V resource allocation
Day 2, Room: A 1:40 PM-3:10 PM
Dhruv is a research staff member at the Institute of Defense Analyses and obtained his Ph.D. in Statistics and Operations Research from the University of North Carolina at Chapel Hill.
Abstract: A practitioner's framework for federated model V&V resource allocation
Recent advances in computation and statistics led to an increasing use of Federated Models for system evaluation. A federated model is a collection of sub models interconnected where the outputs of a sub-model act as inputs to subsequent models. However, the process of verifying and validating federated models is poorly understood and testers often struggle with determining how to best allocate limited test resources for model validation. We propose a graph-based representation of federated models, where the graph encodes the connections between sub-models. Vertices of the graph are given by sub-models. A directed edge between vertex a and b is drawn if a inputs into b. We characterize sub-models through vertex attributes and quantify their uncertainties through edge weights. The graph-based framework allows us to quantify the uncertainty propagated through the model and optimize resource allocation based on the uncertainties.
Session Materials: https://dataworks.testscience.org/wp-content/uploads/formidable/23/Wed_2A_Patel.pptx
Dr. Douglas Schmidt
Director, Operational Test & Evaluation
Day 2, Room: A+B 8:45 AM-9:25 AM
Dr. Douglas C. Schmidt was sworn in as Director, Operational Test and Evaluation (DOT&E) on April 8, 2024. A Presidential appointee confirmed by the United States Senate, he serves as the senior advisor to the Secretary of Defense on operational and live fire test and evaluation of Department of Defense weapon systems.
Prior to DOT&E, Dr. Schmidt served as the Cornelius Vanderbilt Professor of Engineering in Computer Science, the Associate Chair of Computer Science, and a Senior Researcher at the Institute for Software Integrated Systems at Vanderbilt University.
From 2010 to 2014, Dr. Schmidt was a member of the Air Force Scientific Advisory Board (AF SAB), where he served as Vice Chair of studies on cyber situational awareness for Air Force mission operations and on sustaining hardware and software for U.S. aircraft. He also served on the advisory board for the joint Army/Navy Future Airborne Capability Environment initiative. From 2000 to 2003, Dr. Schmidt served as a program manager in the Defense Advanced Research Projects Agency (DARPA) Information Exploitation Office and Information Technology Office.
Dr. Schmidt is an internationally renowned and widely cited researcher. Dr. Schmidt received Bachelor and Master of Arts degrees in Sociology from the College of William and Mary, and Master of Science and Doctorate degrees in Computer Science from the University of California, Irvine.
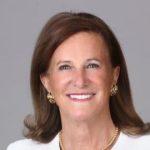
Dr. Elisabeth Paté-Cornell
NASA Advisory Council/Professor, Stanford University
Day 2, Room: A+B 9:25 AM-10:05 AM
M. Elisabeth Paté-Cornell is the Burt and Deedee MacMurtry Professor in the department of Management Science and Engineering at Stanford University, a department which she founded then chaired from January 2000 to June 2011. She was elected to the National Academy of Engineering in 1995, to its Council (2001-2007), and to the French Académie des Technologies (2003). She was a member of the President’s Intelligence Advisory Board (2001-2004; 2006-2008), of the Boards of Trustees of the Aerospace Corporation (2004-2016), of InQtel (2006-2017) and of the Draper Corporation (2009-2016). She is a member of the NASA Advisory Council, and currently co-chairs the National Academies Committee on methods of analysis of the risks of nuclear war and nuclear terrorism. She is a world leader in engineering risk analysis and risk management. Her research and that of her Engineering Risk Research Group at Stanford have focused on the inclusion of technical and management factors in probabilistic risk analysis models with applications to the NASA shuttle tiles, offshore oil platforms and medical systems. Since 2001, she has combined risk analysis and game analysis to assess intelligence information and the risks of terrorist attacks. More recently her research has centered on the failure risks of cyber systems and artificial intelligence in risk management decision. She is past president (1995) and fellow of the Society for Risk Analysis, and a fellow of the Institute for Operations Research and Management Science. She received the 2021 IEEE Ramo medal for Systems Science and Engineering, and the 2022 PICMET Award in Engineering Risk Management. She has been a consultant to many industrial firms and government organizations. She has authored or co-authored more than a hundred papers in refereed journals and conference proceedings and has received several best-paper awards from professional organizations and peer-reviewed journals.

Fatemeh Salboukh
PhD Student, University of Massachusetts Dartmouth
Enhancing Multiple Regression-based Resilience Model Prediction with Transfer Function
Day 2, Room: B 3:30 PM-5:00 PM
Fatemeh Salboukh is a PhD student in the Department of Engineering and Applied Science at the University of Massachusetts Dartmouth. She received her Master’s from the University of Allame Tabataba’i in Mathematical Statistics (September, 2020) and Bachelor’s degree from Yazd University (July, 2018) in Applied Statistics.
Abstract: Enhancing Multiple Regression-based Resilience Model Prediction with Transfer Function
Sharing Analysis Tools, Methods, and Collaboration Strategies
Resilience engineering involves creating and maintaining systems capable of efficiently managing disruptive incidents. Past research in this field has employed various statistical techniques to track and forecast the system's recovery process within the resilience curve. However, many of these techniques fall short in terms of flexibility, struggling to accurately capture the details of shocks. Moreover, most of them are not able to predict long-term dependencies. To address these limitations, this paper introduces an advanced statistical method, the transfer function, which effectively tracks and predicts changes in system performance when subjected to multiple shocks and stresses of varying intensity and duration. This approach offers a structured methodology for planning resilience assessment tests tailored to specific shocks and stresses and guides the necessary data collection to ensure efficient test execution. Although resilience engineering is domain-specific, the transfer function is a versatile approach, making it suitable for various domains. To assess the effectiveness of the transfer function model, we conduct a comparative analysis with the interaction regression model, using historical data on job losses during the 1980 recessions in the United States. This comparison not only underscores the strengths of the transfer function in handling complex temporal data but also reaffirms its competitiveness compared to existing methods. Our numerical results using goodness of fit measures provide compelling evidence of the transfer function model's enhanced predictive power, offering an alternative for advancing resilience prediction in time series analysis.
Session Materials: https://dataworks.testscience.org/wp-content/uploads/formidable/23/Wed_SS_Salboukh.pptx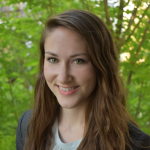
Gabriela Parasidis
Lead Systems Engineer, MITRE
Mission Engineering
Day 2, Room: C 1:40 PM-3:10 PM
Gabriela Parasidis is a Lead Systems Engineer in the MITRE Systems Engineering Innovation Center. She applies Digital Engineering to Mission Engineering and Systems of Systems (SoS) Engineering to support Department of Defense acquisition decisions. She has led research in hypersonics, including analyses related to flight dynamics, aerodynamics, aerothermodynamics, and structural loading. She holds a B.S. in Mechanical Engineering from Cornell University and a M.S. in Systems Engineering from Johns Hopkins University.
Abstract: Mission Engineering
Sharing Analysis Tools, Methods, and Collaboration Strategies
The US Department of Defense (DoD) has expanded their emphasis on the application of systems engineering approaches to ‘missions’. As originally defined in the Defense Acquisition Guidebook, Mission Engineering (ME) is “the deliberate planning, analyzing, organizing, and integrating of current and emerging operational and system capabilities to achieve desired operational mission effects”. Based on experience to date, the new definition reflects ME as an “an interdisciplinary approach and process encompassing the entire technical effort to analyze, design, and integrate current and emerging operational needs and capabilities to achieve desired mission outcomes”. This presentation presents the current mission engineering methodology, describes how it is currently being applied, and explores the role of T&E in the ME process. Mission engineering is applying systems engineering to missions – that is, engineering a system of systems, (including organizations, people and technical systems) to provide desired impact on mission or capability outcomes. Traditionally, systems of systems engineering focused on designing systems or systems of systems to achieve specified technical performance. Mission engineering goes one step further to assess whether the system of systems, when deployed in a realistic user environment, achieves the user mission or capability objectives. Mission engineering applies digital model-based engineering approaches to describe the sets of activities in the form of ‘mission threads’ (or activity models) needed to execute the mission and then adds information on players and systems used to implement these activities in the form of ‘mission engineering threads.’ These digital ‘mission models’ are then implemented in operational simulations to assess how well they achieve user capability objectives. Gaps are identified and models are updated to reflect proposed changes, including reorientation of systems and insertion of new candidate solutions, and which are assessed relative to changes in overall mission effectiveness.
Session Materials: https://dataworks.testscience.org/wp-content/uploads/formidable/23/Wed_2C_Parasidis.pptx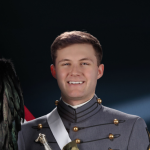
Garrett Chrisman
Cadet, United States Military Academy
Wildfire Burned Area Mapping Using Sentinel-1 SAR and Sentinel-2 MSI with Convolutional Neural Networks
Day 2, Room: B 3:30 PM-5:00 PM
Garrett Chrisman is currently an undergraduate cadet at the United States Military Academy, West Point, majoring in Applied Statistics and Data Science. His academic focus includes Python, R, and machine learning applications. Garrett has engaged in research on wildfire severity assessment using Convolutional Neural Networks and satellite imagery. Additionally, he has held leadership roles, including being the Treasurer for his class, Captain of the Cycling team, and the President of the Finance Club. His work demonstrates a blend of technical skill and leadership.
Abstract: Wildfire Burned Area Mapping Using Sentinel-1 SAR and Sentinel-2 MSI with Convolutional Neural Networks
The escalating environmental and societal repercussions of wildfires, underscored by the occurrence of four of the five largest wildfires in Colorado within the past five years, necessitate efficient mapping of burned areas to enhance emergency response and fire control strategies. This study investigates the potential of Synthetic Aperture Radar (SAR) capabilities of the Sentinel-1 satellite, in conjunction with optical imagery from Sentinel-2, to expedite the assessment of wildfire conditions and progression. Our research is structured into four distinct cases; each applied to our dataset comprising seven Colorado wildfires. In each case, we iteratively refined our methods to mitigate the inherent challenges associated with SAR data. Our results demonstrate that while SAR imagery may not match the precision of traditional methodologies, it offers a valuable trade-off by providing a sufficiently accurate estimate of burned areas in significantly less time.
Furthermore, we developed a deep learning framework for predicting burn severity using both Sentinel-1 SAR and Sentinel-2 MSI data acquired during wildfire events. Our findings underscore the potential of spaceborne imagery for real-time burn severity prediction, providing valuable insights for the effective management of wildfires. This research contributes to the advancement of wildfire monitoring and response, particularly in regions prone to such events like Colorado, and underscores the significance of remote sensing technologies in addressing contemporary environmental challenges.

Gavin Collins
R&D Statistician, Sandia National Laboratories
Bayesian Projection Pursuit Regression
Day 3, Room: C 1:00 PM-3:00 PM
Gavin received a joint BS/MS degree in statistics from Brigham Young University in 2018, then went on to complete a PhD in statistics at Ohio State University in 2023. He recently started as a full-time R&D statistician at Sandia National Laboratories in Albuquerque, New Mexico. His research interests include Bayesian statistics, nonparametric regression, functional data analysis, and emulation and calibration of computational models.
Abstract: Bayesian Projection Pursuit Regression
Sharing Analysis Tools, Methods, and Collaboration Strategies
In projection pursuit regression (PPR), a univariate response variable is approximated by the sum of M "ridge functions," which are flexible functions of one-dimensional projections of a multivariate input variable. Traditionally, optimization routines are used to choose the projection directions and ridge functions via a sequential algorithm, and M is typically chosen via cross-validation. We introduce a novel Bayesian version of PPR, which has the benefit of accurate uncertainty quantification. To infer appropriate projection directions and ridge functions, we apply novel adaptations of methods used for the single ridge function case (M=1), called the Bayesian Single Index Model; and use a Reversible Jump Markov chain Monte Carlo algorithm to infer the number of ridge functions $M$. We evaluate the predictive ability of our model in 20 simulated scenarios and for 23 real datasets, in a bake-off against an array of state-of-the-art regression methods. Finally, we generalize this methodology and demonstrate the ability to accurately model multivariate response variables. Its effective performance indicates that Bayesian Projection Pursuit Regression is a valuable addition to the existing regression toolbox.
Session Materials: https://dataworks.testscience.org/wp-content/uploads/formidable/23/Thrs_CS3_Collins.pdf
Gina Sigler
STAT COE contractor, HII/STAT COE
Failure Distributions for Parallel Dependent Identical Weibull Components
Day 3, Room: B 9:50 AM-11:50 AM
Dr. Gina Sigler, contractor with Huntington Ingalls Industries (HII), is the DoD Program Lead at the Scientific Test and Analysis Techniques (STAT) Center of Excellence (COE). She has been working at the STAT COE since 2018, where she provides rigorous test designs and best practices to programs across the DOD. Before joining the STAT COE, she worked as a faculty associate in the Statistics Department at the University of Wisconsin-Madison for three years. She earned a B.S. degree in statistics from Michigan State University, an M.S. in statistics from the University of Wisconsin-Madison, and a PhD in Applied Mathematics-Statistics from the Air Force Institute of Technology.
Abstract: Failure Distributions for Parallel Dependent Identical Weibull Components
Solving Program Evaluation Challenges
For a parallel system, when one component fails, the failure distribution of the remaining components will have an increased failure rate. This research takes a novel approach to finding the associated failure distribution of the full system using ordinal statistic distributions for correlated Weibull components, allowing for unknown correlations between the dependent components. A Taylor series approximation is presented for two components; system failure time distributions are also derived for two failures in a two component system, two failures in an n component system, three failures in a three component system, and k failures in an component system. Additionally, a case study is presented on aircraft turnbuckles. Simulated data is used to illustrate how the derived formulas can be used to create a maintenance plan for the second turnbuckle in the two component system.
Session Materials: https://dataworks.testscience.org/wp-content/uploads/formidable/23/Thrs_4B_Sigler.pdf
Giri Gopalan
Scientist, Los Alamos
A Statistical Framework for Benchmarking Foundation Models with Uncertainty
Day 2, Room: C 3:30 PM-5:00 PM
Giri Gopalan is a staff scientist in the statistical sciences group at Los Alamos National Laboratory. His current research interests include statistics in the physical sciences, spatial and spatiotemporal statistics, and statistical uncertainty quantification. Prior to his present appointment, Giri was Assistant Professor at
California Polytechnic State University and a Visiting Assistant Professor at the University of California Santa Barbara, both in statistics. He has taught courses such as time series, mathematical statistics, and probability for engineering students.
Abstract: A Statistical Framework for Benchmarking Foundation Models with Uncertainty
Advancing Test & Evaluation of Emerging and Prevalent Technologies
Modern artificial intelligence relies upon foundation models (FMs), which are prodigious, multi-purpose machine learning models, typically deep neural networks, trained on a massive data corpus. Many benchmarks assess FMs by evaluating their performances on a battery of tasks for which the FMs are adapted to solve, but uncertainty is usually not accounted for in such benchmarking practices. This talk will present statistical approaches for performing uncertainty quantification with benchmarks meant to compare FMs. We demonstrate bootstrapping of task evaluation data, Bayesian hierarchical models for task evaluation data, rank aggregation techniques, and visualization of model performance under uncertainty with different task weightings. The utility of these statistical approaches is illustrated with real machine learning benchmark data, and a crucial finding is that the incorporation of uncertainty leads to less clear-cut distinctions in FM performance than would otherwise be apparent.
Session Materials: https://dataworks.testscience.org/wp-content/uploads/formidable/23/Wed_3C_Gopalan-1.pdf
Giuseppe Cataldo
Planetary Protection Lead, NASA
Global Sensitivity Analyses for Test Planning under Constraints with Black-box Models
Day 3, Room: B 1:00 PM-3:00 PM
Giuseppe Cataldo leads the NASA planetary protection efforts for the last mission of the Mars Sample Return program. In this role, he oversees the efforts aimed at safely returning rock and atmosphere samples from Mars without contaminating the Earth's biosphere with potentially hazardous biological particles. Previously, he was the chief engineer of NASA's EXCLAIM mission and the near-infrared camera NASA contributed to the PRIME telescope. He worked on the James Webb Space Telescope from 2014 to 2020 and on a variety of other NASA missions and technology development projects. His expertise is in the design, testing and management of space systems, gained over 10+ years at NASA and by earning his doctorate at the Massachusetts Institute of Technology. Giuseppe is the recipient of numerous awards including NASA's Early Career Public Achievement Medal and Mentoring Award. He speaks six languages, plays the violin, loves swimming and skiing as well as helping the homeless with his friends and wife.
Abstract: Global Sensitivity Analyses for Test Planning under Constraints with Black-box Models
Sharing Analysis Tools, Methods, and Collaboration Strategies
This work describes sensitivity analyses performed on complex black-box models used to support experimental test planning under limited resources in the context of the Mars Sample Return program, which aims at bringing to Earth rock and atmospheric samples from Mars. We develop a systematic workflow that allows the analysts to simultaneously obtain quantitative insights on key drivers of uncertainty, on the direction of impact, and the presence of interactions. We apply novel optimal transport-based global sensitivity measures to tackle the multivariate nature of the output. On the modeling side, we apply multi-fidelity techniques that leverage low-fidelity models to speed up the calculations and make up for the limited amount of high-fidelity samples, while keeping these in the loop for accuracy guarantees. The sensitivity analysis reveals insights useful for the analysts to understand the model's behavior and identify the factors to focus on during testing in order to maximize the value of information extracted from them to ensure mission success when limited resources are available.
Session Materials: https://dataworks.testscience.org/wp-content/uploads/formidable/23/Thrs_CS2_Cataldo.pptx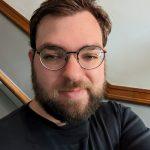
Harris Bernstein
Data Scientist, Johns Hopkins University Applied Physics Lab
Bayesian Reliability Growth Planning for Discrete Systems
Day 3, Room: B 9:50 AM-11:50 AM
Harris Bernstein is a data scientist with experience in implementing complex machine learning models and data visualization techniques while collaborating with scientist and engineers across varying domains.
He currently serves as a Senior Data Scientist at the Johns Hopkins University Applied Physics Lab in the System Performance Analysis Group. His current research includes optimal experimental designs for machine learning methods and incorporating statistical models inside of digital engineering environments.
Previously he worked on the Large Hadron Collider beauty (LHCb) experiment at Syracuse University as part of the Experimental Particle Physics Group. There he was the principle analyst on a branching fraction measurement that incorporated complex statistical modeling that accounted several different kinds of physical processes.
Education:
Doctor of Philosophy. Physics. Syracuse University. New York.
Bachelor of Science. Physics. Pennsylvania State University. Pennsylvania.
Abstract: Bayesian Reliability Growth Planning for Discrete Systems
Improving the Quality of Test & Evaluation
Developmental programs for complex systems with limited resources often face the daunting task of predicting the time needed to achieve system reliability goals.
Traditional reliability growth plans rely heavily on operational testing. They use confidence estimates to determine the required sample size, and then work backward to calculate the amount of testing required during the developmental test program to meet the operational test goal and satisfy a variety of risk metrics. However, these strategies are resource-intensive and do not take advantage of the information present in the developmental test period.
This presentation introduces a new method for projecting the reliability growth of a discrete, one-shot system. This model allows for various corrective actions to be considered, while accounting for both the uncertainty in the corrective action effectiveness and the management strategy used to parameterize those actions. Solutions for the posterior distribution on the system reliability are found numerically, while allowing for a variety of prior distributions on the corrective action effectiveness and the management strategy. Additionally, the model can be extended to account for system degradation across testing environments. A case study demonstrates how this model can use historical data with limited failure observations to inform its parameters, making it even more valuable for real-world applications.
This work builds upon previous research in Reliability Growth planning from Drs. Brian Hall and Martin Wayne.
Himanshu Upadhyay
Associate Professor -ECE, Florida International University
Generative AI -Large Language Model Introduction
Day 2, Room: C 3:30 PM-5:00 PM
Dr Himanshu Upadhyay is serving Florida International University for the past 23 years, leading the AI & Cyber Center of Excellence at Applied Research Center. He is working as an Associate Professor in Electrical & Computer Engineering teaching Artificial Intelligence and Cybersecurity courses. His research focuses on Artificial Intelligence, Machine Learning, Deep Learning, Generative AI, Cyber Security, Big Data, Cyber Analytics/Forensics, Malware Analysis and Blockchain. He has published multiple papers in reputed journals & conferences and is mentoring AI & Cyber Fellows, undergraduate and graduate students supporting multiple AI & Cybersecurity research projects from various federal agencies.
Abstract: Generative AI -Large Language Model Introduction
Generative artificial intelligence (AI) is a rapidly advancing field and transformative technology that involves the creation of new content. Generative AI encompasses AI models that produce novel data, information, or documents in response to the prompts. This technology has gained significant attention due to the emergence of models like DALL-E , Imagen, and ChatGPT. Generative AI excels in generating content across various domains. The versatility of Generative AI extends to generating text, software code, images, videos, and music by statistically analyzing patterns in training data.
One of the most prominent applications of Generative AI is ChatGPT, developed by OpenAI. ChatGPT is a sophisticated language model trained on vast amounts of text data from diverse sources. It can engage in conversations, answer questions, write essays, generate code snippets, and more. Generative AI's strengths lie in its ability to produce diverse and seemingly original outputs quickly.
Large Language Models (LLMs) are advanced deep learning algorithms that can understand, summarize, translate, predict, and generate content using extensive datasets. These models work by being trained on massive amounts of data, deriving relationships between words and concepts, and then using transformer neural network processes to understand and generate responses.
LLMs are widely used for tasks like text generation, translation, content summarization, rewriting content, classification, and categorization. They are trained on huge datasets to understand language better and provide accurate responses when given prompts or queries. The key algorithms used in LLMs include:
• Word Embedding: This algorithm represents the meaning of words in a numerical format, enabling the AI model to process and analyze text data efficiently.
• Attention Mechanisms: These algorithms allow the AI to focus on specific parts of input text, such as sentiment-related words, when generating an output, leading to more accurate responses.
• Transformers: Transformers are a type of neural network architecture designed to solve sequence-to-sequence tasks efficiently by using self-attention mechanisms. They excel at handling long-range dependencies in data sequences. They learn context and meaning by tracking relationships between elements in a sequence.
This presentation will focus on basics of large language models, algorithms, and applications to nuclear decommissioning knowledge management.
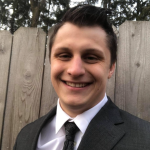
Jacob Langley
Data Science Fellow II, IDA
A Comparative Analysis of AI Topic Coverage Across Degree Programs
Day 2, Room: Cafe 5:00 PM-7:00 PM
Jacob Langley is a Data Science Fellow at the Institute for Defense Analyses (IDA). He holds a master's degree in economics and a graduate certificate in statistics. At IDA, Jacob has been serving as an AI researcher for the Science and Technology Policy Institute and assists the Chief Digital AI Office (CDAO) of the DoD.
Abstract: A Comparative Analysis of AI Topic Coverage Across Degree Programs
This study employs cosine similarity topic modeling to analyze the curriculum content of AI (Artificial Intelligence) bachelor’s and master’s degrees, comparing them with Data Science bachelor’s and master’s degrees, as well as Computer Science (CS) bachelor’s degrees with concentrations in AI. 97 programs total were compared. 52 topics of interest were identified at the course level. The analysis creates a representation for each of the 52 identified topics by compiling course descriptions whose course title matches into a bag-of-words. Cosine similarity is employed to compare the topic coverage of each program against all course descriptions of required courses from within that program.
Subsequently, Kmeans and Hierarchical clustering methods are applied to the results to investigate potential patterns and similarities among the programs. The primary objective was to discern whether there are distinguishable differences in the topic coverage of AI degrees in comparison to CS bachelor’s degrees with AI concentrations and Data Science degrees.
The findings reveal a notable similarity between AI bachelor’s degrees and CS bachelor’s degrees with AI concentrations, suggesting a shared thematic focus. In contrast, both AI and CS bachelor’s programs exhibit distinct dissimilarities in topic coverage when compared to Data Science bachelor’s and master's degrees. A notable difference being that the Data Science degrees exhibit much higher coverage of math and statistics than the AI and CS bachelor’s degrees. This research contributes to our understanding of the academic landscape, and helps scope the field as public and private interest into AI is at an all-time high.

Dr. James Wisnowski
Adsurgo
Design and Analysis of Experiments – Next-Level Methods with Case Studies
Day 1, Room: A 9:00 AM-4:00 PM
Dr. James Wisnowski, co-founder and principal consultant at Adsurgo, leads the enterprise consulting and government divisions of Adsurgo. Dr. Wisnowski has consulting experience and expertise in applied statistics, program management, strategic planning, military operations, design of experiments, reliability engineering, quality engineering, data mining, text analytics, simulation modelling, along with operations research analysis. He has published refereed journal articles and texts in addition to presenting consulting results, new research, and short courses at conferences worldwide. He retired from the US Air Force as an officer with 20 years of service as an acquisition, test, personnel, and force structure analyst in addition to having significant leadership responsibilities as a squadron commander, joint staff officer, and Air Force Academy professor.
Abstract: Design and Analysis of Experiments – Next-Level Methods with Case Studies
This is the short course for you if you are familiar with the fundamental techniques in the science of test and want to learn useful, real-world, and advanced methods applicable in the DoD/NASA test community. The focus will be on use cases not typically covered in most short courses. JMP software will primarily be used, and datasets will be provided for you to follow along many of the hands-on demonstrations of practical case studies. Design topics will include custom design of experiments tips, choosing optimality criteria, creating designs from existing runs, augmenting adaptively in high gradient regions, creating designs with constraints, repairing broken designs, mixture design intricacies, modern screening designs, designs for computer simulation, accelerated life test, and measurement system testing. Analysis topics will include ordinary least squares, stepwise, and logistic regression, generalized regression (LASSO, ridge, elastic net), model averaging (to include Self-Validated Ensemble Models), random effects (split-plot, repeated measures), comparability/equivalence, functional data analysis (think your data is a curve), nonlinear approaches and multiple response optimization and trade-space analysis. The day will finish with an hour-long Q&A session to help solve your specific T&E challenges.
Session Materials: https://dataworks.testscience.org/wp-content/uploads/formidable/23/DATAWorks-2024-Next-Level-DOE-Short-Course-Materials.zip
James Starling
Associate Professor, United States Military Academy
Creating Workflows for Synthetic Data Generation and Advanced Military Image Classificatio
Day 3, Room: A 1:00 PM-3:00 PM
(please note that we would like to present work from both FSU and USMA during this presentation. We plan on having 5-7 presenters provide their insights on a handful of items related to the creation and use of synthetic data generation in Unreal Engine)
Dr. James K. Starling is an Associate Professor and Director for the Center for Data Analysis and Statistics at the United States Military Academy, West Point. He has served in the United States Army as an Artilleryman and an Operations Research and Systems Analysis (ORSA) analyst for over 23 years. His research interests include military simulations, optimization, remote sensing, and object detection and recognition.
Abstract: Creating Workflows for Synthetic Data Generation and Advanced Military Image Classificatio
Sharing Analysis Tools, Methods, and Collaboration Strategies
The US Government has a specific need for tools that intelligence analysts can use to search and filter data effectively. Artificial Intelligence (AI), through the application of Deep Neural Networks (DNNs) can assist in a multitude of military applications, requiring a constant supply of relevant data sets to keep up with the always-evolving battlefield. Existing imagery does not adequately represent the evolving nature of modern warfare; therefore, finding a way to simulate images of future conflicts could give us a strategic advantage against our adversaries. Additionally, using physical cameras to capture sufficient various lighting and environmental conditions is nearly impossible. The technical challenge in this area is to create software tools for edge computing devices integrated with cameras to process the video feed locally without having to send the video data through bandwidth-constrained networks to servers in data centers. The ability to collect and process data locally, often in austere environments, can accelerate decision making and action taken in response to emergency situations. An important part of this challenge is to create labeled datasets that are relevant to the problem and are needed for training the edge-efficient AI. Teams from Fayetteville State University (FSU) and The United States Military Academy (USMA) will present their proposed workflows that will enable accurate detection of various threats using Unreal Engine (UE) to generate synthetic training data. In principle, production of synthetic data is unlimited and can be customized to location, various environmental variables, and human and crowd characteristics. Together, both teams address the challenges of realism and fidelity; diversity and variability; and integration with real data.
The focus of the FSU team is on creating semi-automated workflows to create simulated human-crowd behaviors and the ability to detect anomalous behaviors. It will provide methods of specifying collective behaviors to create crowd simulations of many human agents, and for selecting a few of those agents to exhibit behaviors that are outside of the defined range of normality. The analysis is needed for rapid detection of anomalous activities that can pose security threats and cost human lives.
The focus of the USMA team will be in creating semi-autonomous workflows that evaluate the ability of DNNs to identify key military assets under various environmental conditions, specifically armored vehicles and personnel. We aim to vary environmental parameters to simulate varying light conditions and introduce obscuration experiments using artificial means like smoke and natural phenomena like fog to add complexity to the scenarios. Additionally, the USMA team will explore a variety of camouflage patterns and various levels of defilade.
The outcome of both teams is to provide workflow solutions that maximize the use of UE to provide realistic datasets that simulate future battlefields and emergency scenarios for evaluating and training existing models. These studies pave the way for creating advanced models trained specifically for military application. Creating adaptive models that can keep up with today’s evolving battlefield will give the military a great advantage in the race for artificial intelligence applications.

James Theimer
Operations Research Analyst, HS COBP
Using Bayesian Network of subsystem statistical models to assess system behavior
Day 2, Room: A 1:40 PM-3:10 PM
Dr. James Theimer is a Scientific Test and Analysis Techniques Expert employed by Huntington Ingles Industries Technical Solutions and working to support the Homeland Security Center of Best Practices.
Dr. Theimer worked for Air Force Research Laboratory and predecessor organizations for more than 35 years. He worked on modeling and simulation of sensors systems and supporting devices. His doctoral research was on modeling pulse formation in fiber lasers. He worked with a semiconductor reliability team as a reliability statistician and led a team which studied statistical validation of models of automatic sensor exploitation systems. This team also worked with programs to evaluate these systems.
Dr. Theimer has a PhD in Electrical Engineering from Rensselaer Polytechnic Institute, and MS in Applied Statistics from Wright State University, and MS in Atmospheric Science from SUNY Albany and a BS in Physics from University of Rochester.
Abstract: Using Bayesian Network of subsystem statistical models to assess system behavior
Situations exists when a system-level test is rarely accomplished or simply not feasible. When subsystem testing is available, to include creating a subsystem statistical model, an approach is required to combine these models. A Bayesian Network (BN) is an approach to address this problem. A BN models system behavior using subsystem statistical models. The system is decomposed into a network of subsystems and the interactions between the subsystems are described. Each subsystem is in turn described by a statistical model which determines the subjective probability distribution of the outputs given a set of inputs. Previous methods have been developed for validating performance of the subsystem models and subsequently what can be known about system performance. This work defined a notional system, created the subsystem statistical models, generated synthetic data, and developed the Bayesian Network.
Then, subsystem models are validated followed by a discussion on how system level information is derived from the Bayesian Network.
James Ferry
Principal Research Scientist, Metron, Inc.
Dynamo: Adaptive T&E via Bayesian Decision Theory
Day 3, Room: D 9:50 AM-11:50 AM
James Ferry’s research focuses on the problems of the Defense and Intelligence communities. His interests encompass the application of Bayesian methods to a variety of domains: Test & Evaluation, tracking and data association for kinematic and non-kinematic data, and the synthesis of classical detection and tracking theory with the modern theory of networks. Prior to Metron, he worked in computational fluid dynamics at UIUC, specializing in multiphase flow and thermal convection. Dr. Ferry holds a Ph.D. and M.S. in applied mathematics from Brown University and an S.B. in mathematics from MIT.
Abstract: Dynamo: Adaptive T&E via Bayesian Decision Theory
Improving the Quality of Test & Evaluation
The Dynamo paradigm for T&E compares a set of test options for a system by computing which of them provides the greatest expected operational benefit relative to the cost of testing. This paradigm will be described and demonstrated for simple, realistic cases. Dyanmo stands for DYNAmic Knowledge + MOneyball. These two halves of Dynamo are its modeling framework and its chief evaluation criterion, respectively.
A modeling framework for T&E is what allows test results (and domain knowledge) to be leveraged to predict operational system performance. Without a model, one can only predict, qualitatively, that operational performance will be similar to test performance in similar environments. For quantitative predictions one can formulate a model that inputs a representation of an operational environment and outputs the probabilities of the various possible outcomes of using the system there. Such models are typically parametric: they have a set of unknown parameters to be calibrated during test. The more knowledge one has about a suitable model’s parameters, the better predictions one can make about the modeled system’s operational performance. The Bayesian approach to T&E encodes this knowledge as a probability distribution over the model parameters. This knowledge is initialized with data from previous testing and with subject matter expertise, and it is “dynamic” because it is updated whenever new test results arrive.
An evaluation criterion is a metric for the operational predictions provided by the modeling framework. One type of metric is about whether test results indicate a system meets requirements: this question can be addressed with increasing nuance as one employs more sophisticated modeling frameworks. Another type of metric is how well a test design will tighten knowledge about model parameters, regardless of what the test results themselves are. The Dynamo paradigm can leverage either, but it uses a “Moneyball” metric for recommending test decisions. A Moneyball metric quantifies the expected value of the knowledge one would gain from testing (whether from an entire test event, or from just a handful of trials) in terms of the operational value this knowledge would provide. It requires a Bayesian modeling framework so that incremental gains in knowledge can be represented and measured. A Moneyball metric quantifies stakeholder preferences in the same units as testing costs, which enables a principled cost/benefit analysis not only of which tests to perform, but of whether to conduct further testing at all.
The essence of Dynamo is that it applies Bayesian Decision Theory to T&E to maintain and visualize the state of knowledge about a system under test at all times, and that it can make recommendations at any time about which test options to conduct to provide the greatest expected benefit to stakeholders relative to the cost of testing. This talk will discuss the progress to date developing Dynamo and some of the future work remaining to make it more easily adaptable to testing specific systems.
Session Materials: https://dataworks.testscience.org/wp-content/uploads/formidable/23/Thrs_4D_Ferry.pdf
Jamie Thorpe
Cybersecurity R&D, Sandia National Laboratories
Lessons Learned for Study of Uncertainty Quantification in Cyber-Physical System Emulation
Day 3, Room: B 1:00 PM-3:00 PM
Jamie Thorpe is a cybersecurity researcher at Sandia National Laboratories in Albuquerque, NM, where she develops the tools and methodologies needed to help build and analyze models of critical infrastructure systems. Her research interests include cyber resilience metrics, efficient system model development, data analysis for emulated environments, and rigorous cyber experimentation.
Abstract: Lessons Learned for Study of Uncertainty Quantification in Cyber-Physical System Emulation
Solving Program Evaluation Challenges
Over the past decade, the number and severity of cyber-attacks to critical infrastructure has continued to increase, necessitating a deeper understanding of these systems and potential threats. Recent advancements for high-fidelity system modeling, also called emulation, have enabled quantitative cyber experimentation to support analyses of system design, planning decisions, and threat characterization. However, much remains to be done to establish scientific methodologies for performing these cyber analyses more rigorously.
Without a rigorous approach to cyber experimentation, it is difficult for analysts to fully characterize their confidence in the results of an experiment, degrading the ability to make decisions based upon analysis results, and often defeating the purpose of performing the analysis. This issue is particularly salient when analyzing critical infrastructures or similarly impactful systems, where confident, well-informed decision making is imperative. Thus, the integration of tools for rigorous scientific analysis with platforms for emulation-driven experimentation is crucial.
This work discusses one such effort to integrate the tools necessary to perform uncertainty quantification (UQ) on an emulated model, motivated by a study on a notional critical infrastructure use case. The goal of the study was to determine how variations in the aggressiveness of the given threat affected how resilient the system was to the attacker. Resilience was measured using a series of metrics which were designed to capture the system’s ability to perform its mission in the presence of the attack. One reason for the selection of this use case was that the threat and system models were believed to be fairly deterministic and well-understood. The expectation was that results would show a linear correlation between the aggressiveness of the attacker and the resilience of the system. Surprisingly, this hypothesis was not supported by the data.
The initial results showed no correlation, and they were deemed inconclusive. These findings spurred a series of mini analyses, leading to extensive evaluation of the data, methodology, and model to identify the cause of these results. Significant quantities of data collected as part of the initial UQ study enabled closer inspection of data sources and metrics calculation. In addition, tools developed during this work facilitated supplemental statistical analyses, including a noise study. These studies all supported the conclusion that the system model and threat model chosen were far less deterministic than initially assumed, highlighting key lessons learned for approaching similar analyses in the future.
Although this work is discussed in the context of a specific use case, the authors believe that the lessons learned are generally applicable to similar studies applying statistical testing to complex, high-fidelity system models. Insights include the importance of deeply understanding potential sources of stochasticity in a model, planning how to handle or otherwise account for such stochasticity, and performing multiple experiments and looking at multiple metrics to gain a more holistic understanding of a modeled scenario. These results highlight the criticality of approaching system experimentation with a rigorous scientific mindset.

Jasmine Ratchford
Research Scientist, CMU Software Engineering Institute
Experimental Design for Usability Testing of LLMs
Day 3, Room: A 1:00 PM-3:00 PM
Jasmine Ratchford is a Machine Learning Research Scientist and the CMU Software Engineering Institute (SEI). Dr. Ratchford received her Ph.D. in Physics from the University of Texas at Austin and has spent 15 years working across the federal government, supporting efforts at DARPA, DHS, and DOT&E. At SEI, Dr. Ratchford focuses on AI research & engineering practices in areas such as large language model development and scientific machine learning.
Abstract: Experimental Design for Usability Testing of LLMs
Improving the Quality of Test & Evaluation
Large language models (LLMs) are poised to dramatically impact the process of composing, analyzing, and editing documents, including within DoD and IC communities. However, there have been few studies that focus on understanding human interactions and perceptions of LLM outputs, and even fewer still when one considers only those relevant to a government context. Furthermore, there is a paucity of benchmark datasets and standardized data collection schemes necessary for assessing the usability of LLMs in complex tasks, such as summarization, across different organizations and mission use cases. Such usability studies require an understanding beyond the literal content of the document; the needs and interests of the reader must be considered, necessitating an intimate understanding of the operational context. Thus, adequately measuring the effectiveness and suitability of LLMs requires usability testing to be incorporated into the testing and evaluation process.
However, measures of usability are stymied by three challenges. First, there is an unsatisfied need for mission-relevant data that can be used for assessment, a critical first step. Agencies must provide data for assessment of LLM usage, such as report summarization, to best evaluate the effectiveness of LLMs. Current widely available datasets for assessing LLMs consist primarily of ad hoc exams ranging from the LSAT to sommelier exams. High performance on these exams offers little insight into LLM performance on mission tasks, which possess a unique lexicon, set of high-stakes mission applications, and DoD and IC userbase. Notably, our prior work indicates that currently available curated datasets are unsuitable proxies for government reporting. Our search for proxy data for intelligence reports led us on a path to create our own dataset in order to evaluate LLMs within mission contexts.
Second, a range of experimental design techniques exists for collecting human-centric measures of LLM usability, each with their own benefits and disadvantages. Navigating the tradeoffs between these different techniques is challenging, and the lack of standardization across different groups inhibits comparison between groups. A discussion is provided on the potential usage of commonly conducted usability studies, including heuristic evaluations, observational and user experience studies, and tool instrumentation focusing on LLMs used in summarization. We will describe the pros and cons of each study, crafting guidance against their approximate required resources in terms of time (planning, participant recruitment, study, and analysis), compute, and data. We will demonstrate how our data collection prototype for summarization tasks can be used to streamline the above.
The final challenge involves associating human-centric measures, such as ratings of fluency, to other more quantitative and mission-level metrics. We will provide an overview of measures for summarization quality, including ratings for accuracy, concision, fluency, and completeness, and discuss current efforts and existing challenges in associating those measures to quantitative and qualitative metrics. We will also discuss the value of such efforts in building a more comprehensive assessment of LLMs, as well as the relevance of these efforts to document summarization.
Session Materials: https://dataworks.testscience.org/wp-content/uploads/formidable/23/Thrs_4C_Ratchford.pptx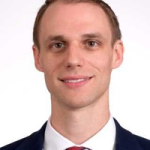
Jason Schlup, Jared Aguayo, and Misael Valentin
Silence of the Logs: A Cyber Red Team Data Collection Framework
Day 2, Room: B 10:30 AM-12:30 PM
Dr. Jason Schlup received his Ph.D. in Aeronautics from the California Institute of Technology in 2018. He is now a Research Staff Member at the Institute for Defense Analyses and provides analytical support to the Director, Operational Test and Evaluation’s Cyber Assessment Program. His research and interest areas include enterprise network attack analysis, improving data collection methodologies, and cloud security. Jason also contributes to IDA’s Cyber Lab capability, focusing on Internet Protocol-based training modules and outreach opportunities.
Jared Aguayo, a professional in computer science and software engineering at Johns Hopkins APL, holds a Bachelor's in Computer Science and a Master's in Software Engineering from the University of Texas at El Paso, where he was an SFS scholar. Specializing in 5G and SDN research during his master's, he honed his development and teamwork skills through capstone projects with the Army and Pacific Northwest National Lab. At APL, Jared applies these skills to significant projects, leveraging his willingness to learn and experience.
Misael Valentin is a software engineer with the Resilient Military Systems group at Johns Hopkins University Applied Physics Laboratory. He graduated with a BS in Computer Engineering with a focus on embedded systems from the University of Puerto Rico, and later obtained an MS in Computer Science with a focus on machine learning from Johns Hopkins University. As part of his work at APL, Misael develops software that helps to enable the creation of cyber-resilient systems in support of multiple sponsors spanning multiple domains, from Virginia Class submarines, to national security space systems, and nuclear command, control, and communications. He is also the APL representative to the DOT&E AI Working Group.
Abstract: Silence of the Logs: A Cyber Red Team Data Collection Framework
Sharing Analysis Tools, Methods, and Collaboration Strategies
Capturing the activities of Cyber Red Team operators as they conduct their mission in a way that is both reproducible and also granular enough for detailed analysis poses a challenge to test organizations for cyber testing. Cyber Red Team members act as both operators and data collectors, all while keeping a busy testing schedule and working within a limited testing window. Data collection often suffers at the expense of meeting testing objectives. Data collection assistance may therefore be beneficial to support Cyber Red Team members so they can conduct cyber operations while still delivering the needed data.
To assist in data collection, DOT&E, IDA, Johns Hopkins University Applied Physics Lab, and MITRE are developing a framework, including a data standard that supports data collection requirements, for Cyber Red Teams called Silence of the Logs (SotL). The goal of delivering SotL is to have Red Teams continue operations as normal while automatically logging activity in the SotL data standard and generating data needed for analyses. In addition to the data standard and application framework, the SotL development team has created example capabilities that record logs from a commonly used commercial Red Team tool in the data standard format. As Cyber Red Teams adopt other Red Team tools, they can use the SotL data standard and framework to create their own logging mechanisms to meet data collection requirements. Analysts also benefit from the SotL data standard as it enables reproducible data analysis. This talk demonstrates current SotL capabilities and presents possible data analysis techniques enabled by SotL.
Session Materials: https://dataworks.testscience.org/wp-content/uploads/formidable/23/Wed_1B_Schlup.pptx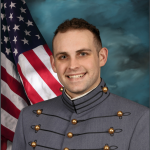
Jason Ingersoll
Cadet, West Point
Tactical Route Optimization: A Data Driven Method for Military Route Planning
Day 2, Room: Cafe 5:00 PM-7:00 PM
Jason Ingersoll is an operations research major from the United States Military Academy, with a focus on the innovative applications of mathematics and computer science. They began their research journey by utilizing Markov Chains to predict NBA game scores, later incorporating Monte Carlo simulations. Their internships at Lockheed Martin and MIT Lincoln Labs further allowed them to work on radar optimization and design a short-range communication system using infrared LEDs. Additionally, Jason Ingersoll has presented research on the ethical use of brain-computer interfaces to address PTSD in soldiers at an Oxford conference and is published in the American Intelligence Journal. During their senior year, they have worked on a military route planning program, integrating A-Star algorithms, GPS, and LIDAR data, as part of their capstone project. Their graduate research plan includes pursuing a degree in Artificial Intelligence and Machine Learning at an institution like MIT, aiming to advance AI's ethical integration within the military. With a passion for bridging technological innovation and ethical responsibility, Jason Ingersoll is dedicated to enhancing the military's decision-making capabilities and software through the responsible application of AI.
Abstract: Tactical Route Optimization: A Data Driven Method for Military Route Planning
Advancing Test & Evaluation of Emerging and Prevalent Technologies
Military planners frequently face the challenging task of devising a route plan based solely on a map and a grid coordinate of their objective. This traditional approach is not only time-consuming but also mentally taxing. Moreover, it often compels planners to make broad assumptions, resulting in a route that is based more on educated guesses than on data-driven analysis. To address these limitations, this research explores the potential of utilizing a path-finding algorithm to assist planners such as A*. Specifically, our algorithm aims to identify the route that minimizes the likelihood of enemy detection, thereby providing a more optimized and data-driven path for mission success. We have developed a model that takes satellite imagery data and produces a feasible route that minimizes detection given the location of an enemy. Future work includes improving the graphical interface and the development of k-distinct paths to provide planners with multiple options.
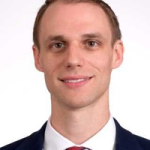
Jason Schlup
Research Staff Member, IDA
Operationally Representative Data and Cybersecurity for Avionics Demonstration
Day 2, Room: Cafe 5:00 PM-7:00 PM
Dr. Jason Schlup received his Ph.D. in Aeronautics from the California Institute of Technology in 2018. He is now a Research Staff Member at the Institute for Defense Analyses and provides analytical support to the Director, Operational Test and Evaluation’s Cyber Assessment Program. His research and interest areas include enterprise network attack analysis, improving data collection methodologies, and cloud security. Jason also contributes to IDA’s Cyber Lab capability, focusing on Internet Protocol-based training modules and outreach opportunities.
Abstract: Operationally Representative Data and Cybersecurity for Avionics Demonstration
Advancing Test & Evaluation of Emerging and Prevalent Technologies
This poster session considers the ARINC 429 standard and its inherent lack of security by using a hardware-in-the-loop (HITL) simulator to demonstrate possible mission effects from a cyber compromise. ARINC 429 is a ubiquitous data bus for civil avionics, enabling safe and reliable communication between devices from disparate manufacturers. However, ARINC 429 lacks any form of encryption or authentication, making it an inherently insecure communication protocol and rendering any connected avionics vulnerable to a range of attacks.
This poster session includes a hands-on demonstration of possible mission effects due to a cyber compromise of the ARINC 429 data bus by putting the audience at the control of the HITL flight simulator with ARINC 429 buses. The HITL simulator uses commercial off-the-shelf avionics hardware including a multi-function display, and an Enhanced Ground Proximity Warning System to generate operationally realistic ARINC 429 messages. Realistic flight controls and flight simulation software are used to further increase the simulator’s fidelity. The cyberattack is based on a system with a malicious device physically-connected to the ARINC 429 bus network. The cyberattack degrades the multi-function display through a denial-of-service attack which disables important navigational aids. The poster also describes how testers can plan to test similar buses found on vehicles and can observe and document data from this type of testing event.
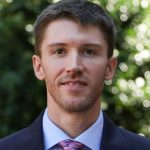
John Dennis
Research Staff Member (Economist), IDA
Data VV&A for AI Enabled Capabilities
Day 3, Room: A 9:50 AM-11:50 AM
John W. Dennis (Jay) earned his PhD in Economics from UNC Chapel Hill. He is a research staff member at the Institute for Defense Analyses, where he is a member of the Human Capital and Test Science groups. He specializes in Econometrics, Statistics, and Data Science.
Abstract: Data VV&A for AI Enabled Capabilities
Advancing Test & Evaluation of Emerging and Prevalent Technologies
Data – collection, preparation, and curation - is a crucial need in the AI lifecycle. Ensuring that the data are consistent, correct, and representative for the intended use is critical to ensuring the efficacy of an AI enabled system. Data verification, validation, and accreditation (VV&A) is meant to address this need. The dramatic increase in the prevalence of AI-enabled capabilities and analytic tools across the DoD has emphasized the need for a unified understanding of data VV&A, as quality data forms the foundation of AI models. In practice, data VV&A and associated activities are often used in an ad-hoc manner that may limit the ability to support development and testing of AI enabled capabilities. However, existing DOD frameworks for data VV&A are applicable to the AI lifecycle and embody important supporting activities for T&E of AI enabled systems. We highlight the importance of data VV&A, relying on established definitions, and outline some concerns and best practices.
Session Materials: https://dataworks.testscience.org/wp-content/uploads/formidable/23/Thrs_4A_Dennis.pptx
Jonathan Rathsam
Senior Research Engineer, NASA Langley Research Center
Overview of a survey methods test for the NASA Quesst community survey campaign
Day 3, Room: C 1:00 PM-3:00 PM
Dr. Jonathan Rathsam is a Senior Research Engineer at NASA’s Langley Research Center in Hampton, Virginia. He conducts laboratory and field research on human perceptions of low noise supersonic overflights. He currently serves as technical lead of survey design and analysis for the X-59 community overflight phase of NASA’s Quesst mission. He also serves as co-chair for Team 6 – Community response to noise and annoyance for the International Commission on Biological Effects of Noise, and previously served as NASA co-chair for DATAWorks. He holds a Ph.D. in Engineering from the University of Nebraska, a B.A. in Physics from Grinnell College in Iowa, and completed postdoctoral research in acoustics at Ben-Gurion University in Israel.
Abstract: Overview of a survey methods test for the NASA Quesst community survey campaign
In its mission to expand knowledge and improve aviation, NASA conducts research to address sonic boom noise, the prime barrier to overland supersonic flight. NASA is currently preparing for a community survey campaign to assess response to noise from the new X-59 aircraft. During each community survey, a substantial number of observations must be collected over a limited timeframe to generate a dose response relationship. A sample of residents will be recruited in advance to fill out a brief survey each time X-59 flies over, approximately 80 times throughout a month. In preparation NASA conducted a month-long test of survey methods in 2023. A sample of 800 residents was recruited from a simulated fly-over area. Because there were no actual X-59 fly-overs, respondents were asked about their reactions to noise from normal aircraft operations. The respondents chose whether to fill out the survey on the web or via a smartphone application. Evaluating response rates and how they evolved over time was a specific focus of the test. Also, a graduated incentive structure was implemented to keep respondents engaged. Finally, location data was collected from respondents since it will be needed to estimate individual noise exposure from X-59. The results of this survey test will help determine the design of the community survey campaign. This is an overview presentation that will cover the key goals, results, and lessons learned from the survey test.
Session Materials: https://dataworks.testscience.org/wp-content/uploads/formidable/23/Thrs_CS3_Rathsam.pptx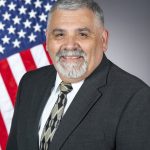
Jose Alvarado
Technical Advisor, AFOTEC Det 5/CTO
Developing Model-Based Flight Test Scenarios
Day 2, Room: C 1:40 PM-3:10 PM
JOSE ALVARADO is a senior test engineer and system analyst for AFOTEC at Edwards AFB, California with over 33 years of developmental and operational test and evaluation experience. He is a Ph.D. candidate in the Systems Engineering doctorate program at Colorado State University with research interests in applying MBSE concepts to the flight test engineering domain and implementing test process improvements through MBT. Jose holds a B.S. in Electrical Engineering from California State University, Fresno (1991), and an M.S. in Electrical Engineering from California State University, Northridge (2002). He serves as an adjunct faculty member for the electrical engineering department at the Antelope Valley Engineering Program (AVEP) overseen by California State University, Long Beach. He is a member of the International Test and Evaluation Association, Antelope Valley Chapter.
Abstract: Developing Model-Based Flight Test Scenarios
The Department of Defense (DoD) is undergoing a digital engineering transformation in every process of the systems engineering lifecycle. This transformation provides the requirement that DoD Test and Evaluation (T&E) processes begin to implement and begin executing model-based testing methodologies. This paper describes and assesses a grey box model-driven test design (MDTD) approach to create flight test scenarios based on model-based systems engineering artifacts. To illustrate the methodology and evaluate the expected outcomes of the process in practice, a case study using a model representation of a training system utilized to train new Air Force Operational Test and Evaluation Center (AFOTEC) members in conducting operational test and evaluation (OT&E) is presented. The results of the grey box MDTD process are a set of activity diagrams that are validated to generate the same test scenario cases as the traditional document-centric approach. Using artifacts represented in System Modeling Language (SysML), this paper will discuss key comparisons between the traditional and MDTD processes. This paper demonstrates the costs and benefits of model-based testing and their relevance in the context of operational flight testing.
Session Materials: https://dataworks.testscience.org/wp-content/uploads/formidable/23/Wed_2C_Alvarado.pptx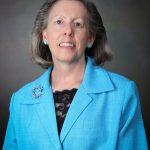
Dr. Judi See
Sandia National Laboratories
Understanding and Applying the Human Readiness Level Scale During User-Centered Design
Day 1, Room: B 9:00 AM-4:00 PM
Dr. Judi See is a systems analyst and human factors engineer at Sandia National Laboratories in Albuquerque, New Mexico. Her work involves leading research and analysis focused on the human component of the nuclear deterrence system. Dr. See has a doctorate degree in human factors, master’s degrees in human factors and systems engineering, and professional certification in human factors and ergonomics through the Board of Certification in Professional Ergonomics. She became a Distinguished Member of the Technical Staff at Sandia National Laboratories in 2021. Her research interests include vigilance, signal detection theory, visual inspection, and human readiness levels.
Abstract: Understanding and Applying the Human Readiness Level Scale During User-Centered Design
The purpose of this short course is to support knowledge and application of the Human Readiness Level (HRL) scale described in ANSI/HFES 400-2021 Human Readiness Level Scale in the System Development Process. The HRL scale is a simple nine-level scale designed to supplement the Technology Readiness Level (TRL) scale to evaluate, track, and communicate the readiness of a technology or system for safe and effective human use. Application of the HRL scale ensures proper attention to human systems design throughout system development, which minimizes or prevents human error and enhances the user experience.
Learning objectives for the short course include:
(1) Understand the relationship between a user-centered design (UCD) process and the HRL Scale. Instructors will discuss a “typical” UCD process describing the design activities and data collected that support HRL Scale evaluation and tracking.
(2) Learn effective application of usability testing in a DOD environment. Instructors will describe iterative, formative usability testing with a hands-on opportunity to perform usability tasks. Human-centered evaluation of system design is a critical activity when evaluating the extent to which a system is ready for human use.
(3) Understand HFES 400-2021 development and contents. Instructors will describe the evolution of the HRL concept to convey its significance and the rigor behind the development of the technical standard. Instructors will walk through major sections of the standard and describe how to apply them.
(4) Learn how the HRL scale is applied in current and historical acquisition programs. Instructors will describe real-world Army applications of the HRL scale, including a case study of a software modernization program.
(5) Apply the HRL scale to practical real-world problems. Attendees will gain hands-on experience applying the HRL scale during group exercises that simulate teamwork during the system development process. Group exercises incorporate three different scenarios, being both hardware and software solutions at various stages of technological development. The hands-on exercises specifically address common questions about the practical use of the HRL scale. Course attendees do not need prior human factors/ergonomics knowledge or ability. The HRL scale is intended to be applied by human systems professionals with proper ability and experience; however, recipients of HRL scale ratings include many other types of personnel in design, engineering, and acquisition as well as high-level decision-makers, all of whom benefit from understanding the HRL scale. Before attending the course, students should download a free copy of the ANSI/HFES technical standard at https://my.hfes.org/online-store/publications and bring it to the course in electronic or hard copy format. Laptops are not necessary for the course but may facilitate notetaking and completion of the group exercises.
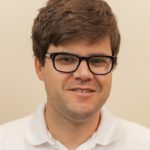
Justin Krometis and Adam Ahmed
Leveraging Bayesian Methods to support Integrated Testing
Day 3, Room: D 9:50 AM-11:50 AM
Justin Krometis is a Research Assistant Professor in the Intelligent Systems Division of the Virginia Tech National Security Institute and an Affiliate Research Assistant Professor in the Virginia Tech Department of Mathematics. His research is in the development of theoretical and computational frameworks for Bayesian inference, particularly in high-dimensional regimes, and in the application of those methods to domain sciences ranging from fluids to geophysics to testing and evaluation. His areas of interest include statistical inverse problems, parameter estimation, machine learning, data science, and experimental design. Dr. Krometis holds a Ph.D. in mathematics, a M.S. in mathematics, a B.S. in mathematics, and a B.S. in physics, all from Virginia Tech.
Adam S. Ahmed is a Research Scientist at Metron, Inc. and is the technical lead for the Metron DOT&E effort. His research interests include applying novel Bayesian approaches to testing and evaluation, machine learning methods for small datasets as applied to undersea mine classification, and time series classification for continuous active sonar systems. Prior to Metron, he worked on the synthesis and measurement of skyrmion-hosting materials for next generation magnetic memory storage devices at The Ohio State University. Dr. Ahmed holds a Ph.D. and M.S. in physics from The Ohio State University, and a B.S. in physics from University of Illinois Urbana-Champaign.
Abstract: Leveraging Bayesian Methods to support Integrated Testing
This mini-tutorial will outline approaches to apply Bayesian methods to the test and evaluation process, from development of tests to interpretation of test results to translating that understanding into decision-making. We will begin by outlining the basic concepts that underlie the Bayesian approach to statistics and the potential benefits of applying that approach to test and evaluation. We will then walk through application to an example (notional) program, setting up data models and priors on the associated parameters, and interpreting the results. From there, techniques for integrating results from multiple stages of tests will be discussed, building understanding of system behavior as evidence accumulates. Finally, we will conclude by describing how Bayesian thinking can be used to translate information from test outcomes into requirements and decision-making. The mini-tutorial will assume some background in statistics but the audience need not have prior exposure to Bayesian methods.
Session Materials: https://dataworks.testscience.org/wp-content/uploads/formidable/23/Thrs_4D_Krometis_Ahmed.zip
Karen O'Brien
Senior Principal Data Scientist, Modern Technology Solutions, Inc
Hypersonic Glide Vehicle Trajectories: A conversation about synthetic data in T&E
Day 3, Room: A 1:00 PM-3:00 PM
Karen O’Brien is a senior principal data scientist and AI/ML practice lead at Modern Technology Solutions, Inc. In this capacity, she leverages her 20-year Army civilian career as a scientist, evaluator, ORSA, and analytics leader to aid DoD agencies in implementing AI/ML and advanced analytics solutions. Her analytics career ranged ‘from ballistics to logistics’ and most of her career was in Army Test and Evaluation Command or supporting Army T&E from the Army Research Laboratory. She was physics and chemistry nerd in her early career, but now uses her M.S. in Predictive Analytics from Northwestern University to help her DoD clients tackle the toughest analytics challenges in support of the nation’s Warfighters.
Abstract: Hypersonic Glide Vehicle Trajectories: A conversation about synthetic data in T&E
Advancing Test & Evaluation of Emerging and Prevalent Technologies
The topic of synthetic data in test and evaluation is steeped in controversy – and rightfully so. Generative AI techniques can be erratic, producing non-credible results that should give evaluators pause. At the same time, there are mission domains that are difficult to test, and these rely on modeling and simulation to generate insights for evaluation. High fidelity modeling and simulation can be slow, computationally intensive, and burdened by large volumes of data – challenges which become prohibitive as test complexity grows.
To mitigate these challenges, we posit a defensible, physically valid generative AI approach to creating fast-running synthetic data for M&S studies of hard-to-test scenarios. Characterized as a “Narrow Digital Twin,” we create an exemplar Generative AI model of high-fidelity Hypersonic Glide Vehicle trajectories. The model produces a set of trajectories that meets user-specified criteria (particularly as directed by a Design of Experiments) and that can be validated against the equations of motion that govern these trajectories. This presentation will identify the characteristics of the model that make it suitable for generating synthetic data and propose easy-to-measure acceptability criteria. We hope to advance a conversation about appropriate and rigorous uses of synthetic data within T&E.
Session Materials: https://dataworks.testscience.org/wp-content/uploads/formidable/23/Thrs_CS1_OBrien_For-website.pdf
Karen Alves da Mata
Graduate Research Assistant, University of Massachusetts Dartmouth
Quantitative Reliability and Resilience Assessment of a Machine Learning Algorithm
Day 2, Room: B 3:30 PM-5:00 PM
Karen da Mata is a Ph.D. student in the Electrical and Computer Engineering Department at the University of Massachusetts - Dartmouth. She received her MS in Computer Engineering from UMassD in 2023 and her BS degree in the Electrical Engineering Department at the Federal University of Ouro Preto - Brazil - in 2018.
Abstract: Quantitative Reliability and Resilience Assessment of a Machine Learning Algorithm
Advancing Test & Evaluation of Emerging and Prevalent Technologies
Advances in machine learning (ML) have led to applications in safety-critical domains, including security, defense, and healthcare. These ML models are confronted with dynamically changing and actively hostile conditions characteristic of real-world applications, requiring systems incorporating ML to be reliable and resilient. Many studies propose techniques to improve the robustness of ML algorithms. However, fewer consider quantitative methods to assess the reliability and resilience of these systems. To address this gap, this study demonstrates how to collect relevant data during the training and testing of ML suitable for applying software reliability, with and without covariates, and resilience models, and the subsequent interpretation of these analyses. The proposed approach promotes quantitative risk assessment of machine learning technologies, providing the ability to track and predict degradation and improvement in the ML model performance and assisting ML and system engineers with an objective approach to compare the relative effectiveness of alternative training and testing methods. The approach is illustrated in the context of an image recognition model subjected to two generative adversarial attacks and then iteratively retrained to improve the system's performance. Our results indicate that software reliability models incorporating covariates characterized the misclassification discovery process more accurately than models without covariates. Moreover, the resilience model based on multiple linear regression incorporating interactions between covariates tracked and predicted degradation and recovery of performance best. Thus, software reliability and resilience models offer rigorous quantitative assurance methods for ML-enabled systems and processes.
Session Materials: https://dataworks.testscience.org/wp-content/uploads/formidable/23/Wed_SS_Da-Mata.pdf
Dr. Karl Pazdernik
Pacific Northwest National Laboratory
Text Analysis: Introduction to Advanced Language Modeling
Day 1, Room: D 9:00 AM-4:00 PM
Dr. Karl Pazdernik is a senior data scientist at Pacific Northwest National Laboratory. He is also a research assistant professor at North Carolina State University (NCSU) and the former chair of the American Statistical Association Section on Statistics in Defense and National Security. His research has focused on the dynamic modeling of multi-modal data with a particular interest in text analytics, spatial statistics, pattern recognition, anomaly detection, Bayesian statistics, and computer vision. Recent projects include natural language processing of multilingual unstructured financial data, anomaly detection in combined open-source data streams, automated biosurveillance and disease forecasting, and deep learning for defect detection and element mass quantification in nuclear materials. He received a Ph.D. in Statistics from Iowa State University and was a postdoctoral scholar at NCSU under the Consortium for Nonproliferation Enabling Capabilities.
Abstract: Text Analysis: Introduction to Advanced Language Modeling
This course will provide a broad overview of text analysis and natural language processing (NLP), including a significant amount of introductory material with extensions to state-of-the-art methods. All aspects of the text analysis pipeline will be covered including data preprocessing, converting text to numeric representations (from simple aggregation methods to more complex embeddings), and training supervised and unsupervised learning methods for standard text-based tasks such as named entity recognition (NER), sentiment analysis, topic modeling, and text generation using Large Language Models (LLMs). The course will alternate between presentations and hands-on exercises in Python. Translations from Python to R will be provided for students more comfortable with that language. Attendees should be familiar with Python (preferably), R, or both and have a basic understanding of statistics and/or machine learning. Attendees will gain the practical skills necessary to begin using text analysis tools for their tasks, an understanding of the strengths and weaknesses of these tools, and an appreciation for the ethical considerations of using these tools in practice.
Session Materials: https://dataworks.testscience.org/wp-content/uploads/formidable/23/Text_Analysis_DATAWorks_2024.pdf
Karly Parcell
Cadet, United States Military Academy
Object Identification and Classification in Threat Scenarios
Day 2, Room: Cafe 5:00 PM-7:00 PM
My name is Karly Parcell, I am a class of 2024 Cadet, majoring in Applied Statistics and Data Science at the United States Military Academy. My collaborators for this paper are COL James Starling and Dr. Brian Choi.
Abstract: Object Identification and Classification in Threat Scenarios
Sharing Analysis Tools, Methods, and Collaboration Strategies
In rapidly evolving threat scenarios, the accurate and timely identification of hostile enemies armed with weapons is crucial to strategic advantage and personnel safety. This study aims to develop both a timely and accurate model utilizing YOLOv5 for the detection of weapons and persons in real-time drone footage and generate an alert containing the count of weapons and persons detected. Existing methods in this field often focus on either minimizing type I/type II errors or the speed at which the model runs. In our current work, we have focused on two main points of emphasis throughout training our model. The minimization of type II error (minimizing instances of weapons of persons present but not detected) and keeping accuracy and precision consistent while increasing the speed of our model to keep up with real-time footage. Various parameters were adjusted within our model, including but not limited to speed, freezing layers, and image size. Going from our first to the final adjusted model, overall precision and recall went from 71.9% to 89.2% and 63.7% to 77.5%, respectively. The occurrences of misidentification produced from our model decreased dramatically, from 27% of persons misidentified as either weapon or background noise to 14%, and the misidentification of weapons from 50% to 34%. An important consideration for future work is mitigating overfitting the model to a particular dataset when training. In real-world implementation, our model needs to perform well across a variety of conditions and angles not all of which were introduced in the training data set.

Kate Maffey and Robert Edman
MLTEing Models: Negotiating, Evaluating, and Documenting Model and System Qualities
Day 3, Room: A 9:50 AM-11:50 AM
CPT Kate Maffey is a Data Scientist at the U.S. Army's Artificial Intelligence Integration Center (AI2C), where she specializes in applied machine learning evaluation research.
Abstract: MLTEing Models: Negotiating, Evaluating, and Documenting Model and System Qualities
Many organizations seek to ensure that machine learning (ML) and artificial intelligence (AI) systems work as intended in production but currently do not have a cohesive methodology in place to do so. To fill this gap, we built MLTE (Machine Learning Test and Evaluation, colloquially referred to as “melt”), a framework and implementation to evaluate ML models and systems. The framework compiles state-of-the-art evaluation techniques into an organizational process for interdisciplinary teams, including model developers, software engineers, system owners, and other stakeholders. MLTE tooling, a Python package, supports this process by providing a domain-specific language that teams can use to express model requirements, an infrastructure to define, generate, and collect ML evaluation metrics, and the means to communicate results.
In this presentation, we will discuss current MLTE details as well as future plans to support developmental testing (DT) and operational testing (OT) organizations and teams. A problem in the Department of Defense (DoD) is that test and evaluation (T&E) organizations are segregated: OT organizations work independently from DT organizations, which leads to inefficiencies. Model developers doing contractor testing (CT) may not have access to mission and system requirements and therefore fail to adequately address the real-world operational environment. Motivation to solve these two problems has generated a push for Integrated T&E — or T&E as a Continuum — in which testing is iteratively updated and refined based on previous test outcomes, and is informed by mission and system requirements. MLTE helps teams to better negotiate, evaluate, and document ML model and system qualities, and will aid in the facilitation of this iterative testing approach. As MLTE matures, it can be extended to further support Integrated T&E by (1) providing test data and artifacts that OT can use as evidence to make risk-based assessments regarding the appropriate level of OT and (2) ensuring that CT and DT testing of ML models accurately reflects the challenges and constraints of real-world operational environments.
Session Materials: https://dataworks.testscience.org/wp-content/uploads/formidable/23/Thrs_4A_Maffey_Edman.pptx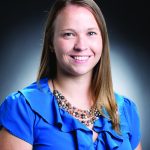
Kelly Koser
Senior Project Manager & Statistician, Johns Hopkins University Applied Physics Laboratory
Adaptive Sequential Experimental Design for Strategic Reentry Simulated Environment
Day 2, Room: A 3:30 PM-5:00 PM
Kelly Koser currently serves as a senior project manager and statistician at the Johns Hopkins University Applied Physics Laboratory (JHU/APL). She has 15 years of experience conducting system test & evaluation (T&E), experimental design, statistical analysis and modeling, program evaluation, and strategic program development activities. Ms. Koser currently supports a variety of U.S. Navy and U.S. Air Force weapon system evaluation activities and reliability assessments. She also leads the investigation and T&E of promising screening technologies to ensure public spaces protection for the Transportation Security Administration (TSA). Ms. Koser holds a Bachelor of Science in Mathematical Sciences from Carnegie Mellon University, a Master of Science in Applied and Computational Mathematics from Johns Hopkins University, and a graduate certificate in Engineering Management from Drexel University.
Abstract: Adaptive Sequential Experimental Design for Strategic Reentry Simulated Environment
Improving the Quality of Test & Evaluation
To enable the rapid design and evaluation of survivable reentry systems, the Johns Hopkins University Applied Physics Laboratory (JHU/APL) developed a simulation environment to quickly explore the reentry system tradespace. As part of that effort, a repeatable process for designing and assessing the tradespace was implemented utilizing experimental design and statistical modeling techniques. This talk will discuss the utilization of the fast flexible filling experimental design and maximum value-weighted squared error (MaxVSE) adaptive sequential experimental design methods and Gaussian Process modeling techniques for assessing features that impact reentry system trajectories and enabling continuous model refinements. The repeatable scripts used to implement these methods allow for integration into other software tools for a complete end-to-end simulation of reentry systems.
Session Materials: https://dataworks.testscience.org/wp-content/uploads/formidable/23/Wed_3A_Koser.pptx
Keltin Grimes
Assistant Machine Learning Research Scientist, Software Engineering Institute
Statistical Validation of Fuel Savings from In-Flight Data Recordings
Day 3, Room: C 1:00 PM-3:00 PM
Keltin joined the Software Engineering Institute's AI Division in June of 2023 as an Assistant Machine Learning Research Scientist after graduating from Carnegie Mellon University with a B.S. in Statistics and Machine Learning and an additional major in Computer Science. His previous research projects have included work on Machine Unlearning, adversarial attacks on ML systems, and ML for materials discovery.
Abstract: Statistical Validation of Fuel Savings from In-Flight Data Recordings
Solving Program Evaluation Challenges
The efficient use of energy is a critical challenge for any organization, but especially in aviation, where entities such as the United States Air Force operate on a global scale, using many millions of gallons of fuel per year and requiring a massive logistical network to maintain operational readiness. Even very small modifications to aircraft, whether it be physical, digital, or operational, can accumulate substantial changes in a fleet’s fuel consumption. We have developed a prototype system to quantify changes in fuel use due to the application of an intervention, with the purpose of informing decision-makers and promoting fuel-efficient practices. Given a set of in-flight sensor data from a certain type of aircraft and a list of sorties for which an intervention is present, we use statistical models of fuel consumption to provide confidence intervals for the true fuel efficiency improvements of the intervention. Our analysis shows that, for some aircraft, we can reliably detect the presence of interventions with as little as a 1% fuel rate improvement and only a few hundred sorties, enabling rapid mitigation of even relatively minor issues.
Session Materials: https://dataworks.testscience.org/wp-content/uploads/formidable/23/Thrs_CS3_Grimes.pptx
Kyle Risher
Undergraduate Research Intern, Virginia Tech National Security Institute
Automated Tools for Improved Accessibility of Bayesian Analysis Methods
Day 2, Room: Cafe 5:00 PM-7:00 PM
Kyle Risher is an undergraduate research intern at the Virginia Tech National Security Institute. His research has been focused on the creation of tools for automating defense system reliability analysis. He is currently a Senior pursuing a B.S. in Statistics from Virginia Tech. After graduation, he will be taking on a Naval Warfighting Analyst role at the Naval Surface Warfare Center in Carderock, Maryland.
Abstract: Automated Tools for Improved Accessibility of Bayesian Analysis Methods
Sharing Analysis Tools, Methods, and Collaboration Strategies
Statistical analysis is integral to the evaluation of defense systems throughout the acquisition process. Unlike traditional frequentist statistical methods, newer Bayesian statistical analysis incorporates prior information, such as historical data and expert knowledge, into the analysis for a more integrated approach to test data analysis. With Bayesian techniques, practitioners can more easily decide what data to leverage in their analysis, and how much that data should impact their analysis results. This provides a more flexible, informed framework for decision making in the testing and evaluation of DoD systems.
However, the application of Bayesian statistical analyses is often challenging due to the advanced statistical knowledge and technical coding experience necessary for the utilization of current Bayesian programming tools. The development of automated analysis tools can help address these barriers and make modern Bayesian analysis techniques available to a wide range of stakeholders, regardless of technical background. By making new methods more readily-available, collaboration and decision-making are made easier and more effective within the T&E community.
To facilitate this, we have developed a web application with the R Shiny package in R. This application uses an intuitive user interface to enable the implementation of our Bayesian reliability analysis approach by non-technical users without the need for any coding knowledge or advanced statistical background. Users can upload reliability data from the developmental testing and operational testing stages of a system of interest and tweak parameters of their choosing to automatically generate plots and estimates of system reliability performance based on their uploaded data and prior knowledge of system behavior.

Leo Blanken and Jason Lepore
Rethinking Defense Planning: Are We Buying Weapons and Forces? Or Security?
Day 3, Room: D 1:00 PM-3:00 PM
Coauthored:
Leo Blanken, Associate Professor, Defense Analysis Department at the Naval Postgraduate School and Irregular Warfare Initiative Fellow (West Point Modern War Institute).
Jason Lepore, Professor and Chair of the Economics Department at the Orfalea College of Business, CalPoly, San Luis Obispo and a Visiting Professor at the Defense Analysis Department, Naval Postgraduate School.
Abstract: Rethinking Defense Planning: Are We Buying Weapons and Forces? Or Security?
Sharing Analysis Tools, Methods, and Collaboration Strategies
We propose a framework to enable an updated approach for modeling national security planning decisions. The basis of our approach is to treat national security as the multi-stage production of a service provided by the state to foster a nation’s welfare. The challenge in analyzing this activity stems from the fact that this a complex process that is conducted by a vast number of actors across four discrete stages of production: budgeting, planning, coercion, warfighting. We argue that decisions made at any given stage of the process that fail to consider actor incentives at all stages of the process may create serious problems. In this presentation we will present our general Feasible Production Framework approach (a formal framework based on Principal-Agent analysis), paying particular attention to the planning stage of production for this audience. This presentation will highlight the trade-offs in modeling within a narrow “single-stage aperture” versus a holistic “multi-stage aperture.”
Session Materials: https://dataworks.testscience.org/wp-content/uploads/formidable/23/Thrs_MT5_Blanken_Lepore.pptx
Logan Ausman
Research Staff Member, IDA
A Framework for OT&E of Rapidly Changing Software Systems: C3I and Business Systems
Day 2, Room: A 10:30 AM-12:30 PM
Logan Ausman is a Research Staff Member at the Institute for Defense Analyses. He has worked on IDA operational evaluation division's project on operational test and evaluation of Joint C3 systems since 2013. His current work also includes supporting projects on operational test and evaluation of major automated information systems, and on test and assurance of artificial intelligence capabilities. Logan earned a PhD in Chemistry from Northwestern University in 2010, with his research focusing on the theory and computational modeling of enhancements of electromagnetic scattering caused by small particles. He earned a BS in Chemistry from the University of Wisconsin-Eau Claire in 2004.
Abstract: A Framework for OT&E of Rapidly Changing Software Systems: C3I and Business Systems
Advancing Test & Evaluation of Emerging and Prevalent Technologies
Operational test and evaluation (OT&E) of a system provides the opportunity to examine how representative individuals and units use the system to accomplish their missions, and complements functionality-focused automated testing conducted throughout development. Operational evaluations of software acquisitions need to consider more than just the software itself; they must account for the complex interactions between the software, the end users, and supporting personnel (such as maintainers, help desk staff, and cyber defenders) to support the decision-maker who uses information processed through the software system. We present a framework for meeting OT&E objectives while enabling the delivery schedule for software acquisitions by identifying potential areas for OT&E efficiencies. The framework includes continuous involvement beginning in the early stages of the acquisition program to prepare a test strategy and infrastructure for the envisioned pace of activity during the develop and deploy cycles of the acquisition program. Key early OT&E activities are to acquire, develop, and accredit test infrastructure and tools for OT&E, and embed the OT&E workforce in software acquisition program activities. Early OT&E community involvement in requirements development and program planning supports procedural efficiencies. It further allows the OT&E community to determine whether the requirements address the collective use of the system and include all potential user roles. OT&E during capability development and deployment concentrates on operational testing efficiencies via appropriately scoped, dedicated tests while integrating information from all sources to provide usable data that meets stakeholder needs and informs decisions. The testing aligns with deliveries starting with the initial capability release and continuing with risk-informed approaches for subsequent software deployments.
Session Materials: https://dataworks.testscience.org/wp-content/uploads/formidable/23/Wed_1A_Ausman.pptx
Lucas Villanti
Cadet, US Military Academy
Analyzing Factors for Starting Pitcher Pull Decision with Survival Analysis
Day 2, Room: Cafe 5:00 PM-7:00 PM
Cadet Lucas Villanti is an aspiring undergraduate at the United States Military Academy, West Point, majoring in Applied Statistics and Data Science. With a focus on R, Python, and machine learning, Lucas has specialized in Sabremetrics, analyzing baseball statistics, particularly in starting pitchers, over the last three years.
As a leader in both the Finance Club and Ski Club, he brings analytical skills and enthusiasm to these groups. Additionally, Lucas has broadened his global perspective through a project in Morocco, where he aided in restoring a soccer field while immersing himself in the local culture. His academic prowess, leadership, and international experiences mark him as a well-rounded and impactful individual.
Abstract: Analyzing Factors for Starting Pitcher Pull Decision with Survival Analysis
Background: Current practice on how starting pitchers are pulled vary, are inconsistent, and not transparent. With the new major league baseball (MLB) data available through Statcast, such decisions can be more consistently supported by combining measured data.
Methods: To address this gap, we scraped pitch-level data from Statcast, a new technology system that collects real time MLB game measurements using laser technology. Here, we used Statcast data within a Cox regression for survival analysis to identify measurable factors that are associated to pitcher longevity. Measurements from 696,743 pitches were extracted for analysis from the 2021 MLB season. The pitcher was considered “surviving” the pitch if they remained in the game. Mortality was defined as the pitcher’s last pitch. Analysis began at the second inning to account for high variation during the first inning.
Results: Statistically significant factors include HSR(Hits to Strike Ratio), Runs per batter faced, and total bases per batter faced yielded the highest hazard coefficients (ranging from 10-23), which means higher risk of being relieved.
Conclusions: Our findings indicate that HSR, runs per batter faced and total bases per batter faced provide decision making information for relieving the starting pitcher.

Mason Zoellner
Undergraduate Research Assistant, Hume Center for National Security and Technology
Maritime Automatic Target Recognition
Day 2, Room: Cafe 5:00 PM-7:00 PM
I am currently serving as an Undergraduate Research Assistant at the Hume Center for National Security and Technology at Virginia Tech. As a computer science major, I excel in analytical skills, software development, and critical thinking. My current research involves contributing to a Digital Transformation Artificial Intelligence/Machine Learning project, where I apply machine learning and computer vision to automatic target recognition.
Abstract: Maritime Automatic Target Recognition
Advancing Test & Evaluation of Emerging and Prevalent Technologies
The goal of this project was to develop an algorithm that automatically detects and predicts the future position of boats in a maritime environment. We integrated these algorithms into an intelligent combat system developed by the Naval Surface Warfare Center Dahlgren Division (NSWCDD). This algorithm used YOLOv8 for computer vision detection and a linear Kalman filter for prediction. The data used underwent extensive augmentation and third party integration. It was tested at a Live, Virtual, and Constructive (LVC) event held at NSWCDD this past fall (October 2023).
The initial models faced challenges of overfitting. However, through processes such as data augmentation, incorporation of third-party data, and layer freezing techniques, we were able to develop a more robust model. Various datasets were processed by tools to improve data robustness. By further labeling the data, we were able to obtain ground truth data to evaluate the Kalman filter. The Kalman filter was chosen for its versatility and predictive tracking capabilities. Qualitative and quantitative analysis were performed for both the YOLO and Kalman filter models.
Much of the project's contribution lay in its ability to adapt to a variety of data. YOLO displayed effectiveness across various maritime scenarios, and the Kalman Filter excelled in predicting boat movements across difficult situations such as abrupt camera movements.
In preparation for the live fire test event, our algorithm was integrated into the NSWCDD system and code was written to produce the expected output files.
In summary, this project successfully developed an algorithm for detecting and predicting boats in a maritime environment. This project demonstrated the potential of the intersection of machine learning, rapid integration of technology, and maritime security.
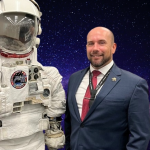
Matthew Wilkerson
Undergraduate Researcher, Intelligent Systems Laboratory, Fayetteville State University
Advancing Edge AI: Benchmarking ResNet50 for Image Classification on Diverse Hardware Platforms
Day 2, Room: Cafe 5:00 PM-7:00 PM
Matthew Wilkerson is an undergraduate student at Fayetteville State University, majoring in computer science. He is an undergraduate researcher at the university’s Intelligent Systems Laboratory, where he is assigned to two NASA-funded research projects involving deep neural networks.
Prior to attending Fayetteville State University, Matthew served in the U.S. Army for over 22 years. He had various assignments, to include: Paralegal NCO, 1st Battalion, 75th Ranger Regiment, Hunter Army Airfield, Georgia (three deployments in support of Operation Iraqi Freedom); Paralegal NCO, 1st Battalion, 5th Special Forces Group (Airborne), Fort Campbell, Kentucky (three deployments in support of Operation Iraq Freedom); Instructor/Writer, 27D Advanced Individual Training, Fort Jackson, South Carolina; Senior Instructor/Writer and later Course Director, 27D Advanced Individual Training, Fort Lee, Virginia; Senior Paralegal NCO, 2nd Infantry Brigade Combat Team, 3rd Infantry Division, Fort Stewart, Georgia; Senior 27D Talent Management NCO, Personnel, Plans, and Training Office, Office of the Judge Advocate General, Pentagon, Washington, DC; Student, US Army Sergeants Major Academy, Fort Bliss, Texas; and Command Paralegal NCO, 82nd Airborne Division, Fort Liberty, North Carolina.
Matthew’s most notable awards and decorations include the Bronze Star Medal, Legion of Merit Medal, Meritorious Service Medal (w/ 2 oak leaf clusters), Valorous Unit Award, Iraqi Campaign Medal (w/ 6 campaign stars), Global War on Terrorism Expeditionary medal, Ranger Tab, Basic Parachutist Badge, and Pathfinder Badge.
Abstract: Advancing Edge AI: Benchmarking ResNet50 for Image Classification on Diverse Hardware Platforms
Advancing Test & Evaluation of Emerging and Prevalent Technologies
The ability to run AI at the edge can be transformative for applications that need to process data to make decisions at the location where sensing and data acquisition takes place. Deep neural networks (DNNs) have a huge number of parameters and consist of many layers, including nodes and edges that contain mathematical relationships that need to be computed when the DNN is run during deployment. This is why it is important to benchmark DNNs on edge computers which are constrained in hardware resources and usually run on a limited supply of battery power. The objective of our NASA funded project which is aligned to the mission of robotic space exploration is to enable AI through fine-tuning of convolutional neural networks (CNNs) for extraterrestrial terrain analysis. This research currently focuses on the optimization of the ResNet50 model, which consists of 4.09 GFLOPs and 25.557 million parameters, to set performance baselines on various edge devices using the Mars Science Laboratory (MSL) v2.1 dataset. Although our initial focus is on Martian terrain classification, the research is potentially impactful for other sectors where efficient edge computing is critical.
We addressed a critical imbalance in the dataset by augmenting the underrepresented class of with an additional 167 images, improving the model's classification accuracy substantially. Pre-augmentation, these images were frequently misclassified as another class, as indicated by our confusion matrix analysis. Post-augmentation, the fine-tuned ResNet50 model achieved an exceptional test accuracy of 99.31% with a test loss of 0.0227, setting a new benchmark for similar tasks.
The core objective of this project extends beyond classification accuracy; it aims to establish a robust development environment for testing efficient edge AI models suitable for deployment in resource-constrained scenarios. The fine-tuned ResNet50-MSL-v2.1 model serves as a baseline for this development. The model was converted into a TorchScript format to facilitate cross-platform deployment and inference consistency.
Our comprehensive cross-platform evaluation included four distinct hardware configurations, chosen to mirror a variety of deployment scenarios. The NVIDIA Jetson Nano achieved an average inference time of 62.04 milliseconds with 85.83% CPU usage, highlighting its utility in mobile contexts. An Intel NUC with a Celeron processor, adapted for drone-based deployment, registered an inference time of 579.87 milliseconds at near-maximal CPU usage of 99.77%. A standard PC equipped with an RTX 3060 GPU completed inference in just 6.52 milliseconds, showcasing its capability for high-performance, stationary tasks. Lastly, an AMD FX 8350 CPU-only system demonstrated a reasonable inference time of 215.17 milliseconds, suggesting its appropriateness for less demanding edge computing applications.
These results not only showcase the adaptability of ResNet50 across diverse computational environments but also emphasize the importance of considering both model complexity and hardware capabilities when deploying AI at the edge. Our findings indicate that with careful optimization and platform-specific tuning, it is possible to deploy advanced AI models like ResNet50 effectively on a range of hardware, from low-power edge devices to high-performance ground stations. Our ongoing research will use these established baselines to further explore efficient AI model deployment in resource-constrained setting.
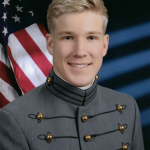
Max Felter
CDT, USCC West Point
Synthetic Data for Target Acquisition
Day 2, Room: Cafe 5:00 PM-7:00 PM
My name is Max Felter and I am currently a sophomore at the United States Military Academy at West Point. I am an applied statistics and data science major in the honors track. I plan to stay involved with research as a cadet and hope to pursue a graduate degree sometime thereafter. In my first year conducting research, I have focused on the topic of computer vision utilizing current off-the-shelf models. I am passionate about the intersection of innovation and service and hope to contribute throughout my career.
Abstract: Synthetic Data for Target Acquisition
Advancing Test & Evaluation of Emerging and Prevalent Technologies
As the battlefield undergoes constant evolution, and we anticipate future conflicts, there is a growing need for apt computer vision models tailored toward military applications. The heightened use of drones and other technology on the modern battlefield has led to a demand for effective models specifically trained on military equipment. However, there has not been a proper effort to assemble or utilize data from recent wars for training future-oriented models. Creating new quality data poses costs and challenges that make it unrealistic for the sole purpose of training these models. This project explores a way around these barriers with the use of synthetic data generation using the Unreal Engine, a prominent computer graphics gaming engine. The ability to create computer-generated videos representative of the battlefield can impact model training and performance. I will be limiting the scope to focus on armored vehicles and the point of view of a consumer drone. Simulating a drone’s point of view in the Unreal Engine, I will create a collection of videos with ample variation. Using this data, I will experiment with various training methods to provide commentary on the best use of synthetic imagery for this task. If shown to be promising, this method can provide a feasible solution to prepare our models and military for what comes next.
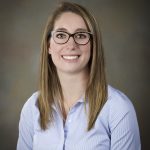
Meghan Sahakian
Principal Member of Technical Staff, Sandia National Laboratories
Design of In-Flight Cyber Experimentation for Spacecraft
Day 3, Room: B 1:00 PM-3:00 PM
Meghan Galiardi Sahakian is a Principal Member of the Technical Staff at Sandia National Laboratories. She earned her PhD in mathematics at University of Illinois Urbana-Champaign in 2016. She has since been at Sandia National Laboratories and her work focuses on cyber experimentation including topics such as design of experiments, modeling/simulation/virtualization, and quantitative cyber resilience metrics.
Abstract: Design of In-Flight Cyber Experimentation for Spacecraft
Improving the Quality of Test & Evaluation
Cyber resilience technologies are critical to ensuring the survival of mission critical assets for space systems. Such emerging cyber resilience technologies ultimately need to be proven out through in-flight experimentation. However, there are significant technical challenges for proving that new technologies actually enhance resilience of spacecraft. In particular, in-flight experimentation suffers from a “low data” problem due to many factors including 1) no physical access limits what types of data can be collected, 2) even if data can be collected, size, weight, and power (SWaP) constraints of the spacecraft make it difficult to store large amounts of data, 3) even if data can be stored, bandwidth constraints limit the transfer of data to the ground in a timely manner, and 4) only a limited number of trials can be performed due to spacecraft scheduling and politics. This talk will discuss a framework developed for design and execution of in-flight cyber experimentation as well as statistical techniques appropriate for analyzing the data. More specifically, we will discuss how data from ground-based test beds can be used to augment the results of in-flight experiments. The discussed framework and statistical techniques will be demonstrated on a use case.
Sandia National Laboratories is a multimission laboratory managed and operated by National Technology & Engineering Solutions of Sandia, LLC, a wholly owned subsidiary of Honeywell International Inc., for the U.S. Department of Energy’s National Nuclear Security Administration under contract DE-NA0003525. SAND2023-14847A.
Session Materials: https://dataworks.testscience.org/wp-content/uploads/formidable/23/Thrs_CS2_Sahakian.pptx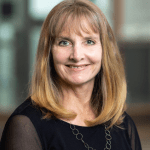
Dr. Missy Cummings
Professor, George Mason University
Day 3, Room: A+B 8:45 AM-9:25 AM
Professor Mary (Missy) Cummings received her B.S. in Mathematics from the US Naval Academy in 1988, her M.S. in Space Systems Engineering from the Naval Postgraduate School in 1994, and her Ph.D. in Systems Engineering from the University of Virginia in 2004. A naval officer and military pilot from 1988-1999, she was one of the U.S. Navy's first female fighter pilots. She is a Professor in the George Mason University College of Engineering and Computing and is the director of the Mason Autonomy and Robotics Center (MARC). She is an American Institute of Aeronautics and Astronautics (AIAA) Fellow, and recently served as the senior safety advisor to the National Highway Traffic Safety Administration. Her research interests include the application of artificial intelligence in safety-critical systems, assured autonomy, human-systems engineering, and the ethical and social impact of technology.

Mohammad Ahmed
NASA Program Data Analyst, OCFO
ADICT - A Power BI visualization tool used to transform budget forecasting.
Day 0, Room: C 9:50 AM-11:50 AM
Mohammad is a data analyst with over 10 years of experience in the field. Started out his career in the insurance industry as a data analyst in the actuarial field working on various risk-based projects and models which include Catastrophe modeling, pricing, program reviewing and reporting. He also has worked supporting Homeland Security in a Data role that also coincided with Data architecture and system administration.
In his current role, he is supporting the Strategic Insights and Budget division within the Office of Chief Financial Officer with scenario forecasting and creating new tools and dashboard's as well as all technical needs.
Abstract: ADICT - A Power BI visualization tool used to transform budget forecasting.
Sharing Analysis Tools, Methods, and Collaboration Strategies
AVATAR Dynamic Interactive Charting Tool (ADICT) is an advanced Power BI tool that has been developed by the National Aeronautics and Space Administration (NASA) to transform budget forecasting and assist in critical budget decision-making. This innovative tool leverages the power of the M language within Power BI to provide organizations with a comprehensive 15-year budget projection system that ensures real-time accuracy and efficiency. It is housed in Power BI for the capabilities to simultaneously update to the model from our excel file named AVATAR.
One of the standout features of ADICT is its capability to allow users to define and apply rate changes. This feature empowers organizations, including NASA, to customize their budget projections by specifying rate variations, resulting in precise and adaptable financial forecasting. NASA integrates ADICT to SharePoint to house the model to avoid local drives and seamlessly update the model to adjust for any scenario. This tool is used a scenario-based planner as well, as it can provide support for workforce planning and budget decisions.
ADICT seamlessly integrates with source Excel sheets, offering dynamic updates as data evolves. This integration eliminates the need for manual data manipulation, enhancing the overall decision-making process. It ensures that financial projections remain current and reliable, enabling organizations like NASA to respond swiftly to changing economic conditions and emerging challenges.
At its core, ADICT enhances budgeting by transforming complex financial data into interactive visualizations, enabling NASA to gain deeper insights into their financial data and make agile decisions. ADICT also seamlessly integrates with source Excel sheets, offering dynamic updates as data evolves. This integration eliminates the need for manual data manipulation, enhancing the overall decision-making process. It ensures that financial projections remain current and reliable for organizations to respond swiftly to changing economic conditions and emerging challenges.

Morgan Brown
Undergrad Researcher, USMA
Using AI to Classify Combat Vehicles in Degraded Environments
Day 2, Room: Cafe 5:00 PM-7:00 PM
Morgan Brown is originally from Phoenix, Arizona, but is currently attending the United States Military Academy pursuing an undergraduate degree in Mathematical Sciences. Throughout her academic career, she has worked on projects relating to modeling ideal fluid flow, data visualization, and developing unconventional communication platforms. She is now working on her senior thesis in order to graduate with honors in May of 2024.
Abstract: Using AI to Classify Combat Vehicles in Degraded Environments
Sharing Analysis Tools, Methods, and Collaboration Strategies
In the last decade, warfare has come to be characterized by rapid technological advances and the increased integration of artificial intelligence platforms. From China’s growing emphasis on advanced technological development programs to Ukraine’s use of facial recognition technologies in the war with Russia, the prevalence of artificial intelligence (AI) is undeniable. Currently, the United States is innovating the use of machine learning (ML) and AI through a variety of projects. Various systems use cutting-edge sensing technologies and emerging ML algorithms to automate the target acquisition process. As the United States attempts to increase its use of ATR and AiTR systems, it is important to consider the inaccuracy that may occur as a result of environmental degradations, such as smoke, fog, or rain. Therefore, this project aims to mimic various battlefield degradations through the implementation of different types of noise, namely Uniform, Gaussian, and Impulse noise to determine the effect of these various degradations on an Commercial-off-the-Shelf image classification system’s ability to correctly identify combat vehicles. This is an undergraduate research project which we wish to present via a Poster Presentation.

Nathan Wray
Senior Operator, DOT&E ACO
Cobalt Strike: A Cyber Tooling T&E Challenge
Day 3, Room: B 1:00 PM-3:00 PM
Dr. Nathan Wray is a technical lead and senior operator on the Advanced Cyber Operations team under the Office of the Director, Operational Test and Evaluation. Within his role, over the past seven years, Dr. Wray has performed red teaming, developed offensive cyber operations capabilities, and assisted cyber teams across the Department of Defense. Before his current role, Dr. Wray had over a decade of experience in operational and research-related positions in the private and public sectors. Dr. Wray's prior research and focus areas include leveraging machine learning to detect crypto-ransomware and researching offensive cyber capabilities, techniques, and related detection methods. Dr. Wray has Computer Engineering, Network Protection, and Information Assurance degrees and received his Doctorate of Science in Cybersecurity from Capitol Technology University in 2018.
Abstract: Cobalt Strike: A Cyber Tooling T&E Challenge
Improving the Quality of Test & Evaluation
Cyber Test and Evaluation serves a critical role in the procurement process of Red Team tools; however, once a tool is vetted and approved for use at the Red Team level, it is generally incorporated into their steady state operations without additional concern with regards to testing or maintenance of the tool. As a result, approved tools may not undergo routine in-depth T&E as new versions are released. This presents a major concern for the Red Team community as new versions can change the Operational Security of those tools. Similarly, cyber defenders - either through lack of training or limited resources - have been known to upload Red Team tools to commercial malware analysis platforms, which inadvertently releases potentially sensitive information about Red Team operations. The DOT&E Advanced Cyber Operations team, as part of the Cyber Assessment Program, performed in-depth analysis into Cobalt Strike, versions 4.8 and newer, an adversary simulation software widely used across the Department of Defense and the United States Government. Advanced Cyber Operations identified several operational security concerns that could disclose sensitive information to an adversary with access to payloads generated by Cobalt Strike. This highlights the need to improve the test and evaluation of cyber tooling, at a minimum, for major releases of tools utilized by Red Teams. Advanced Cyber Operations recommends in-depth, continuous test and evaluation of offensive operations tools and continued evaluation to mitigate potential operational security concerns.
Session Materials: https://dataworks.testscience.org/wp-content/uploads/formidable/23/Thrs_CS2_Wray-1.pptx
Nathan Gaw
Assistant Professor of Data Science, Air Force Institute of Technology
Assessing the Calibration and Performance of Attention-based Spatiotemporal Neural Network
Day 3, Room: B 9:50 AM-11:50 AM
Dr. Nathan Gaw is an Assistant Professor of Data Science in the Department of Operational Sciences at Air Force Institute of Technology, Wright-Patterson AFB, Ohio, USA. His research develops new statistical machine learning algorithms to optimally fuse high-dimensional, heterogeneous, multi-modality data sources to support decision making in military, healthcare and remote sensing. He received his B.S.E. and M.S. in biomedical engineering and a Ph.D. in industrial engineering from Arizona State University (ASU), Tempe, AZ, USA, in 2013, 2014, and 2019, respectively. Dr. Gaw was a Postdoctoral Research Fellow at the ASU-Mayo Clinic Center for Innovative Imaging (AMCII), Tempe, AZ, USA, from 2019-2020, and a Postdoctoral Research Fellow in the School of Industrial and Systems Engineering (ISyE) at Georgia Institute of Technology, Atlanta, GA, USA, from 2020-2021. He is also chair of the INFORMS Data Mining Society, and a member of IISE and IEEE. For additional information, please visit www.nathanbgaw.com.
Abstract: Assessing the Calibration and Performance of Attention-based Spatiotemporal Neural Network
Advancing Test & Evaluation of Emerging and Prevalent Technologies
In the last decade, deep learning models have proven capable of learning complex spatiotemporal relations and producing highly accurate short-term forecasts, known as nowcasts. Various models have been proposed to forecast precipitation associated with storm events hours before they happen. More recently, neural networks have been developed to produce accurate lightning nowcasts, using various types of satellite imagery, past lightning data, and other weather parameters as inputs to their model. Furthermore, the inclusion of attention mechanisms into these spatiotemporal weather prediction models has shown increases in the model’s predictive capabilities.
However, the calibration of these models and other spatiotemporal neural networks is rarely discussed. In general, model calibration addresses how reliable model predictions are, and models are typically calibrated after the model training process using scaling and regression techniques. Recent research suggests that neural networks are poorly calibrated despite being highly accurate, which brings into question how accurate the models are.
This research develops attention-based and non-attention-based deep-learning neural networks that uniquely incorporate reliability measures into the model tuning and training process to investigate the performance and calibration of spatiotemporal deep-learning models. All of the models developed in this research prove capable of producing lightning occurrence nowcasts using common remotely sensed weather modalities, such as radar and satellite imagery. Initial results suggest that the inclusion of attention mechanisms into the model architecture improves the model’s accuracy and predictive capabilities while improving the model’s calibration and reliability.
Session Materials: https://dataworks.testscience.org/wp-content/uploads/formidable/23/Wed_3B_Gaw.pptx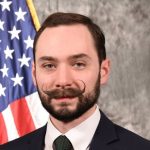
Nicholas Jones
STAT Expert, STAT COE
Case Study: State Transition Maps for Mission Model Development and Test Objective Identifiers
Day 3, Room: B 9:50 AM-11:50 AM
Mr. Nicholas Jones received his Master’s Degree in Materials Science and Engineering from the University of Dayton, Ohio. After working in mission performance analysis at Missile Defense Agency, he currently works at the Scientific Test and Analysis Techniques Center of Excellence (STAT COE) in direct consultation with DoD programs. Mr. Jones assists programs with test planning and analysis, and also supports STAT COE initiatives for Model Validation Levels (MVLs) and development of STAT to support Cyber T&E.
Abstract: Case Study: State Transition Maps for Mission Model Development and Test Objective Identifiers
Sharing Analysis Tools, Methods, and Collaboration Strategies
Coming soon
Session Materials: https://dataworks.testscience.org/wp-content/uploads/formidable/23/Thrs_4B_Jones.pptx
Nikolai Lipscomb
Research Staff Member, IDA
A Mathematical Programming Approach to Wholesale Planning
Day 2, Room: C 1:40 PM-3:10 PM
Nikolai is a Research Staff Member at the Institute for Defense Analyses and is part of the Sustainment Group within the Operational Evaluation Division, focusing on tasks for the Naval Supply Systems Command (NAVSUP).
Prior to IDA, Nikolai was a PhD student at the University of North Carolina at Chapel Hill where he received his doctorate from the Department of Statistics & Operations Research.
Nikolai's areas of research interest are optimization, supply networks, stochastic systems, and decision problems.
Abstract: A Mathematical Programming Approach to Wholesale Planning
The DOD’s materiel commands generally rely on working capital funds (WCFs) to fund their purchases of spares. A WCF insulates the materiel commands against the disruptions of the yearly appropriations cycle, and allows for long-term planning and contracting. A WCF is expected to cover its own costs by allocating its funds judiciously and adjusting the prices it charges to the end customer, but the multi-year lead times associated with most items means that items must be ordered years in advance of anticipated need. Being financially conservative (ordering less) leads to backorders, while minimizing backorders (ordering more) often introduces financial risk by buying items that may not be sold in a timely manner. In this work, we develop an optimization framework that produces a "Buy List" of repairs and procurements for each fiscal year. The optimizer seeks to maximize a financial and readiness-minded objective function subject to constraints such as budget limitations, contract priorities, and historical variability of demand signals. Buy Lists for each fiscal year provide a concrete baseline for examining the repair/procurement decisions of real wholesale planners and comparing performance via simulation of different histories.
Session Materials: https://dataworks.testscience.org/wp-content/uploads/formidable/23/Wed_2C_Lipscomb.pptx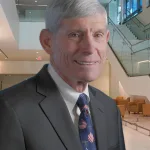
Gen. Norty Schwartz
President, IDA
Day 2, Room: A+B 8:30 AM-8:45 AM
Norty Schwartz serves as President of IDA where he directs the activities of more than 1,000 scientists and technologists.
Norty has a long and prestigious career of service and leadership that spans over five decades. He was most recently President and CEO of Business Executives for National Security (BENS). During his six-year tenure at BENS, he was also a member of IDA’s Board of Trustees.
Prior to retiring from the U.S. Air Force, he served as the 19th Chief of Staff of the U.S. Air Force from 2008 to 2012. He previously held senior joint positions as Director of the Joint Staff and as the Commander of the U.S. Transportation Command. He began his service as a pilot with the airlift evacuation out of Vietnam in 1975.
Norty is a U.S. Air Force Academy graduate and holds a master’s degree in business administration from Central Michigan University. He is also an alumnus of the Armed Forces Staff College and the National War College.
He is a member of the Council on Foreign Relations and a 1994 Fellow of Massachusetts Institute of Technology’s Seminar XXI. He has been married to Suzie since 1981.
Abstract:
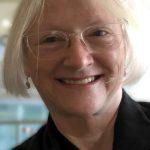
Dr. Pam Savage-Knepshield
CACI, International
Understanding and Applying the Human Readiness Level Scale During User-Centered Design
Day 1, Room: B 9:00 AM-4:00 PM
Pam Savage-Knepshield is employed by CACI, International as the user-centered design (UCD) lead for Army Field Artillery Command and Control Systems supporting the U.S. Army Project Manager Mission Command in Aberdeen Proving Ground, Maryland. She has a doctorate degree in cognitive psychology and is a Fellow of the Human Factors and Ergonomics Society. With over 35 years of human factors experience working in industry, academia, and the US Army, her interests focus on user-centered design from front-end development identifying user needs and translating them into user stories, through usability testing and post-fielding user satisfaction assessment.
Abstract: Understanding and Applying the Human Readiness Level Scale During User-Centered Design
The purpose of this short course is to support knowledge and application of the Human Readiness Level (HRL) scale described in ANSI/HFES 400-2021 Human Readiness Level Scale in the System Development Process. The HRL scale is a simple nine-level scale designed to supplement the Technology Readiness Level (TRL) scale to evaluate, track, and communicate the readiness of a technology or system for safe and effective human use. Application of the HRL scale ensures proper attention to human systems design throughout system development, which minimizes or prevents human error and enhances the user experience.
Learning objectives for the short course include:
(1) Understand the relationship between a user-centered design (UCD) process and the HRL Scale. Instructors will discuss a “typical” UCD process describing the design activities and data collected that support HRL Scale evaluation and tracking.
(2) Learn effective application of usability testing in a DOD environment. Instructors will describe iterative, formative usability testing with a hands-on opportunity to perform usability tasks. Human-centered evaluation of system design is a critical activity when evaluating the extent to which a system is ready for human use.
(3) Understand HFES 400-2021 development and contents. Instructors will describe the evolution of the HRL concept to convey its significance and the rigor behind the development of the technical standard. Instructors will walk through major sections of the standard and describe how to apply them.
(4) Learn how the HRL scale is applied in current and historical acquisition programs. Instructors will describe real-world Army applications of the HRL scale, including a case study of a software modernization program.
(5) Apply the HRL scale to practical real-world problems. Attendees will gain hands-on experience applying the HRL scale during group exercises that simulate teamwork during the system development process. Group exercises incorporate three different scenarios, being both hardware and software solutions at various stages of technological development. The hands-on exercises specifically address common questions about the practical use of the HRL scale. Course attendees do not need prior human factors/ergonomics knowledge or ability. The HRL scale is intended to be applied by human systems professionals with proper ability and experience; however, recipients of HRL scale ratings include many other types of personnel in design, engineering, and acquisition as well as high-level decision-makers, all of whom benefit from understanding the HRL scale. Before attending the course, students should download a free copy of the ANSI/HFES technical standard at https://my.hfes.org/online-store/publications and bring it to the course in electronic or hard copy format. Laptops are not necessary for the course but may facilitate notetaking and completion of the group exercises.

Patricia Gallagher
Data Scientist, Jet Propulsion Laboratory
Optimizing Mission Concept Development: A Bayesian Approach Utilizing DAGs
Day 2, Room: C 10:30 AM-12:30 PM
Patricia Gallagher is a Data Scientist at Jet Propulsion Laboratory (JPL) in the Systems Modeling, Analysis & Architectures group. In her current role, Patricia focuses on supporting mission formulation through the development of tools and models, with a particular emphasis on cost modeling. Prior to this, she spent four years as a Project Resource Analyst at JPL, gaining a strong foundation in the business operations side of missions. Patricia holds a Bachelor's degree in Economics with a minor in Mathematics from California State University, Los Angeles, and a Master of Science in Data Science from University of California, Berkeley.
Abstract: Optimizing Mission Concept Development: A Bayesian Approach Utilizing DAGs
This study delves into the application of influence diagrams in mission concept development at the Jet Propulsion Laboratory, emphasizing the importance of how technical variables influence mission costs. Concept development is an early stage in the design process that requires extensive decision-making, in which the tradeoffs between time, money, and scientific goals are explored to generate a wide range of project ideas from which new missions can be selected. Utilizing influence diagrams is one strategy for optimizing decision making. An influence diagram represents decision scenarios in a graphical and mathematical manner, providing an intuitive interpretation of the relationships between input variables (which are functions of the decisions made in a trade space) and outcomes. These input-to-outcome relationships may be mediated by intermediate variables, and the influence diagram provides a convenient way to encode the hypothesized “trickle-down” structure of the system. In the context of mission design and concept development, influence diagrams can inform analysis and decision-making under uncertainty to encourage the design of realistic projects within imposed cost limitations, and better understand the impacts of trade space decisions on outcomes like cost.
This project addresses this initiative by focusing on the analysis of an influence diagram framed as a Directed Acyclic Graph (DAG), a graphical structure where vertices are connected by directed edges that do not form loops. Edge weights in the DAG represent the strength and direction of relationships between variables. The DAG aims to model the trickle-down effects of mission technical parameters, such as payload mass, payload power, delta V, and data volume, on mission cost elements. A Bayesian multilevel regression model with random effects is used for estimating the edge weights in a specific DAG (constructed according to expert opinion) that is meant to represent a hypothesized trickle-down structure from technical parameters to cost. This Bayesian approach provides a flexible and robust framework, allowing us to incorporate prior knowledge, handle small datasets effectively, and leverage its capacity to capture the inherent uncertainty in our data.
Session Materials: https://dataworks.testscience.org/wp-content/uploads/formidable/23/Wed_1C_Gallagher.pptx
Patrick Bjornstad
Systems Engineer I, Jet Propulsion Laboratory
Unlocking our Collective Knowledge: LLMs for Data Extraction from Long-Form Documents
Day 3, Room: A 1:00 PM-3:00 PM
Patrick Bjornstad is a Systems Engineer / Data Scientist at Jet Propulsion Laboratory in the Systems Modeling, Analysis & Architectures group. With expertise in a range of topics including statistical modeling, machine/deep learning, software development, and data engineering, Patrick has been involved with a variety of projects, primarily supporting formulation work at JPL. Patrick earned a B.S. in an Applied & Computational Mathematics and an M.S. in Applied Data Science at the University of Southern California (USC).
Abstract: Unlocking our Collective Knowledge: LLMs for Data Extraction from Long-Form Documents
Advancing Test & Evaluation of Emerging and Prevalent Technologies
As the primary mode of communication between humans, natural language (oftentimes found in the form of text) is one of the most prevalent sources of information across all domains. From scholarly articles to industry reports, textual documentation pervades every facet of knowledge dissemination. This is especially true in the world of aerospace. While other structured data formats may struggle to capture complex relationships, natural language excels by allowing for detailed explanations that a human can understand. However, the flexible, human-centered nature of text has made it traditionally difficult to incorporate into quantitative analyses, leaving potentially valuable insights and features hidden within the troves of documents collecting dust in various repositories.
Large Language Models (LLMs) are an emerging technology that can bridge the gap between the expressiveness of unstructured text and the practicality of structured data. Trained to predict the next most likely word following a sequence of text, LLMs built on large and diverse datasets must implicitly learn knowledge related to a variety of fields in order to perform prediction effectively. As a result, modern LLMs have the capability to interpret the underlying semantics of language in many different contexts, allowing them to digest long-form, domain-specific textual information in a fraction of the time that a human could. Among other things, this opens up the possibility of knowledge extraction: the transformation of unstructured textual knowledge to a structured format that is consistent, queryable, and amenable to being incorporated in future statistical or machine learning analyses.
Specifically, this work begins by highlighting the use of GPT-4 for categorizing NASA work contracts based on JPL’s organizational structure using textual descriptions of the contract’s work, allowing the lab to better understand how different divisions will be impacted by the increasingly outsourced work environment. Despite its simplicity, the task demonstrates the capability of LLMs to ingest unstructured text and produce structured results (categorical features for each contract indicating the JPL organization that the work would involve) useful for statistical analysis. Potential extensions to this proof of concept are then highlighted, such as the generation of knowledge-graphs/ontologies to encode domain and mission-specific information. Access to a consistent, structured graphical knowledge base would not only improve data-driven decision making in engineering contexts by exposing previously out-of-reach data artifacts to traditional analyses (e.g., numerical data extracted from text, or even graph embeddings which encode entities/nodes as vectors in a way that captures the entity’s relation to the overall structure of the graph), but could also accelerate the development of specialized capabilities like the mission Digital Twin (DT) by enabling access to a reliable, machine-readable database of mission and domain expertise.
Session Materials: https://dataworks.testscience.org/wp-content/uploads/formidable/23/Thrs_CS1_Bjornstad.pptxDr. Pete Parker
Statistician and Team Lead, NASA
Day 3, Room: 3:15 PM-4:00 PM
Abstract:

Peter Juarez
Research Engineer, NASA
Applications for inspection simulation at NASA
Day 2, Room: C 10:30 AM-12:30 PM
Peter Juarez is a research engineer who specializes in multiple fields of NDE including Design for Inspection (DFI), NDE modeling ultrasound, thermography, guided wave, artificial flaw manufacturing and automated data processing. Peter implements these skills in the fulfillment of both commercial aviation and NASA space programs, such as the Advanced Composites Project (ACP), High Rate Composites Advanced Manufacturing (HiCAM), Orion capsule heat shield inspection, and the Advanced Composite Solar Sail System (ACS3).
Abstract: Applications for inspection simulation at NASA
Sharing Analysis Tools, Methods, and Collaboration Strategies
The state of the art of numerical simulation of nondestructive evaluations (NDE) has begun to transition from research to application. The simulation software, both commercial and custom, has reached a level of maturity where it can be readily deployed to solve real world problems. The next area of research that is beginning to emerge is determining when and how to NDE simulation should be applied. At NASA Langley Research Center, NDE simulations have already been utilized for several aerospace projects to facilitate or enhance understanding of the inspection optimization and interpretation of results. Researchers at NASA have identified several different scenarios where it is appropriate to utilized NDE simulations. In this presentation, we will describe these scenarios, give examples of each instance, and demonstrate how NDE simulations were applied to solve problems with an emphasis on the mechanics of integrating with other workgroups. These examples will include inspection planning for multi-layer pressure vessels as well as on-orbit inspections.
Session Materials: https://dataworks.testscience.org/wp-content/uploads/formidable/23/Wed_1C_Juarez.pptx
Peter Parker
Statistician, NASA
Practical Experimental Design Strategies for Binary Responses under Operational Constraints
Day 2, Room: A 3:30 PM-5:00 PM
Peter A. Parker and Nathan Cruze
Abstract: Practical Experimental Design Strategies for Binary Responses under Operational Constraints
Defense and aerospace testing commonly involves binary responses to changing levels of a system configuration or an explanatory variable. Examples of binary responses are hit or miss, detect or not detect, and success or fail, and they are a special case of categorical responses with multiple discreet levels. The test objective is typically to estimate a statistical model that predicts the probability of occurrence of the binary response as a function of the explanatory variable(s). Statistical approaches are readily available for modeling binary responses; however, they often assume that the design features large sample sizes that provide responses distributed across the range of the explanatory variable. In practice, these assumptions are often challenged by small sample sizes and response levels focused over a limited range of the explanatory variable(s). These practical restrictions are due to experimentation cost, operational constraints, and a primary interest in one response level, e.g., testing may be more focused on hits compared to a misses. This presentation provides strategies to address these challenges with an emphasis on collaboration techniques to develop experimental design approaches under practical constraints. Case studies are presented to illustrate these strategies from estimating human annoyance to low noise supersonic overflights in NASA’s Quesst mission and evaluating detection capability of nondestructive evaluation methods for fracture-critical human-spaceflight components. This presentation offers practical guidance on experimental design strategies for binary responses under operational constraints.
Session Materials: https://dataworks.testscience.org/wp-content/uploads/formidable/23/Wed_3A_Parker.pdf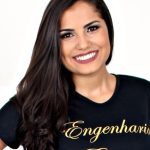
Priscila Silva
Graduate Research Assistant, University of Massachusetts Dartmouth, Department of Electrical and Computer Engineering
Regression and Time Series Mixture Approaches to Predict Resilience
Day 2, Room: B 3:30 PM-5:00 PM
Priscila Silva is a Ph.D. candidate in Electrical and Computer Engineering at University of Massachusetts Dartmouth (UMassD). She received her MS degree in Computer Engineering from UMassD in 2022, and her BS degree in Electrical Engineering from Federal University of Ouro Preto (UFOP) in 2017. Her research interests include system reliability and resilience engineering for performance predictions, including computer, cyber-physical,
infrastructure, finance, and environment domains.
Abstract: Regression and Time Series Mixture Approaches to Predict Resilience
Improving the Quality of Test & Evaluation
Resilience engineering is the ability to build and sustain a system that can deal effectively with disruptive events. Previous resilience engineering research focuses on metrics to quantify resilience and models to characterize system performance. However, resilience metrics are normally computed after disruptions have occurred and existing models lack the ability to predict one or more shocks and subsequent recoveries. To address these limitations, this talk presents three alternative approaches to model system resilience with statistical techniques based on (i) regression, (ii) time series, and (iii) a combination of regression and time series to track and predict how system performance will change when exposed to multiple shocks and stresses of different intensity and duration, provide structure for planning tests to assess system resilience against particular shocks and stresses and guide data collection necessary to conduct tests effectively. These modeling approaches are general and can be applied to systems and processes in multiple domains. A historical data set on job losses during the 1980 recessions in the United States is used to assess the predictive accuracy of these approaches. Goodness-of-fit measures and confidence intervals are computed, and interval-based and point-based resilience metrics are predicted to assess how well the models perform on the data set considered. The results suggest that resilience models based on statistical methods such as multiple linear regression and multivariate time series models are capable of modeling and predicting resilience curves exhibiting multiple shocks and subsequent recoveries. However, models that combine regression and time series account for changes in performance due to current and time-delayed effects from disruptions most effectively, demonstrating superior performance in long-term predictions and higher goodness-of-fit despite increased parametric complexity.
Session Materials: https://dataworks.testscience.org/wp-content/uploads/formidable/23/Wed_SS_Silva.pdfRachel Sholder and Kathy Kha
Cost Considerations for Estimating Small Satellite Integration & Test
Day 3, Room: C 9:50 AM-11:50 AM
Rachel Sholder is a parametric cost analyst within the Systems Engineering Group of the APL Space Exploration Sector. She joined APL in 2017 after graduating with M.S. in Statistics and B.S. in Mathematics from Lehigh University. Rachel has since become a valuable team member responsible for life-cycle space mission cost estimates at various stages of programmatic development (pre-proposal, proposal, mission milestone, trade studies, etc.). She is an active participant in the NASA Cost Estimating Community. Rachel was named the “NASA Cost and Schedule Rising Star” in 2023. Rachel is currently working towards a Doctor of Engineering degree with a focus in applied mathematics and statistics.
Kathy Kha is a parametric cost analyst within the Systems Engineering Group in the Space Exploration Sector at The Johns Hopkins University Applied Physics Laboratory (APL). She has been working at APL since 2018 and is APL’s subject-matter expert in parametric cost analysis. At APL, she is responsible for life-cycle space mission cost estimates at various stages of programmatic development (pre-proposal, proposal, mission milestone, trade studies, etc.). Prior to joining APL, her work included consulting engagements providing cost estimates and proposal evaluation support for NASA source selection panels for space science and Earth science missions and cost estimating support at NASA Ames Research Center. She has a bachelor’s degree in Applied Mathematics from the University of California – San Diego, a master’s degree in Systems Engineering from the University of Southern California and a doctorate in engineering from The Johns Hopkins University.
Abstract: Cost Considerations for Estimating Small Satellite Integration & Test
In the early phases of project formulation, mission integration and test (I&T) costs are typically estimated via a wrap factor approach, analogies to similar missions adjusted for mission specifics, or a Bottom Up Estimate (BUE). The wrap factor approach estimates mission I&T costs as a percentage of payload and spacecraft hardware costs. This percentage is based on data from historical missions, with the assumption that the project being estimated shares similar characteristics with the underlying data set used to develop the wrap factor. This technique has worked well for traditional spacecraft builds since typically as hardware costs grow, I&T test costs do as well. However, with the emergence of CubeSats and nanosatellites, the cost basis of hardware is just not large enough to use the same approach. This suggests that there is a cost “floor” that covers basic I&T tasks, such as a baseline of labor and testing.
This paper begins the process of developing a cost estimating relationship (CER) for estimating Small Satellite (SmallSat) Integration & Test (I&T) costs. CERs are a result of a cost estimating methodology using statistical relationships between historical costs and other program variables. The objective in generating a CER equation is to show a relationship between the dependent variable, cost, to one or more independent variables. The results of this analysis can be used to better predict SmallSat I&T costs.
Session Materials: https://dataworks.testscience.org/wp-content/uploads/formidable/23/Thrs_4C_Sholder_Kha-2.pptxRobert Edman
Machine Learning Research Scientist, Software Engineering Institute
Sensor Fusion for Automated Gathering of Labeled Data in Edge Settings
Day 3, Room: A 1:00 PM-3:00 PM
This work is a collaboration between the Army AI Integration Center and the Software Engineering Institute. Collaborators include Dr. Robert Edman, CPT Bryce Wilkins , Dr. Jose Morales who focus on the rapid maturation of basic research into Army relevant capabilities, particularly through testing using Army relevant data and metrics.
Abstract: Sensor Fusion for Automated Gathering of Labeled Data in Edge Settings
Data labeling has been identified as the most significant bottleneck and expense in the development of ML enabled systems. High quality labeled data also plays a critical role in the testing and deployment of AI/ML enabled systems, by providing a realistic measurement of model performance in a realistic environment. Moreover, the lack of agreement on test and production data is a commonly cited failure mode for ML systems.
This work focuses on methods for automatic label acquisition using sensor fusion methods, specifically in edge settings where multiple sensors, including multi-modal sensors, provide multiple views of an object. When multiple sensors provide probable detection of an object, the detection capabilities of the overall system (as opposed to those of each component of the system) can be improved to highly probable or nearly certain. This is accomplished via a system network of belief propagation that fuses the observations of an object from multiple sensors. These nearly certain detections can, in turn, be used as labels in a semi-supervised like manner. Once the detection likelihood exceeds a specified threshold, the data and the associated label can be used in retraining to produce higher performing models in near real time to improve overall detection capabilities.
Automated edge retraining scenarios provide a particular challenge for test and evaluation because it also requires high confidence tests that generalize to potentially unseen environments. The rapid and automated collection of labels enables edge retraining, federated training, dataset construction, and improved model performance. Additionally, improved model performance is an enabling capability for downstream system tasks, including more rapid model deployment, faster time to detect, fewer false positives, simplified data pipelines, and decreased network bandwidth requirements.
To demonstrate these benefits, we have developed a scalable reference architecture and dataset that allows repeatable experimentation for edge retraining scenarios. This architecture allows exploration of the complex design space for sensor fusion systems, with variation points including: methods for belief automation, automated labeling methods, automatic retraining triggers, and drift detection mechanisms. Our reference architecture exercises all of these variation points using multi-modal data (overhead imaging, ground-based imaging, and acoustic data).
Session Materials: https://dataworks.testscience.org/wp-content/uploads/formidable/23/Thrs_CS1_Edman.pdf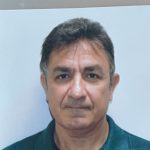
Roger Ghanem
Professor, University of Southern California
Introduction to Uncertainty Quantification
Day 2, Room: D 10:30 AM-12:30 PM
Roger Ghanem is Professor of Civil and Environmental Engineering at the U of Southern California where he also holds the Tryon Chair in Stochastic Methods and Simulation. Ghanem's research is in the general areas of uncertainty quantification and computational science with a focus on coupled phenomena. He received his PhD from Rice University and had served on the faculty of SUNY-Buffalo and Johns Hopkins University before joining USC in 2005.
Abstract: Introduction to Uncertainty Quantification
Advancing Test & Evaluation of Emerging and Prevalent Technologies
Uncertainty quantification (UQ) sits at the confluence of data, computers, basic science, and operation. It has emerged with the need to inform risk assessment with rapidly evolving science and to bring the full power of sensing and computing to bear on its management.
With this role, UQ must provide analytical insight into several disparate disciplines, a task that may seem daunting and highly technical. But not necessarily so.
In this mini-tutorial, I will present foundational concepts of UQ, showing how it is the simplicity of the underlying ideas that allows them to straddle multiple disciplines. I will also describe how operational imperatives have helped shape the evolution of UQ and discuss how current research at the forefront of UQ can in turn affect these operations.

Russell Gilabert
Researcher/Engineer, NASA Langley Research Center
Simulated Multipath Using Software Generated GPS Signals
Day 3, Room: B 1:00 PM-3:00 PM
Russell Gilabert is a computer research engineer in the Safety Critical Avionics Systems Branch at NASA Langley Research Center. Russell received his MSc in electrical engineering from Ohio University in 2018. His research is currently focused on GNSS augmentation techniques and dependable navigation for autonomous aerial vehicles.
Abstract: Simulated Multipath Using Software Generated GPS Signals
Advancing Test & Evaluation of Emerging and Prevalent Technologies
Depending on the environment, multipath can be one of the largest error sources contributing to degradation in Global Navigation Satellite System (GNSS) (e.g., GPS) performance. Multipath is a phenomenon that occurs as radio signals reflect off of surfaces, such as buildings, producing multiple copies of the original signal. When this occurs with GPS signals, it results in one or more delayed signals arriving at the receiver with or without the on-time/direct GPS signal. The receiver measures the composite of these signals which, depending on the severity of the multipath, can substantially degrade the accuracy of the receiver's calculated position. Multipath is commonly experienced in cities due to tall buildings and its mitigation is an ongoing area of study. This research demonstrates a novel approach for simulating GPS multipath through the modification of an open-source tool, GPS-SDR-SIM. The resulting additional testing capability could allow for improved development of multipath mitigating technologies.
Currently, open-source tools for simulating GPS signals are available and can be used in the testing and evaluation of GPS receiver equipment. These tools can generate GPS signals that, when used by a GPS receiver, result in computation of a position solution that was pre-determined at the time of signal generation. That is, the signals produced are properly formed for the pre-determined location and result in the receiver reporting that position. This allows for a GPS receiver under test to be exposed to various simulated locations and conditions without having to be physically subjected to them. Additionally, while these signals are generated by a software simulation, they can be processed by real or software defined GPS receivers. This work utilizes the GPS-SDR-SIM software tool for GPS signal generation and while this tool does implement some sources of error that are inherent to GPS, it cannot inject multipath. GPS-SDR-SIM was modified in this effort to produce additional copies of signals with pre-determined delays. These additional delayed signals mimic multipath and represent what happens to GPS signals in the real world as they reflect off of surfaces and arrive at a receiver in place of or alongside the direct GPS signal.
A successful proof of concept was prototyped and demonstrated using this modified version of GPS-SDR-SIM to produce simulated GPS signals as well as additional simulated multipath signals. The generated data was processed using a software defined GPS receiver and it was found that the introduction of simulated multipath signals successfully produced the expected characteristics of a composite multipath signal. Further maturation of this work could allow for the development of a GPS receiver testing and evaluation framework and aid in the development of multipath mitigating technologies.
Session Materials: https://dataworks.testscience.org/wp-content/uploads/formidable/23/Thrs_CS2_Gilabert.pptx
Russell Kupferer
Naval Warfare Action Officer, DOT&E
Threat Integration for Full Spectrum Survivability Assessments
Day 2, Room: A 10:30 AM-12:30 PM
Russell Kupferer is an Action Officer in the Naval Warfare directorate of the office of Director, Operational Test and Evaluation (DOT&E). In this role, he provides oversight of the Live Fire Test and Evaluation (LFT&E) programs for all USN ships and submarines. Mr. Kupferer received his bachelor’s degree in Naval Architecture and Marine Engineering at Webb Institute.
Abstract: Threat Integration for Full Spectrum Survivability Assessments
Advancing Test & Evaluation of Emerging and Prevalent Technologies
The expansion of DOT&E’s oversight role to cover full spectrum survivability and lethality assessments includes a need to reexamine how threats are evaluated in a Live Fire Test and Evaluation (LFT&E) rubric. Traditionally, threats for LFT&E assessment have been considered in isolation, with focus on only conventional weapon hits that have the potential to directly damage the system under test. The inclusion of full spectrum threats - including electronic warfare, directed energy, CBRNE, and cyber - requires a new approach to how LFT&E assessments are conducted. Optimally, assessment of full spectrum threats will include integrated survivability vignettes appropriate to how our systems will actually be used in combat and how combinations of adversary threats are likely to be used against them. This approach will require new assessment methods with an increased reliance on data from testing at design sites, component/surrogate tests, and digital twins.
Session Materials: https://dataworks.testscience.org/wp-content/uploads/formidable/23/Wed_1A_Kupferer.pptx
Ryan Krolikowski
CDT, United States Military Academy
Command Slating Sensitivity Analysis
Day 2, Room: Cafe 5:00 PM-7:00 PM
I am currently a cadet at the United States Military Academy at West Point, pursuing an undergraduate degree in Operations Research.
Abstract: Command Slating Sensitivity Analysis
Improving the Quality of Test & Evaluation
The Army's Human Resources Command (HRC) annually takes on the critical task of the Centralized Selection List (CSL) process, where approximately 400 officers are assigned to key battalion command roles. This slating process is a cornerstone of the Army's broader talent management strategy, involving collaborative input from branch proponent officers and culminating in the approval of the Army Chief of Staff. The study addresses crucial shortcomings in the existing process for officer assignments, focusing on the biases and inconsistent weighting that affect slate selection outcomes. It examines the effects of incorporating specific criteria like Skill Experience Match (SEM), Knowledge, Skills, Behaviors (KSB), Order of Merit List (OML), and Officer Preferences (PREF) into the selection process of a pilot program. Our research specifically addresses the terms efficiency, strength, and weakness within the context of the pairing process. Our objective is to illuminate the potential advantages of a more comprehensive approach to decision-making in officer-job assignments, ultimately enhancing the effectiveness of placing the most suitable officer in the most fitting role.
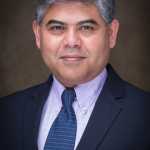
Sambit Bhattacharya
Professor, Fayetteville State University
AI for Homeland Security: A Comprehensive Approach for Detecting Sex Trafficking
Day 2, Room: Cafe 5:00 PM-7:00 PM
Sambit Bhattacharya is a Computer Scientist with more than 15 years of experience in teaching and research. He has a PhD in Computer Science and Engineering from the State University of New York at Buffalo. He is a tenured Full Professor of Computer Science at Fayetteville State University, North Carolina, USA. In 2023 he was honored by the University of North Carolina (UNC) Board of Governor’s Award for Teaching Excellence. Dr. Bhattacharya is experienced in developing and executing innovative and use-inspired research in Artificial Intelligence and Machine Learning (AIML) with a broad range of techniques and applications, and with multidisciplinary teams. He has more than 60 peer reviewed publications and has delivered 50+ oral presentations, including keynote lectures at conferences. Dr. Bhattacharya works on research in the applications of AIML to geospatial intelligence, computer vision with synthetic data for target recognition, efficiency and latency reducing inferencing for edge computing, automation and manufacturing. Dr. Bhattacharya leads projects funded by the National Science Foundation, the US Department of Defense (DoD), including support for research aligned with interests of the US Intelligence Community (IC), NASA, the North Carolina Department of Transportation, and the University of North Carolina Research Opportunities Initiative. He directs the Intelligent Systems Lab (ISL) at Fayetteville State University which hosts research and houses resources like robotics equipment, and high-performance computing for AIML research. The ISL supports faculty advisors and students, and collaborations with external partners. He is a Senior Member of the Institute of Electrical and Electronics Engineers (IEEE). Beginning in 2014 and while on teaching leave from the university, Dr. Bhattacharya served as faculty research fellow in the following research labs of the DoD and the IC: Naval Research Lab (DC), Army Research Lab (Adelphi, MD), and the National Geospatial Intelligence Agency (NGA). He has been appointed as Visiting Scientist (part-time) at NGA starting 2023. He was Faculty in Residence at Google’s Global HQ in Mountain View, CA in 2017 and he collaborates with industry through grant funded partnerships and consulting opportunities.
Abstract: AI for Homeland Security: A Comprehensive Approach for Detecting Sex Trafficking
Sharing Analysis Tools, Methods, and Collaboration Strategies
Sex trafficking remains a global problem, requiring new innovations to detect and disrupt such criminal enterprises. Our research project is an application of artificial intelligence (AI) methods, and the knowledge of social science and homeland security to the detection and understanding of the operational models of sex trafficking networks (STNs). Our purpose is to enhance the AI capabilities of software-based detection technologies and support the homeland defense community in detecting and countering human sex trafficking, including the trafficking of underage victims.
To accomplish this, we propose a novel architecture capable of jointly representing and learning from multiple modalities, including images and text. The interdisciplinary nature of this work involves the fusion of computer vision, natural language processing, and deep neural networks (DNNs) to address the complexities of sex trafficking detection from online advertisements. This research proposes the creation of a software prototype as an extension of the Image Surveillance Assistant (ISA) built by our research team, to focus on cross-modal information retrieval and context understanding critical for identifying potential sex trafficking cases. Our initiative aligns with the objectives outlined in the DHS Strategic Plan, aiming to counter both terrorism and security threats, specifically focusing on the victim-centered approach to align with security threat segments.
We leverage current AI and machine learning techniques integrated by the project to create a working software prototype. DeepFace, a DNN for biometric analysis of facial image features such as age, race, and gender from images is utilized. Few-shot text classification, utilizing the SciKit Learn Python library and Large Language Models (LLMs), is enabling the detection of written trafficking advertisements. The prime funding agency, the Department of Homeland Security (DHS) mandates the use of synthetic data for this unclassified project, so we have developed code to leverage Application Programming Interfaces (APIs) that connect to LLMs and generative AI for images to create synthetic training and test data for the DNN models. Test and evaluation with synthetic data are the core capabilities of our approach to build prototype software that can potentially be used for real applications with real data.
Ongoing work includes creating a program to fuse the outputs from AI models on a single advertisement input. The fusion program will provide the numeric value of the likelihood of the class of advertisement, ranging from classes such as legal advertisement to different categories of trafficking. This research project is a potential contribution to the development of deployment-ready software for intelligence agencies, law enforcement, and border security. We currently show high accuracy for detecting advertisements related to victims of specific demographic categories. We have identified areas where increased accuracy is needed, and we are collecting more training data to address those gaps. The AI-based capabilities emerging from our research hold promise for enhancing the understanding of STN operational models, addresses technical challenges of sex trafficking detection, and also emphasizes the broader societal impact and alignment with national security goals.
Sarah Shaffer
Research Staff Member, IDA
Meta-analysis of the SALIANT procedure for assessing team situation awareness
Day 2, Room: B 1:40 PM-3:10 PM
Dr. Sarah Shaffer received her Ph.D. in Experimental Psychology from Florida International University. Her research focuses on the application of cognitive science principles in decision-
making and problem-solving, strategy, and attention and memory. Her research spans a variety of topics including information management in decision-making and intelligence collection, deception detection, and elicitation. Upon completing her doctorate, she pursued a fellowship working with federal law enforcement to conduct research in areas including stochastic terrorism, deception, and cybercrime.
Dr. Shaffer is currently a Research Staff Member at the Institute for Defense Analyses (IDA), where she focuses on operations testing and evaluation in the areas of Human Systems
Integration, Human-Computer Interaction, and test design.
Abstract: Meta-analysis of the SALIANT procedure for assessing team situation awareness
Many Department of Defense (DoD) systems aim to increase or maintain Situational Awareness (SA) at the individual or group level. In some cases, maintenance or enhancement of SA is listed as a primary function or requirement of the system. However, during test and evaluation SA is examined inconsistently or is not measured at all. Situational Awareness Linked Indicators Adapted to Novel Tasks (SALIANT) is an empirically-based methodology meant to measure SA at the team, or group, level. While research using the SALIANT model suggests that it effectively quantifies team SA, no study has examined the effectiveness of SALIANT across the entirety of the existing empirical research. The aim of the current work is to conduct a meta-analysis of previous research to examine the overall reliability of SALIANT as an SA measurement tool. This meta-analysis will assess when and how SALIANT can serve as a reliable indicator of performance at testing. Additional applications of SALIANT in non-traditional operational testing domains will also be discussed.
Session Materials: https://dataworks.testscience.org/wp-content/uploads/formidable/23/Wed_2B_Shaffer.pptx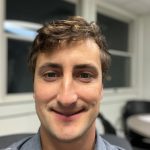
Shane Hall
Division Chief - Analytics and Artificial Intelligence, Army Evaluation Center
Functional Data Analysis of Radar Tracking Data
Day 2, Room: Cafe 5:00 PM-7:00 PM
Shane Hall is the Division Chief of the Analytics and Artificial Intelligence Division within the Army Evaluation Center. Shane graduated from Penn State University in 2011 with a Bachelor's degree in Statistics and a Masters of Applied Statistics. Shane began his Army civilian career immediately after college, working for the Army Public Health Command as their statistician. In 2016, he moved to the Army Evaluation Center as a statistician. In 2022, he became the Division Chief of the Analytics team and newly formed Artificial Intelligence Team.
Abstract: Functional Data Analysis of Radar Tracking Data
Sharing Analysis Tools, Methods, and Collaboration Strategies
Functional data are an ordered series of data collected over a continuous scale, such as time or distance. The data are collected in ordered x,y pairs and can be viewed as a smoothed line with an underlying function. The Army Evaluation Center (AEC) has identified multiple instances where functional data analysis could have been applied, but instead evaluators used more traditional and/or less statistically rigorous methods to evaluate the data. One of these instances is radar tracking data.
This poster highlights historical shortcomings of how AEC currently analyzes functional data, such as radar tracking data and our vision for future applications. Using a notional data from a real radar example, the response of 3D track error is plotted against distance, where each function represents a unique run number and additional factors held constant throughout a given run. The example includes the selected model, functional principle components, the resulting significant factors, and summary graphics used for report. Additionally, the poster will highlight historical analysis methods and the improvements the functional data analysis method brings. The analysis and output from this poster will utilize JMP's functional data analysis platform.
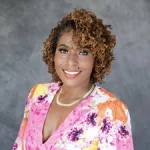
Ms. SherAaron Hurt
The Carpentries
R for Reproducible Scientific Analysis
Day 1, Room: C 9:00 AM-4:00 PM
SherAaron (Sher!) Hurt is the Director of Workshops and Instruction for The Carpentries, an organisation that teaches foundational coding and data science skills to researchers worldwide. As the Director of Workshops and Training she provides strategy, oversight, and overall management, planning, vision, and leadership for The Carpentries Workshops and Instruction Team. She oversees and support all administration, communications, and data entry aspects for workshops. Sher! oversees and supports all Instructor and Trainer training aspects, including curriculum development and maintenance, certification, and community management. Sher! supports the certified Instructor community by developing appropriate programming, communications processes, and workflows. She develop resources and workflows to ensure the overall health of workshops and the Instructor Training and Trainer Training programs. She earned her B.S. in Business Management at Michigan Technological University and M.A. degree in Hospitality Management at Florida International University. Sher! resides in Detroit, MI where she enjoys travel and fitness.
Abstract: R for Reproducible Scientific Analysis
The Carpentries more introductory R lesson. In addition to their standard content, this workshop covers data analysis and visualization in R, focusing on working with tabular data and other core data structures, using conditionals and loops, writing custom functions, and creating publication-quality graphics. As their more introductory R offering, this workshop also introduces learners to RStudio and strategies for getting help. This workshop is appropriate for learners with no previous programming experience.
Session Materials Website: https://barneyricca.github.io/2024-04-16-ida/

Starr D'Auria
NDE Engineer, Extende
CIVA NDT Simulation: Improving Inspections Today for a Better Tomorrow
Day 2, Room: C 10:30 AM-12:30 PM
Starr D’Auria is an NDE Engineer at Extende Inc, where she specializes in CIVA simulation software and TRAINDE NDT training simulators. She offers sales, technical support, training, and consulting services for these products. She holds a Bachelor of Science in Mechanical Engineering from LeTourneau University and has Level II VT and UT training, as well as ET, RT, PT and MT training. She leads the Hampton Roads ASNT chapter as the chairperson and serves on the steering committee for the DWGNDT.
Abstract: CIVA NDT Simulation: Improving Inspections Today for a Better Tomorrow
Improving the Quality of Test & Evaluation
CIVA NDT simulation software is a powerful tool for non-destructive testing (NDT) applications. It allows users to design, optimize, and validate inspection procedures for various NDT methods, such as ultrasonic, eddy current, radiographic, and guided wave testing. Come learn about the benefits of using CIVA NDT simulation software to improve the reliability, efficiency, and cost-effectiveness of NDT inspections.
Session Materials: https://dataworks.testscience.org/wp-content/uploads/formidable/23/Wed_1C_DAuria.pptx
Steven Movit
Research Staff Member, IDA
Operationally Representative Data and Cybersecurity for Avionics
Day 2, Room: B 10:30 AM-12:30 PM
Dr. Steven Movit earned bachelor's degrees in Astrophysics and Statistics from Rice University in Houston, Texas, followed by a doctorate from Penn State University in Astronomy and Astrophysics, where he worked on the IceCube telescope.
Dr. Movit joined the Institute for Defense Analyses in 2011, where he has concentrated on aircraft survivability, including cyber survivability and electronic warfare, mainly supporting analyses for the Director, Operational Test and Evaluation. Dr. Movit started a "non-IP" cyber lab at IDA in 2021 which has developed hardware-in-the-loop simulators for research and training.
Abstract: Operationally Representative Data and Cybersecurity for Avionics
Advancing Test & Evaluation of Emerging and Prevalent Technologies
This talk discusses the ARINC 429 standard and its inherent lack of security, demonstrates proven mission effects in a hardware-in-the-loop (HITL) simulator, and presents a data set collected from real avionics.
ARINC 429 is a ubiquitous data bus for civil avionics, enabling safe and reliable communication between devices from disparate manufacturers. However, ARINC 429 lacks any form of encryption or authentication, making it an inherently insecure communication protocol and rendering any connected avionics vulnerable to a range of attacks.
We constructed a HITL simulator with ARINC 429 buses to explore these vulnerabilities, and to identify potential mission effects. The HITL simulator includes commercial off-the-shelf avionics hardware including a multi-function display, an Enhanced Ground Proximity Warning System, as well as a realistic flight simulator.
We performed a denial-of-service attack against the multi-function display via a compromised transmit node on an ARINC 429 bus, using commercially available tools, which succeeded in disabling important navigational aids. This simple replay attack demonstrates how effectively a “leave-behind” device can cause serious mission effects.
This proven adversarial effect on physical avionics illustrates the risk inherent in ARINC 429 and the need for the ability to detect, mitigate, and recover from these attacks. One potential solution is an intrusion detection system (IDS) trained using data collected from the electrical properties of the physical bus. Although previous research has demonstrated the feasibility of an IDS on an ARINC 429 bus, none have been trained on data generated by actual avionics hardware.
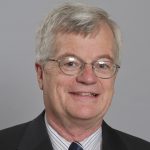
Terril Hurst
Senior Engineering Fellow, Raytheon
A Bayesian Approach for Credible Modeling, Simulation, and Analysis
Day 3, Room: C 1:00 PM-3:00 PM
Terril Hurst has worked at Raytheon since 2005, with focus on rigorous methods for developing and using modeling and simulation resources. He teaches several courses, including a Johns Hopkins/Raytheon course in credible modeling and simulation, and internal courses for DASE, Bayesian Networks, and Bayesian Analysis. Terril has attended and contributed regularly for fifteen years to DATAWorks, CASD, and ACAS.
Prior to joining Raytheon, Dr. Hurst worked for 27 years at Hewlett-Packard Laboratories on developing and testing computer data storage devices and distributed systems. He obtained all of his degrees at Brigham Young University and completed a post-doctoral appointment in artificial intelligence at Stanford University.
Terril and his wife Mary have six children and seventeen grandchildren, who he includes in his amateur astronomy and model rocket hobbies.
Abstract: A Bayesian Approach for Credible Modeling, Simulation, and Analysis
During the 2016 Conference on Applied Statistics in Defense (CASD), we presented a paper describing “The DASE Axioms.” The paper included several “divide-and-conquer” strategies for addressing the Curse of Dimensionality that is typical in simulated systems.
Since then, a new, integrate-and-conquer approach has emerged, which applies decision-theoretic concepts from Bayesian Analysis (BA). This paper and presentation re-visit the DASE axioms from the perspective of BA.
Over the past fifteen years, we have tailored and expanded conventional design-of-experiments (DOE) principles to take advantage of the flexibility that is offered by modeling, simulation, and analysis (MSA). The result is embodied within three, high-level checklists: (a) the Model Description and Report (MDR) protocol enables iteratively developing credible models and simulation (M&S) for an evolving intended use; (b) the 7-step Design & Analysis of Simulation Experiments (DASE) protocol guides credible M&S usage; and (c) the Bayesian Analysis (BA ) protocol enables fully quantifying the uncertainty that accumulates, both when building and using M&S.
When followed iteratively by all MSA stakeholders throughout the product lifecycle, the MSA protocols result in effective and efficient risk-informed decision making.
The paper and presentation include several quantitative examples to show how the three MSA protocols interact. For example, we show how to use BA to combine Sim and field data for calibrating M&S. Thereafter, given a well-specified query, adaptive sampling is illustrated for optimizing usage of high-performance computing (HPC), either to minimize resources required to answer a specific query, or to maximize HPC utilization within a fixed time period.
The Bayesian approach to M&S development and usage reflects a shift in perspective, from viewing MSA as mainly a design tool, to being a digital test and evaluation venue. This change renders fully relevant all of the attendant operational constraints and associated risks regarding M&S scheduling, availability, cost, accuracy, and delay in analyzing inappropriately large HPC data sets. The MSA protocols employ statistical models and other aspects of Scientific Test and Analysis Techniques (STAT) that are being taught and practiced within the operational test and evaluation community.
Session Materials: https://dataworks.testscience.org/wp-content/uploads/formidable/23/Thrs_CS3_Hurst.pptx
Thomas Ulrich
Human Factors and Reliability Research Scientist, Idaho National Laboratory
Rancor-HUNTER: A Virtual Plant and Operator Environment for Predicting Human Performance
Day 2, Room: B 1:40 PM-3:10 PM
Abstract: Rancor-HUNTER: A Virtual Plant and Operator Environment for Predicting Human Performance
Advancing Test & Evaluation of Emerging and Prevalent Technologies
Advances in simulation capabilities to model physical systems have outpaced the development of simulations for humans using those physical systems. There is an argument that the infinite span of potential human behaviors inherently render human modeling more challenging than physical systems. Despite this challenge, the need for modeling humans interacting with these complex systems is paramount. As technologies have improved, many of the failure modes originating from the physical systems have been solved. This means the overall proportion of human errors has increased, such that it is not uncommon to be the primary driver of system failure in modern complex systems. Moreover, technologies such as automated systems may introduce emerging contexts that can cause new, unanticipated modes of human error. Therefore, it is now more important than ever to develop models of human behavior to realize overall system error reductions and achieve established safety margins. To support new and novel concepts of operations for the anticipated wave of advanced nuclear reactor deployments, human factors and human reliability analysis researchers need to develop advanced simulation-based approaches. This talk presents a simulation environment suitable to both collect data and then perform Monte Carlo simulations to evaluate human performance and develop better models of human behavior. Specifically, the Rancor Microworld Simulator models a complex energy production system in a simplified manner. Rancor includes computer-based procedures, which serve as a framework to automatically classify human behaviors without manual, subjective experimenter coding during scenarios. This method supports a detailed level of analysis at the task level. It is feasible for collecting large sample sizes required to develop quantitative modelling elements that have historically challenged traditional full-scope simulator study approaches. Additionally, the other portion of this experimental platform, the Human Unimodel for Nuclear Technology to Enhance Reliability (HUNTER), is presented to show how the collected data can be used to evaluate novel scenarios based on the contextual factors, or performance shaping factors, derived from Rancor simulations. Rancor-HUNTER is being used to predict operator performance with new procedures, such as results from control room modernization or new-build situations. Rancor-HUNTER is also proving a useful surrogate platform to model human performance for other complex systems.
Session Materials: https://dataworks.testscience.org/wp-content/uploads/formidable/23/Wed_2B_Ulrich.pptxTodd Remund
Staff Data Scientist, Northrop Grumman
Rocket Motor Design Qualification Through Enhanced Reliability Assurance Testing
Day 3, Room: C 1:00 PM-3:00 PM
Todd received his BS and MS degrees in statistics from Brigham Young University in Provo Utah. After graduation he worked for ATK where he worked on the Shuttle program, Minute Man, Peace Keeper, and other DOD and NASA related programs. He left ATK to go to Edwards AFB to work as a civil servant. While there he had the opportunity to do statistics on nearly every fighter, bomber, cargo plane, refueler, and UAV that you can think of. After six years at Edwards AFB he returned to Utah to work at Orbital ATK, now Northrop Grumman Space Systems where he currently works as a staff data scientist / statistician and LMDS technical fellow.
Abstract: Rocket Motor Design Qualification Through Enhanced Reliability Assurance Testing
Improving the Quality of Test & Evaluation
Composite pressure vessel designs for rocket motors must be qualified for use in both military and space applications. By intent, demonstration testing methods ignore a priori information about a system which inflates typically constrained test budgets and often have low probability of test success. On the other hand, reliability assurance tests encourage use of previous test data and other relevant information about a system. Thus, an assurance testing approach can dramatically reduce the cost of a qualification test. This work extends reliability assurance testing to allow scenarios with right-censored and exact failure possibilities. This enhancement increases the probability of test success and provides a post-test re-evaluation of test results. The method is demonstrated by developing a rocket motor design qualification assurance test.
Session Materials: https://dataworks.testscience.org/wp-content/uploads/formidable/23/Thrs_CS3_Remund.pptx
Dr. Tom Donnelly
JMP Statistical Discovery LLC
Design and Analysis of Experiments – Next-Level Methods with Case Studies
Day 1, Room: A 9:00 AM-4:00 PM
Tom Donnelly works as a Systems Engineer for JMP Statistical Discovery supporting users of JMP software in the Defense and Aerospace sector. He has been actively using and teaching Design of Experiments (DOE) methods for the past 40 years to develop and optimize products, processes, and technologies. Donnelly joined JMP in 2008 after working as an analyst for the Modeling, Simulation & Analysis Branch of the US Army’s Edgewood Chemical Biological Center – now DEVCOM CBC. There, he used DOE to develop, test, and evaluate technologies for detection, protection, and decontamination of chemical and biological agents. Prior to working for the Army, Tom was a partner in the first DOE software company for 20 years where he taught over 300 industrial short courses to engineers and scientists. Tom received his PhD in Physics from the University of Delaware.
Abstract: Design and Analysis of Experiments – Next-Level Methods with Case Studies
Advancing Test & Evaluation of Emerging and Prevalent Technologies
This is the short course for you if you are familiar with the fundamental techniques in the science of test and want to learn useful, real-world, and advanced methods applicable in the DoD/NASA test community. The focus will be on use cases not typically covered in most short courses. JMP software will primarily be used, and datasets will be provided for you to follow along many of the hands-on demonstrations of practical case studies. Design topics will include custom design of experiments tips, choosing optimality criteria, creating designs from existing runs, augmenting adaptively in high gradient regions, creating designs with constraints, repairing broken designs, mixture design intricacies, modern screening designs, designs for computer simulation, accelerated life test, and measurement system testing. Analysis topics will include ordinary least squares, stepwise, and logistic regression, generalized regression (LASSO, ridge, elastic net), model averaging (to include Self-Validated Ensemble Models), random effects (split-plot, repeated measures), comparability/equivalence, functional data analysis (think your data is a curve), nonlinear approaches and multiple response optimization and trade-space analysis. The day will finish with an hour-long Q&A session to help solve your specific T&E challenges.
Session Materials: https://dataworks.testscience.org/wp-content/uploads/formidable/23/DATAWorks-2024-Next-Level-DOE-Short-Course-Materials-1.zip
Tyler Morgan-Wall
Research Staff Member, IDA
Simulation Insights on Power Analysis with Binary Responses: From SNR Methods to 'skprJMP'
Day 2, Room: A 3:30 PM-5:00 PM
Dr. Tyler Morgan-Wall is a Research Staff Member at the Institute for Defense Analyses, and is the developer of the software library skpr: a package developed at IDA for optimal design generation and power evaluation in R. He is also the author of several other R packages for data visualization, mapping, and cartography. He has a PhD in Physics from Johns Hopkins University and lives in Silver Spring, MD.
Abstract: Simulation Insights on Power Analysis with Binary Responses: From SNR Methods to 'skprJMP'
Sharing Analysis Tools, Methods, and Collaboration Strategies
Logistic regression is a commonly-used method for analyzing tests with probabilistic responses in the test community, yet calculating power for these tests has historically been challenging. This difficulty prompted the development of methods based on signal-to-noise ratio (SNR) approximations over the last decade, tailored to address the intricacies of logistic regression's binary outcomes and complex probability distributions. Originally conceived as a solution to the limitations of then-available statistical software, these approximations provided a necessary, albeit imperfect, means of power analysis. However, advancements and improvements in statistical software and computational power have reduced the need for such approximate methods. Our research presents a detailed simulation study that compares SNR-based power estimates with those derived from exact Monte Carlo simulations, highlighting the inadequacies of SNR approximations. To address these shortcomings, we will discuss improvements in the open-source R package "skpr" as well as present "skprJMP," a new plug-in that offers more accurate and reliable power calculations for logistic regression analyses for organizations that prefer to work in JMP. Our presentation will outline the challenges initially encountered in calculating power for logistic regression, discuss the findings from our simulation study, and demonstrate the capabilities and benefits "skpr" and "skprJMP" provide to an analyst.
Session Materials: https://dataworks.testscience.org/wp-content/uploads/formidable/23/Wed_3A_Morgan-Wall.pptxTyler Morgan-Wall
RSM, IDA
Live demo: Monte Carlo power evaluation with “skpr” and “skprJMP”
Day 2, Room: Cafe 5:00 PM-7:00 PM
Abstract: Live demo: Monte Carlo power evaluation with “skpr” and “skprJMP”
Sharing Analysis Tools, Methods, and Collaboration Strategies
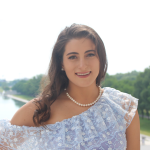
Victoria Nilsen
Operations Research Analyst, NASA HQ
Examining the Effects of Implementing Data-Driven Uncertainty in Cost Estimating Models
Day 3, Room: C 9:50 AM-11:50 AM
Vicky Nilsen is an Operations Research Analyst within the Office of the Chief Financial Officer (OCFO) at NASA Headquarters in Washington, DC. In this role, Vicky serves as OCFO's point of contact for cost and schedule analysis, research, and model development. Vicky began her tenure as a civil servant at NASA in March 2022. Prior to this, she has been affiliated with NASA in various ways. In 2017, she was a systems engineer on the George Washington University CubeSat project, sponsored by NASA's CubeSat Launch Initiative. From 2018-2020, she performed academic research for the Mission Design Lab at NASA's Goddard Space Flight Center and Team X at the Jet Propulsion Lab. In 2019, she was an intern in OCFO's Portfolio Investment Analysis branch working on cost and schedule analysis for the Human Landing System of the Artemis mission. Most recently, she worked as a contractor supporting the development of NASA's 2022 Strategic Plan and Evidence Act. She is extremely passionate about the work that she has done and continues to do at NASA and aims to drive change and innovation within NASA's Project Planning & Control and Cost Estimating communities.
Abstract: Examining the Effects of Implementing Data-Driven Uncertainty in Cost Estimating Models
Solving Program Evaluation Challenges
When conducting probabilistic cost analysis, correlation assumptions are key assumptions and often a driver for the total output or point estimate of a cost model. Although the National Aeronautics and Space Administration (NASA) has an entire community dedicated to the development of statistical cost estimating tools and techniques to manage program and project performance, the application of accurate and data-driven correlation coefficients within these models is often overlooked. Due to the uncertain nature of correlation between random variables, NASA has had difficulty quantifying the relationships between spacecraft subsystems with specific, data-driven correlation matrices. Previously, the NASA cost analysis community has addressed this challenge by either selecting a blanket correlation value to address uncertainty within the model or opting out of using any correlation value altogether. One hypothesized method of improving NASA cost estimates involves deriving subsystem correlation coefficients from the residuals of the regression equations for the cost estimating relationships (CERs) of various spacecraft subsystems and support functions. This study investigates the feasibility of this methodology using the CERs from NASA's Project Cost Estimating Capability (PCEC) model. The correlation coefficients for each subsystem of the NASA Work Breakdown Structure were determined by correlating the residuals of PCEC's subsystem CERs. These correlation coefficients were then compiled into a 20x20 correlation matrix and were implemented into PCEC as an uncertainty factor influencing the model's pre-existing cost distributions. Once this correlation matrix was implemented into the cost distributions of PCEC, the Latin Hypercube Sampling function of the Microsoft Excel add-in Argo was used to simulate PCEC results for 40 missions within the PCEC database. These steps were repeated three additional times using the following correlation matrices: (1) a correlation matrix assuming the correlation between each subsystem is zero, (2) a correlation matrix assuming the correlation between each subsystem is 1, and (3) a correlation matrix using a blanket value of 0.3. The results of these simulations showed that the correlation matrix derived from the residuals of the subsystem CERs significantly reduced bias and error within PCEC's estimating capability. The results also indicated that the probability density function and cumulative distribution function of each mission in the PCEC database were altered significantly by the correlation matrices that were implemented into the model. This research produced (1) a standard subsystem correlation matrix that has been proven to improve estimating accuracy within PCEC and (2) a replicable methodology for creating this correlation matrix that can be used in future cost estimating models. This information can help the NASA cost analysis community understand the effects of applying uncertainty within cost models and perform sensitivity analyses on project cost estimates. This is significant because NASA has been frequently critiqued for underestimating project costs and this methodology has shown promise in improving NASA's future cost estimates and painting a more realistic picture of the total possible range of spacecraft development costs.
Session Materials: https://dataworks.testscience.org/wp-content/uploads/formidable/23/Thrs_4C_Nilsen-1.pptx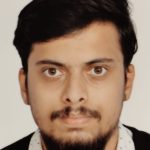
Vishal Subedi
PhD Student, University of Maryland Baltimore County
Classifying violent anti-government conflicts in Mexico: A machine learning framework
Day 2, Room: B 3:30 PM-5:00 PM
I am a first year PhD student at UMBC. My interests lie in applied statistics, machine learning and deep learning.
Abstract: Classifying violent anti-government conflicts in Mexico: A machine learning framework
Domestic crime, conflict, and instability pose a significant threat to many contemporary governments.
These challenges have proven to be particularly acute within modern-day Mexico. While there have been significant developments in predicting intrastate armed and electoral conflict in various contemporary settings, such efforts have thus far been limited in their use of spatial as well as temporal correlations, as well as in the features they have considered. Machine learning, especially deep learning, has been proven to be highly effective in predicting future conflicts
using word embeddings in Convolutional Neural Networks (CNN) but lacks the spatial structure and, due to the black box nature, cannot explain the importance of predictors. We develop a novel methodology using machine learning that can accurately classify future anti-government violence in Mexico. We further demonstrate that our approach can identify important leading predictors of such violence. This can help policymakers make informed decisions and can also help governments and NGOs better allocate security and humanitarian resources, which could prove beneficial in tackling this problem. Using a variety of political event aggregations from the ICEWS database alongside other textual and demographic features, we trained various classical machine learning algorithms, including but not limited
to Logistic Regression, Random Forest, XGBoost, and a Voting classifier. The development of this reseearch was a stepwise process in three phases where the following phase was built upon the shortcomings of the previous phases. In the very first phase, we considered a mix of CNN + Long Short Term Memory (LSTM) networks to decode the spatial and temporal relationship in the data. The performance of all the black box deep learning models was not at par with the classical machine learning models. The second phase deals with the analysis of the temporal relationships in the data to identify the dependency of the conflicts over time and its lagged relationship. This also serves as a method to reduce feature dimension space by removing variables not covered with the cutoff lag. The third phase talks about the general variable selection methodologies used to further reduce the feature space along with identifying the important predictors that fuel anti-government violence along with their directional effect using Shapley additive values. The voting classifier, utilizing a subset of features derived from LASSO
across 100 simulations, consistently surpasses alternative models in performance and demonstrates efficacy in accurately classifying future anti-government conflicts. Notably, Random Forest feature importance indicates that some features, including but not limited to homicides, accidents, material conflicts, and positive worded citizen information sentiments emerge as pivotal predictors in the classification of anti-government conflicts. Finally, in the fourth phase, we conclude the
research by analysing the spatial structure of the data using Moran’s I index extended version for spatiotemporal data to identify the global spatial dependency and local clusters followed by modelling the data spatially and evaluating the same using Gaussian Process Boost(GPBoost). The global spatial autocorrelation is minimal, characterized by localized conflicts cluster within the region. Furthermore, the Voting Classifier demonstrates superior performance over GPBoost, leading to the inference that no substantial spatial dependency exists among the various locations.

Yosef Razin
Research Associate, IDA
Developing AI Trust: From Theory to Testing and the Myths In Between
Day 2, Room: B 1:40 PM-3:10 PM
Yosef S. Razin is a Research Associate at IDA and doctoral candidate in Robotics at the Georgia Institute of Technology, specializing in human-machine trust and the particular challenges to trust that AI poses. His research has spanned the psychology of trust, ethical and legal implications, game theory, and trust measure development and validation. His applied research has focused on human-machine teaming, telerobotics, autonomous cars, and AI-assistants and decision support. At IDA, he is in the Operational Evaluation Division and involved with the Human-System Integration group and the Test Science group.
Abstract: Developing AI Trust: From Theory to Testing and the Myths In Between
The Director, Operational Test and Evaluation (DOT&E) and the Institute for Defense Analyses (IDA) are developing recommendations for how to account for trust and trustworthiness in AI-enabled systems during Department of Defense (DoD) Operational Testing (OT). Trust and trustworthiness have critical roles in system adoption, system use and misuse, and performance of human-machine teams. The goal, however, is not to maximize trust, but to calibrate the human’s trust to the system’s trustworthiness. Trusting more than a system warrants can result in shattered expectations, disillusionment, and remorse. Conversely, under trusting implies that humans are not making the most of available resources.
Trusted and trustworthy systems are commonly referenced as essential for the deployment of AI by political and defense leaders and thinkers. Executive Order 14110 requires “safe, secure, and trustworthy development and use” of AI. Furthermore, the desired end state of the Department of Defense Responsible AI Strategy is trust. These terms are not well characterized and there is no standard, accepted model for understanding, or method for quantifying, trust or trustworthiness for test and evaluation (T&E). This has resulted in trust and trust calibration rarely being assessed in T&E. This is, in part, due to the contextual and relational nature of trustworthiness. For instance, the developmental tester requires a different level of algorithmic transparency than the operational tester or the operator; whereas the operator may need more understandability than transparency. This means that to successfully operationally test AI-enabled systems, such testing must be done at the right level, with the actual operators and commanders and up-to-date CONOPS as well as sufficient time for training and experience for trust to evolve. The need for testing over time is further amplified by particular features of AI, wherein machine behaviors are no longer as predictable or static as traditional systems but may continue to be updated and adaptive. Thus, testing for trust and trustworthiness cannot be one and done.
It is critical to ensure that those who work within AI – in its design, development, and testing – understand exactly what trust actually means, why it is important, and how to operationalize and measure it. This session will empower testers by:
• Establishing a common foundation for understanding what trust and trustworthiness are.
• Defining key terms related to trust, enabling testers to think about trust more effectively.
• Demonstrating the importance of trust calibration for system acceptance and use and the risks of poor calibration.
• Decomposing the factors within trust to better elucidate how trust functions and what factors and antecedents have been shown to effect trust in human-machine interaction.
• Introducing concepts on how to design AI-enabled systems for better trust calibration, assurance, and safety.
• Proposing validated and reliable survey measures for trust.
• Discussing common cognitive biases implicated in trust and AI and both the positive and negative roles biases play.
• Addressing common myths around trust in AI, including that trust or its measurement doesn’t matter, or that trust in AI can be “solved” with ever more transparency, understandability, and fairness.
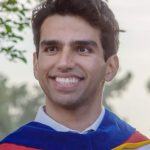
Zaki Hasnain
Data Scientist, NASA Jet Propulsion Laboratory
Onboard spacecraft thermal modeling using physics informed machine learning
Day 2, Room: C 10:30 AM-12:30 PM
Dr. Zaki Hasnain is a data scientist in NASA JPL’s Systems Engineering Division where he participates in and leads research and development tasks for space exploration. His research interests include physics informed machine learning and system health management for autonomous systems. He has experience developing data-driven, game-theoretic, probabilistic, physics-based, and machine learning models and algorithms for space, cancer, and autonomous systems applications. He received a B.S. in engineering science and mechanics at Virginia Polytechnic and State University. He received M.S. and Ph.D. degrees in mechanical engineering, and a M.S. in computer science at University of Southern California.
Abstract: Onboard spacecraft thermal modeling using physics informed machine learning
Modeling thermal states for complex space missions, such as the surface exploration of airless bodies, requires high computation, whether used in ground-based analysis for spacecraft design or during onboard reasoning for autonomous operations. For example, a finite-element-method (FEM) thermal model with hundreds of elements can take significant time to simulate on a typical workstation, which makes it unsuitable for onboard reasoning during time-sensitive scenarios such as descent and landing, proximity operations, or in-space assembly. Further, the lack of fast and accurate thermal modeling drives thermal designs to be more conservative and leads to spacecraft with larger mass and higher power budgets.
The emerging paradigm of physics-informed machine learning (PIML) presents a class of hybrid modeling architectures that address this challenge by combining simplified physics models (e.g., analytical, reduced-order, and coarse mesh models) with sample-based machine learning (ML) models (e.g., deep neural networks and Gaussian processes) resulting in models which maintain both interpretability and robustness. Such techniques enable designs with reduced mass and power through onboard thermal-state estimation and control and may lead to improved onboard handling of off-nominal states, including unplanned down-time (e.g. GOES-7 cite{bedingfield1996spacecraft}, and M2020)
The PIML model or hybrid model presented here consists of a neural network which predicts reduced nodalizations (coarse mesh size) given on-orbit thermal load conditions, and subsequently a (relatively coarse) finite-difference model operates on this mesh to predict thermal states. We compare the computational performance and accuracy of the hybrid model to a purely data-driven model, and a high-fidelity finite-difference model (on a fine mesh) of a prototype Earth-orbiting small spacecraft. This hybrid thermal model promises to achieve 1) faster design iterations, 2) reduction in mission costs by circumventing worst-case-based conservative planning, and 3) safer thermal-aware navigation and exploration.